AI Insurance Policy Analysis and Coverage Checker - Get Instant Insights from Your Policy Documents (Get started for free)
Underwriting vs
Actuarial Science Key Differences in Insurance Risk Assessment
Underwriting vs
Actuarial Science Key Differences in Insurance Risk Assessment - Underwriting Focus on Individual Risk Assessment
Underwriters are the front line in insurance risk assessment, taking a deep dive into the specifics of each individual applicant. They meticulously scrutinize personal details, like medical records and financial histories, to pinpoint the unique risks associated with each potential policyholder. Their task isn't simply to categorize people into broad risk groups like actuaries do; it's about understanding the individual case, making informed decisions about policy terms and pricing. This direct engagement with individual risk profiles is crucial for insurers, as they rely on underwriters to make sound decisions that ensure the financial health of the company while complying with complex legal regulations.
The evolution of predictive analytics has significantly impacted this process, equipping underwriters with new tools to dissect data and provide even more nuanced risk assessments. This evolution signifies a shift towards more precise and individualized risk evaluation in underwriting.
Underwriting, at its core, focuses on meticulously evaluating each individual seeking insurance. It's not just about crunching numbers, but delving deep into the specifics of each application. This involves scrutinizing medical records, financial documents, and a wealth of other personal details to assess the likelihood of a claim and, in turn, craft policy terms that accurately reflect the calculated risk.
Think of it like a puzzle. Actuaries, the data wizards of the insurance world, work behind the scenes to establish the overall risk profiles, which act as the framework for underwriting. But underwriters are the ones piecing together the individual pieces – the unique factors of each applicant – to see how they fit into that bigger picture.
This meticulous, person-by-person analysis is increasingly powered by technology. Algorithms are now capable of sifting through mountains of data in real-time, drawing on a vast range of variables including socioeconomic status, driving records, and even credit scores to paint a detailed portrait of risk. This not only allows for more accurate assessments but also drastically shortens the traditional underwriting timelines.
However, the picture is far from complete without considering the human element. While algorithms are incredibly powerful tools, they can't always grasp the nuances of human behavior. Underwriters, through personalized interviews, can glean insights that data alone can't reveal, like an applicant's risk perception and overall outlook on life. This deeper understanding contributes to a more comprehensive and well-rounded risk assessment.
Yet, with the rise of the gig economy, the traditional definition of "risk" is being challenged. The ever-changing employment landscape poses a new challenge for underwriters, as freelancers and contractors don't neatly fit into the conventional frameworks of risk assessment. This demands a continuous adaptation of models to reflect the dynamic nature of work and the evolving factors that contribute to risk.
The landscape of underwriting is constantly evolving, propelled by the intersection of technology, data, and human insight. As we move forward, we can expect a continued emphasis on individual risk assessment, fueled by increasingly sophisticated algorithms, but grounded in the understanding that every individual presents a unique story, one that deserves a personalized and thorough evaluation.
Underwriting vs
Actuarial Science Key Differences in Insurance Risk Assessment - Actuarial Science Emphasis on Statistical Modeling
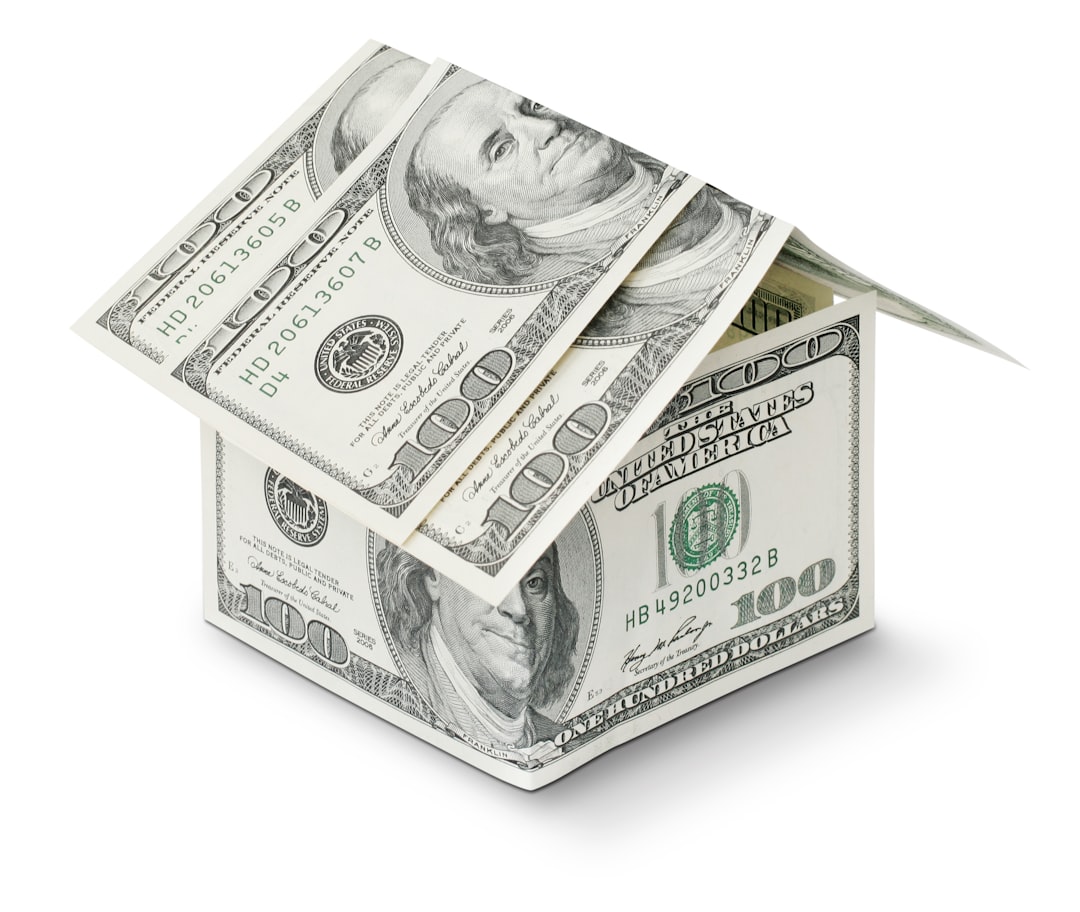
Actuarial science is a key part of the insurance world. It uses sophisticated statistical modeling to assess and predict risks. This discipline focuses on developing predictive frameworks that analyze massive amounts of data. This analysis is essential for creating sound risk management strategies. Unlike underwriters who focus on individual assessments, actuaries look at risk from a broader statistical perspective. They analyze financial implications and try to predict future risks. The constant evolution of statistical modeling in actuarial science reflects the need for the insurance industry to adapt to new challenges and trends in risk assessment. Understanding the intricate connection between actuarial science and underwriting is vital to fully understanding how the insurance world functions.
Actuarial science is a fascinating field that heavily relies on statistical modeling. These models go far beyond simple linear relationships and delve into complex patterns within massive amounts of data. Imagine analyzing not just past claims but also incorporating decades of historical data trends to understand how risks change over time. This gives actuaries a powerful tool for predicting future events.
They use sophisticated methods like survival analysis, where they essentially study the "time until an event," like an insurance claim. Think of it as predicting how long a customer might be a policyholder before a claim is made.
Another intriguing aspect is how actuarial science embraces non-linearity, moving beyond traditional linear models. They employ techniques like decision trees and machine learning to identify intricate patterns that might otherwise be missed.
This isn't just about crunching numbers, though. Actuarial science weaves together insights from fields like economics, finance, mathematics, and even psychology. They use stochastic modeling to factor in uncertainty and create a range of potential outcomes rather than just a single prediction. This gives insurers a more comprehensive view of risks and allows for more strategic management.
I'm particularly interested in the incorporation of big data. Actuaries are now exploring how unstructured data, like social media activity or wearable health metrics, can improve their risk assessments. It's a new frontier in understanding human behavior, and it's shaping how we think about risk.
The actuary's work is subject to strict regulations, ensuring that the models are not only accurate but also comply with legal requirements. It's an intricate interplay between statistical expertise and legal frameworks.
The field is continually evolving, with actuaries incorporating real-time data analysis into their models. This enables them to quickly adjust to changing trends and behaviors. It's fascinating to see how the field is embracing technology to stay ahead of the curve in risk management.
Underwriting vs
Actuarial Science Key Differences in Insurance Risk Assessment - Differences in Risk Evaluation Methods
Underwriting and actuarial science, both integral to insurance risk assessment, utilize distinct methods for evaluating risk. Underwriters focus on the individual, meticulously examining personal details like medical history and financial records to determine unique risks. This individualized approach results in custom-tailored policy terms and premiums for each applicant. In contrast, actuaries analyze large data sets to identify trends and statistically model risk for broader populations. Their work involves developing predictive frameworks that forecast future outcomes, providing insurers with the tools to manage risk effectively.
These contrasting methodologies are fueled by the evolving world of technology and data analytics. Underwriters leverage predictive analytics to provide more nuanced individual assessments, while actuaries refine their models to incorporate real-time data and adapt to emerging trends. This continuous evolution ensures that both disciplines remain at the forefront of risk management. Understanding the distinct approaches of underwriting and actuarial science is crucial for comprehending the intricate dynamics of the insurance industry.
While underwriters delve deep into the specifics of individual applicants, focusing on data like medical records and financial histories, actuaries take a broader statistical approach. They employ powerful statistical models and look to historical data, sometimes spanning decades, to identify overarching risk trends. This allows actuaries to predict future risks and financial implications.
The way these two fields utilize data is quite different. Underwriters are more concerned with immediate risks presented by each applicant, while actuaries use data to make long-term projections, sometimes spanning years.
This leads to a divergence in how these professionals use predictive models. Actuaries use sophisticated techniques like survival analysis and machine learning, allowing them to identify intricate relationships within data that may not be obvious during individual assessments.
One of the biggest differences lies in how they adapt to change. Underwriters typically rely on static data provided at the time of application. Actuaries, however, are increasingly using real-time data analytics to adjust their risk models based on evolving trends. This allows them to stay ahead of the curve in a rapidly changing world.
While actuaries excel at modeling complex risk scenarios, they may overlook the nuances of human behavior. Underwriters, through personal interactions, can often gain a more intimate understanding of how an individual perceives risk.
Actuaries also work within a heavily regulated environment, their models often needing to comply with stringent legal requirements. This places a heavier emphasis on legal frameworks for actuaries, whereas underwriters primarily focus on individual risk and deviations from standardized policies.
Technology is playing a huge role in both fields. While AI is transforming underwriting by enhancing individual evaluations, actuaries are exploring the use of unstructured data, like social media and wearable health metrics, to further refine their risk assessments. This broader approach to data exploration opens up a new frontier in understanding human behavior and its implications for risk.
Finally, the very nature of risk itself presents challenges. Insurance risk can often exhibit fractal characteristics, meaning patterns repeat at different scales. Actuaries are adept at recognizing these complex patterns through sophisticated modeling techniques. These techniques are often not employed by underwriters.
The evolving world of work presents another challenge. The rise of gig workers and other unconventional work arrangements makes it difficult to categorize risks using traditional methods. Actuaries are adapting their models to account for this changing landscape, while underwriters may find it difficult to classify these non-standard applicants effectively.
Both underwriting and actuarial science are vital components of the insurance world. They represent two different approaches to risk evaluation, each with its strengths and weaknesses. As technology and the nature of work continue to evolve, understanding the dynamic interplay between these fields will be crucial to navigating the future of insurance.
Underwriting vs
Actuarial Science Key Differences in Insurance Risk Assessment - Contrasting Approaches to Premium Pricing
"Contrasting Approaches to Premium Pricing" delves into the contrasting ways insurance companies assess risk and determine premiums. Underwriters focus on the individual, carefully evaluating their specific circumstances to tailor policies and prices. Actuarial science, on the other hand, relies on broad statistical analysis to identify risk trends across entire populations, leading to standardized pricing. This difference in perspective impacts how premiums are calculated, with underwriters potentially adjusting for unique circumstances and actuaries providing a more general pricing approach. As the industry evolves, the need to bridge these two perspectives becomes critical, allowing for more nuanced risk assessment and effective pricing strategies in a rapidly changing world.
The pricing of insurance premiums is a complex process influenced by various factors and subject to continuous evolution. This field intersects with disciplines like behavioral economics, data science, and even marketing, highlighting the multifaceted nature of premium pricing.
While actuaries rely on statistical models and vast datasets to predict risk and determine average pricing, a more nuanced approach emerges from the realm of individual pricing, which is the focus of underwriters. This approach, driven by behavioral economics and data analytics, focuses on individual characteristics and preferences. This creates a dynamic interplay between broader statistical trends and the unique characteristics of each policyholder.
A key element of premium pricing lies in understanding the varying levels of price sensitivity across different insurance products. For instance, consumers of auto and health insurance tend to be more price-sensitive, whereas others might be less influenced by premium fluctuations. This creates opportunities to tailor premium strategies to the specific product and consumer segments.
Furthermore, premium pricing often exhibits a curious phenomenon known as the Veblen effect, where higher prices can paradoxically lead to increased demand. Consumers may perceive more expensive insurance products as more desirable, thus deviating from traditional economic logic. This demonstrates the need to consider psychological factors in pricing strategies.
Another crucial aspect is the use of segmentation. Insurers segment customers into groups based on their perceived willingness to pay, enabling them to optimize revenue. However, this segmentation can potentially overlook niche markets with unexpected demand, raising questions about the effectiveness of these strategies in the long run.
Behavioral economics offers valuable insights into customer decision-making. Understanding concepts like framing effects and loss aversion helps insurers craft premium structures that resonate with consumers and predict their purchasing behavior with greater accuracy. These insights are becoming increasingly important as consumers become more sophisticated and demanding in their choices.
Furthermore, the choice of distribution channels also impacts premium pricing. Direct-to-consumer channels can offer lower premiums compared to traditional agents, impacting competitive dynamics and challenging existing market structures.
Adding complexity is the influence of regulations, which can vary significantly across jurisdictions. Some regions impose strict guidelines on premium calculation and adjustment, necessitating a careful balance between competitive pricing and legal compliance.
The use of consumer data for personalized premium pricing is a controversial subject, raising important concerns about data privacy. The potential for data misuse and the lack of transparency around how data influences premiums can lead to ethical dilemmas, emphasizing the need for clear regulations and safeguards.
The integration of advanced technologies like artificial intelligence and machine learning has significantly impacted premium pricing methodologies. These tools enable insurers to analyze vast datasets quickly, facilitating real-time premium adjustments based on emerging risk factors.
However, the focus on acquiring new customers should not overshadow the importance of retaining existing policyholders. Implementing strategies to minimize churn is crucial in a competitive landscape. Tailored premiums and loyalty incentives are increasingly used to retain high-value customers, recognizing the importance of building lasting relationships.
Finally, consumers often experience cognitive dissonance when faced with premium pricing decisions, especially when they perceive a mismatch between premium costs and expected benefits. This can lead to second-guessing and switching behavior, requiring insurers to address this dissonance proactively through transparent communication and effective risk communication strategies.
In conclusion, premium pricing is a dynamic field shaped by a complex interplay of statistical modeling, behavioral economics, technological advancements, and regulatory influences. The evolution of premium pricing strategies necessitates a continuous effort to balance financial objectives with consumer preferences and ethical considerations, ensuring a sustainable and responsible approach to this crucial aspect of the insurance industry.
Underwriting vs
Actuarial Science Key Differences in Insurance Risk Assessment - Roles in Application Review Process
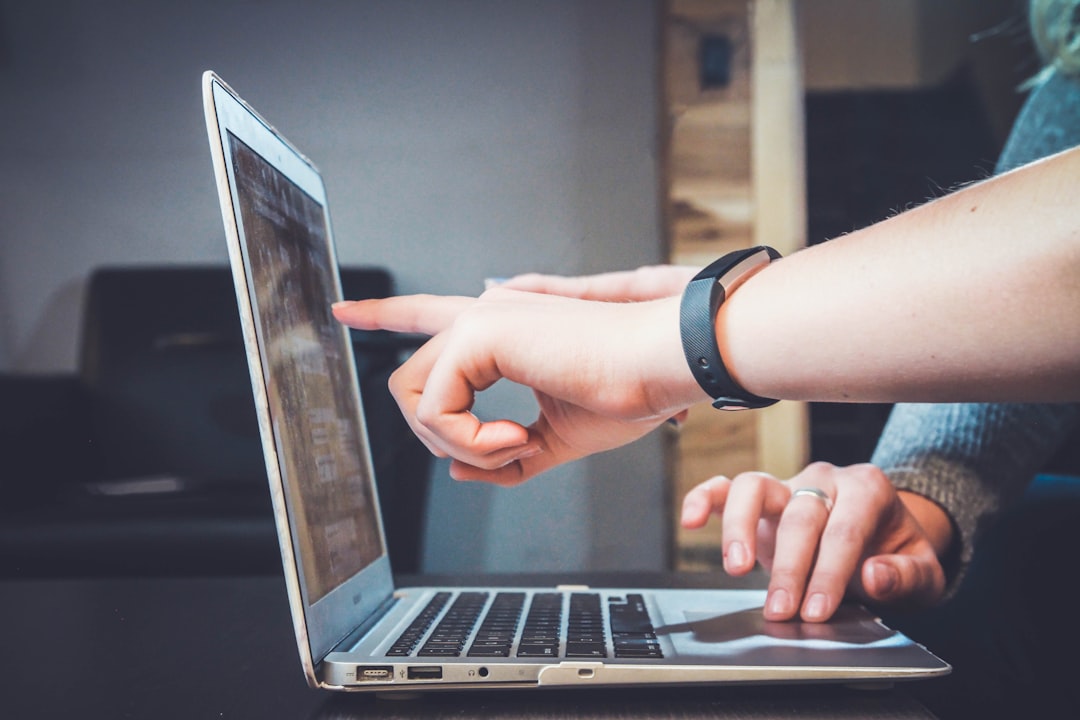
The application review process in insurance risk assessment involves a delicate dance between two key players: underwriters and actuaries. Underwriters act as the individual risk detectives, poring over personal information like medical records and financial statements to understand the unique risks associated with each applicant. They then use this insight to determine the terms and pricing of a potential insurance policy. Meanwhile, actuaries, the data wizards of the insurance world, analyze massive datasets to identify broad risk trends and develop statistical models. These models help determine appropriate premium levels for different risk categories, creating a framework for the underwriting process. It's this constant interplay between the individual-focused approach of underwriting and the statistical insights of actuarial science that ensures a balanced approach to risk management, allowing insurance companies to both cater to individual needs and maintain financial stability. As the world of work changes and technology advances, the collaboration between these two professions is more crucial than ever.
The application review process in insurance involves a fascinating interplay between underwriters and actuaries, each with their unique perspectives and methodologies. While we've already explored the core differences in their approaches to risk assessment, there are some surprising facets to their roles that deserve a closer look.
Firstly, underwriters are not just number crunchers; they also rely on their intuition and experience to evaluate risks. They often have to make calls based on incomplete or ambiguous information, adding a human element to the process. This is particularly important when considering the impact of emerging data sources, like social media activity, which can offer a more nuanced view of an applicant's risk profile.
Actuarial science, meanwhile, takes a long-term view. Actuaries build models that project risks and financial outcomes decades into the future, acting as financial stewards for insurance companies. Their work is subject to rigorous regulatory scrutiny, ensuring that their models are not only accurate but also compliant with complex legal frameworks.
The use of technology has drastically transformed the speed of application processing. Underwriters, empowered by advanced algorithms, can now evaluate an application in minutes, a far cry from the days of lengthy manual reviews. However, it's crucial to remember that even the most sophisticated models have limitations, and there's still a need for human judgment and adaptability to unforeseen events.
As the world evolves, so too must the methods used to assess risk. The rise of remote work, for instance, has created new challenges for underwriters, requiring them to continuously adapt their criteria. Furthermore, cybersecurity concerns are now an integral part of risk assessments, as insurers recognize the potential impact of cyberattacks on their policyholders.
Both underwriters and actuaries are increasingly incorporating insights from behavioral economics to understand how human biases and decision-making impact their assessment of risk. This is leading to more targeted and personalized pricing strategies that cater to individual preferences.
The insurance world is a dynamic landscape, constantly evolving to meet new challenges. The ever-changing nature of risk and the ever-increasing complexity of data requires a blend of human expertise, advanced technologies, and a willingness to adapt. Understanding the nuanced roles of underwriters and actuaries is essential for appreciating how the industry navigates this complex and dynamic environment.
Underwriting vs
Actuarial Science Key Differences in Insurance Risk Assessment - Integration of Actuarial Insights into Underwriting Decisions
The integration of actuarial insights into underwriting decisions aims to improve risk assessment by combining the meticulous individual analysis done by underwriters with the broader statistical data used by actuaries. By incorporating actuarial data, insurers can create more accurate pricing and policy terms that are consistent with statistical trends. This collaboration enhances understanding of risk, especially as both fields grapple with emerging technologies and shifting market dynamics. However, it's crucial to assess how these insights are implemented, ensuring that the human element of underwriting, like understanding individual applicant behavior, doesn't get lost in the data-driven approach. The careful integration of actuarial insights can promote transparency and fairness in the underwriting process, benefitting both insurers and policyholders.
The integration of actuarial insights into underwriting decisions is an ongoing challenge. Despite the power of actuarial modeling, underwriters often struggle to fully incorporate those statistical analyses into their own decision-making. This can lead to inconsistencies in risk assessment and pricing, potentially affecting the insurer's bottom line.
There's a tension between the reliance on complex algorithms by actuaries and the human intuition employed by underwriters. While actuaries focus on objective data analysis, underwriters frequently rely on their experience to navigate ambiguous situations. This difference underscores the crucial role of human judgment in risk evaluation.
Insurance risks are often fractal in nature, meaning similar patterns emerge at different scales. This complexity can be a hurdle for actuarial models, which may not fully capture these nuanced variations.
Behavioral economics is increasingly influencing underwriting, as professionals now recognize the impact of psychological factors on policyholder behavior. This can significantly shift how risk is perceived and assessed, potentially leading to greater reliance on insights beyond traditional data.
The rapid pace of data generation presents a unique challenge. While actuaries are adapting their models to analyze real-time data, underwriters may struggle to keep up with emerging risks stemming from evolving economic conditions.
The increasing use of unstructured data, such as social media activity, offers both opportunities and challenges. It allows underwriters to gather deeper insights but also raises ethical and privacy concerns regarding data utilization.
The gig economy further complicates risk assessment for both underwriters and actuaries. Traditional methods often struggle to accommodate the variable employment patterns and income volatility experienced by gig workers.
The regulatory landscape can create imbalances. While actuarial models are subject to strict oversight, underwriters may face fewer constraints, potentially leading to inconsistencies in risk management practices.
The utilization of advanced algorithms allows underwriters to adjust premiums more frequently, reflecting both current and projected risk changes. While this offers greater flexibility, it may result in volatile pricing environments for consumers.
Finally, actuaries typically focus on long-term risk prediction, while underwriters prioritize short-term risk-reward scenarios. This divergence in perspective can significantly impact how each profession assesses success and failure in policy issuance.
AI Insurance Policy Analysis and Coverage Checker - Get Instant Insights from Your Policy Documents (Get started for free)
More Posts from insuranceanalysispro.com: