Data Analytics and AI Reshaping the Underwriter's Toolbox in 2024 and Beyond
Data Analytics and AI Reshaping the Underwriter's Toolbox in 2024 and Beyond - AI algorithms revolutionize traditional underwriting methodologies
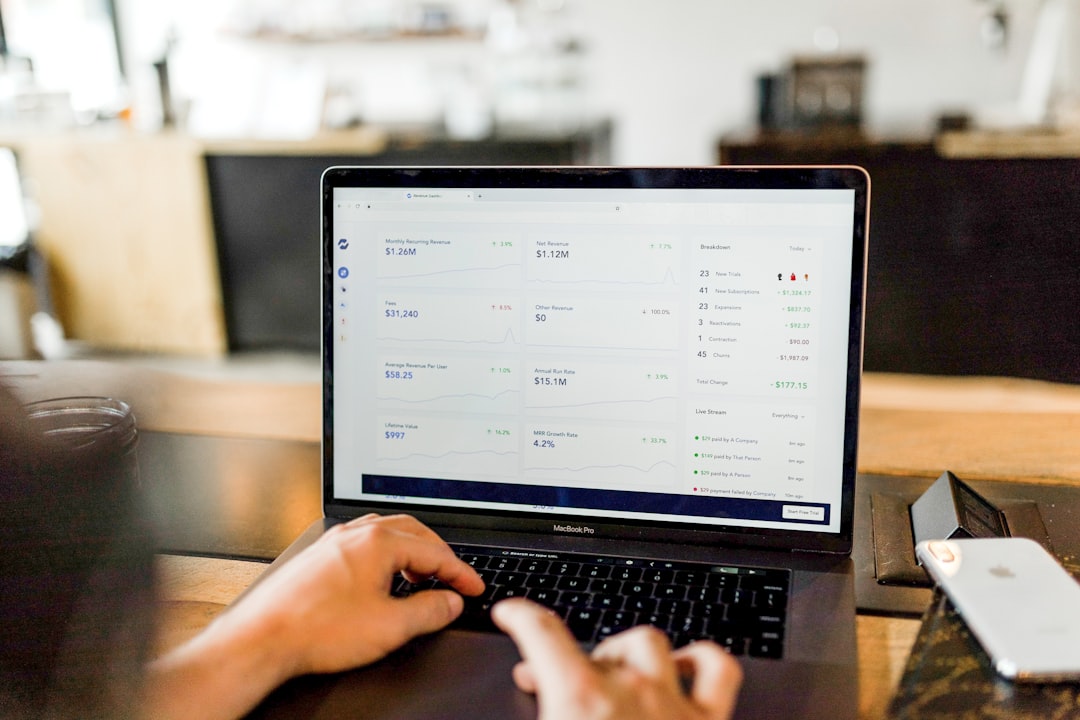
AI is fundamentally changing how insurers evaluate risk. Gone are the days of relying solely on experience and gut feeling. Today, insurers are using AI algorithms to crunch massive amounts of data, creating more accurate risk assessments and pricing models. This shift is especially apparent in commercial and specialty insurance, where AI helps streamline complex processes and incorporate advanced analytics.
However, this move towards a data-driven approach presents its own challenges. Insurers often struggle to access and consolidate data scattered across different systems and formats, making it difficult to fully utilize the potential of AI.
AI is shaking up the way insurance companies evaluate risk. Traditionally, underwriters relied heavily on historical data, but AI algorithms can process real-time data. This means they can adjust risk assessments instantaneously, potentially leading to more accurate decisions. The speed with which AI can analyze data is astonishing; studies have shown that AI systems can reduce underwriting time by a whopping 80%, which could mean insurance providers handle more applications without sacrificing quality.
What’s more, AI algorithms can go beyond simple pattern recognition, using advanced machine learning to uncover subtle patterns in data. This means they can identify risk factors that might escape human underwriters, giving them a more complete picture of the risks involved.
The ability to analyze unstructured data, like social media activity, is another exciting prospect. This allows AI to gather insights into consumer habits and lifestyle choices, going beyond traditional metrics like credit scores. However, this also raises questions about privacy and whether these insights are ethically used.
There are also concerns about the transparency and fairness of AI algorithms. They can be susceptible to manipulation, where malicious inputs could potentially skew assessment results. This creates a risk of incorrect underwriting decisions.
The increasing complexity of AI algorithms could also attract increased regulatory scrutiny. We'll need to ensure that the decisions these algorithms make are transparent and fair, especially considering the impact they have on individual lives. Overall, it’s clear that AI is poised to transform underwriting, but it’s crucial to navigate the ethical and practical challenges it presents.
Data Analytics and AI Reshaping the Underwriter's Toolbox in 2024 and Beyond - Advanced analytics enhance risk assessment accuracy
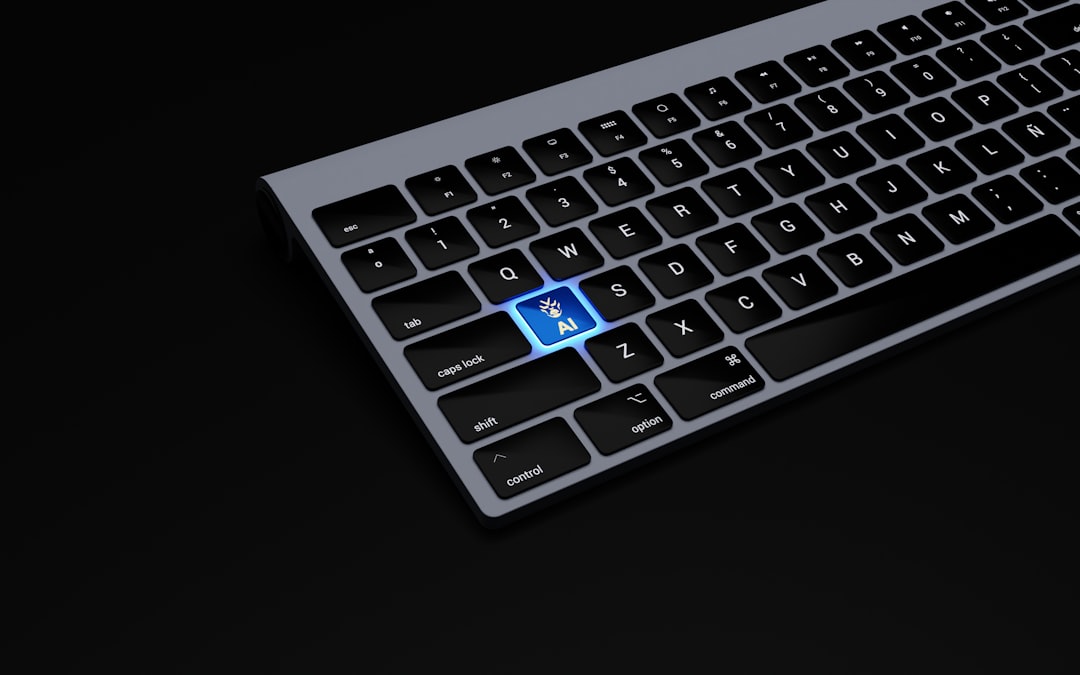
The way insurance companies evaluate risk is being revolutionized by advanced analytics. This means going beyond traditional methods and harnessing the power of massive datasets and sophisticated algorithms. Underwriters are now able to glean deeper insights into customer behavior, risk patterns, and market trends. This allows them to offer more personalized insurance products, something that was impossible just a few years ago.
One of the most significant developments is the ability to analyze real-time data, including unstructured information. This allows AI systems to uncover subtle patterns and nuances that might escape human underwriters, ultimately leading to more accurate assessments.
However, the increasing reliance on these sophisticated systems brings its own set of challenges. There are legitimate concerns about transparency, data integrity, and the ethical implications of these technologies. We need to ensure that these powerful tools are being used responsibly and that the decisions they drive are transparent and fair.
As we move forward, striking the right balance between utilizing the immense potential of advanced analytics while addressing the risks it presents will be crucial for the insurance industry. Only then can we truly harness the power of data to improve risk assessment and create a fairer and more sustainable insurance landscape.
Advanced analytics are enhancing risk assessment accuracy in a variety of ways. The ability to analyze real-time data from sources like telematics devices allows insurers to go beyond simply looking at historical trends and get a more granular understanding of individual behaviors. This can lead to more personalized risk assessments and potentially more accurate pricing. For instance, a driver with a consistently safe driving record could receive a lower premium than someone with a history of speeding or accidents.
Predictive modeling is another powerful tool that uses past claims data to forecast future risks. By analyzing millions of claims, insurers can better understand the factors that contribute to certain types of accidents and losses. This knowledge can be used to identify high-risk individuals or groups and take appropriate actions to mitigate potential claims.
The use of machine learning is particularly noteworthy because it allows for the analysis of vast datasets with a level of precision that often surpasses traditional statistical methods. These algorithms can handle thousands of variables simultaneously, uncovering complex interdependencies between risk factors that would otherwise remain unnoticed. For example, a machine learning algorithm might identify a correlation between specific weather patterns, the type of vehicle driven, and the likelihood of a certain type of accident.
However, the use of advanced analytics also raises some important questions. For example, the reliance on external data sources like public records and satellite imagery raises concerns about privacy and data security. Moreover, while AI can help to identify fraudulent claims, there are concerns about the ethical implications of using AI to make decisions about individual's lives. In the end, advanced analytics is a powerful tool that can be used to improve risk assessment, but it's important to be mindful of its limitations and potential pitfalls. As a curious researcher/engineer, I am excited to see how advanced analytics will continue to evolve in the coming years and how it will reshape the insurance landscape.
Data Analytics and AI Reshaping the Underwriter's Toolbox in 2024 and Beyond - Groundspeed AI delivers structured data in two hours
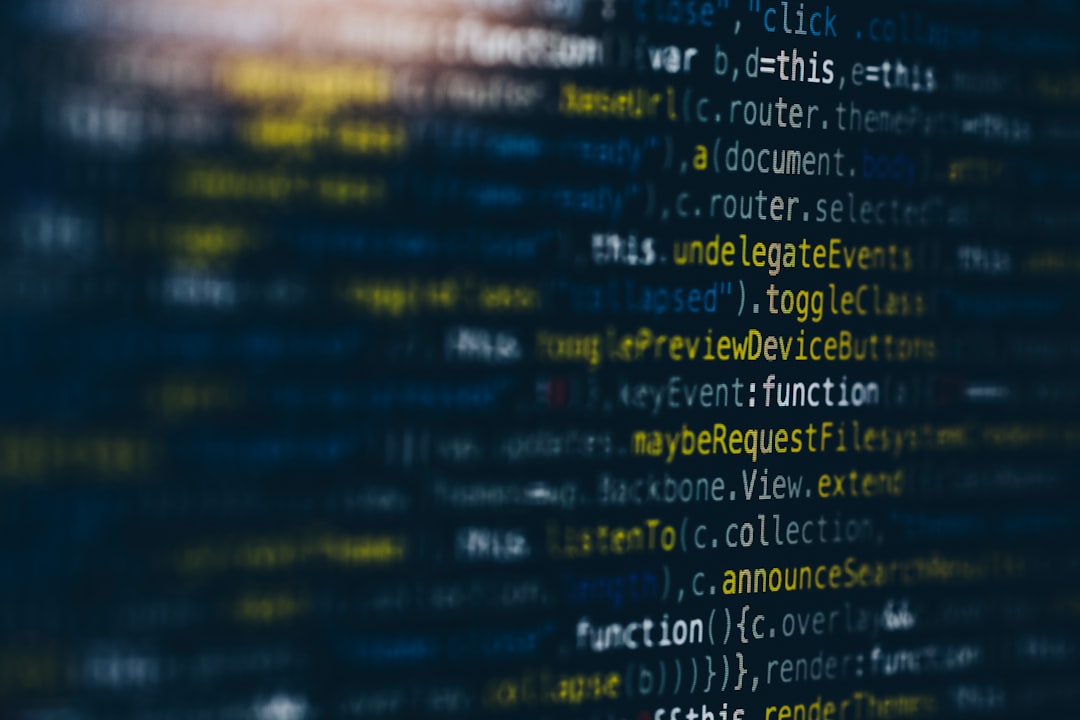
Groundspeed AI boasts the ability to deliver structured data to underwriting teams in a remarkably short timeframe - under two hours. This rapid turnaround, achieved through a combination of API and SFTP methods, positions the platform as a potential disruptor in traditional underwriting practices. Groundspeed AI distinguishes itself by drawing on a variety of external data sources, analyzed through advanced analytics, to provide underwriters with a more comprehensive understanding of risk. The company's Loss Run Automation Technology is noteworthy for its ability to convert unstructured data from a range of documents into a readily usable format, which could translate to more informed and efficient underwriting decisions. While this level of speed and data processing power could usher in a new era of profitability in the industry, its implementation raises important questions about data privacy, security, and the potential impact on the overall underwriting process. The industry must carefully consider the potential ramifications of such rapid data transformation before fully embracing this innovative technology.
Groundspeed AI promises a dramatic shift in the way underwriters handle data. They claim to be able to structure data from various sources in just two hours. This is remarkable, considering the traditional process of meticulously sifting through and organizing data often took days, if not weeks. The speed at which Groundspeed AI can handle information is impressive. But I'm still a bit skeptical.
It's not just about speed; it's also about the volume of data. Groundspeed AI claims to be able to manage huge datasets, which could mean underwriters finally have the potential to quickly process and analyze the deluge of information they encounter daily. This could mean a significant reduction in the backlog of information that often bogs down the underwriting process, leading to faster and more efficient decisions.
The possibility of real-time analysis based on the latest data is another interesting aspect. If they can achieve this, underwriters would be able to assess risk using the most up-to-date information, a potential game changer for the industry.
There are a lot of questions that come to mind, though. For one, what type of data can Groundspeed AI handle? I imagine they have some specific requirements, and I would want to know exactly what those are. I'm also curious to know how they deal with inconsistencies between data from different sources. This is crucial for building accurate risk assessments.
Then there's the issue of scalability. The insurance industry is constantly generating more and more data. Will Groundspeed AI be able to keep up with this growth without compromising speed or accuracy? This is crucial for ensuring that the technology remains valuable in the long run.
It's still early days, but Groundspeed AI's claims are certainly intriguing. Their technology could help insurers make better decisions and unlock the full potential of advanced analytics. But it remains to be seen if they can deliver on their promises, especially as we move into an era of even more complex and rapidly changing data landscapes. This technology deserves further scrutiny to understand its true potential and limitations.
Data Analytics and AI Reshaping the Underwriter's Toolbox in 2024 and Beyond - Automation streamlines underwriting tasks for faster risk evaluation
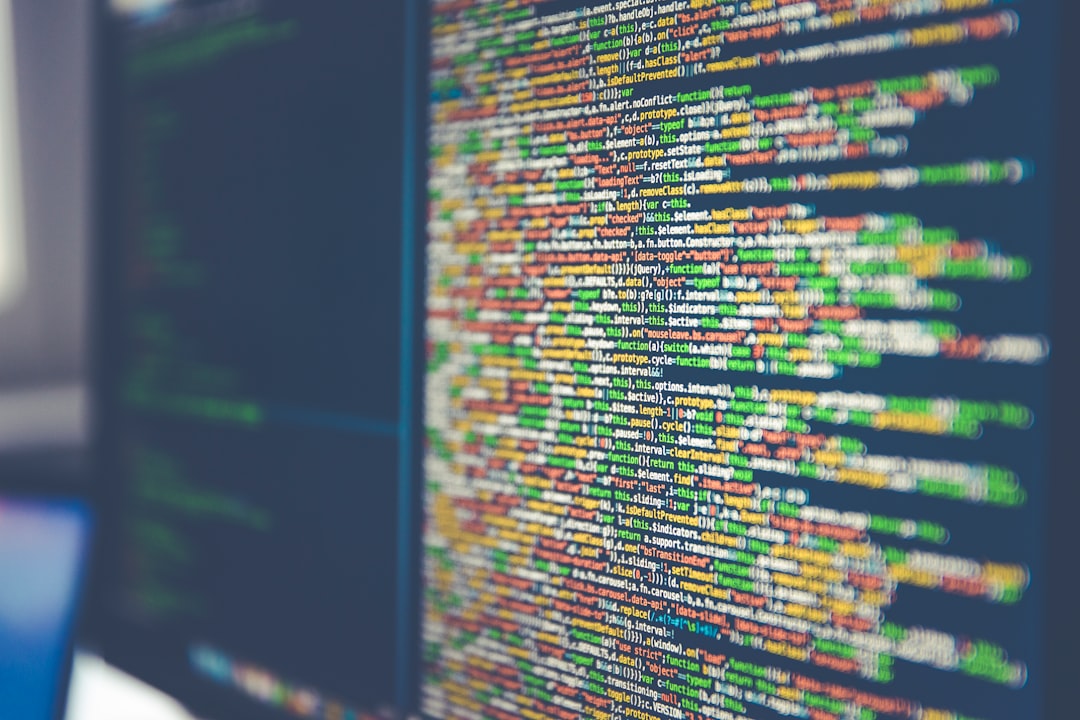
The underwriting process is undergoing a significant transformation, thanks to automation. This has resulted in faster risk evaluations, allowing insurers to handle tasks in minutes that once took days, or even weeks. AI and machine learning are playing a central role, streamlining data-intensive workflows and giving underwriters instant access to critical insights. This shift towards a data-driven approach enables insurers to respond to policyholders more quickly and makes decisions based on concrete analytics rather than gut feeling.
However, this rapid progress introduces its own set of challenges. Questions surrounding data privacy, and the ethical implications of algorithms making decisions, are paramount. Insurers must carefully consider the impact these technologies have on individual lives and work to ensure the decisions driven by these tools are fair and transparent. The insurance industry must strike a delicate balance between the speed and efficiency offered by automation and the ethical considerations it raises. The future of the industry hinges on its ability to successfully navigate these complexities.
The way insurance companies handle underwriting is being drastically changed by automation, a trend that is significantly speeding up the evaluation process. The impact of this is remarkable – on average, insurers are now able to process applications 80% faster than before. This means a policy can be issued much quicker, which directly affects how happy customers are.
But this isn't just about getting things done faster. AI and automation systems are surprisingly adept at dealing with unstructured data, the type that makes up a large chunk (around 80%) of data in the insurance industry. This is a real advantage for getting a more complete picture of someone's risk profile.
These systems are capable of using multiple data sources at once, painting a much broader view of an applicant than ever before. They can look at things like medical history, their behavior, and even their social media posts to reveal insights that could easily be missed by traditional methods.
This emphasis on objective data inputs is important. Automation can potentially lead to more impartial decisions, since it can filter out human bias. But there are valid concerns that AI can't fully replicate the nuanced understanding that a human underwriter brings to the table.
We're already seeing the effects of this shift on the workforce – some studies have found that insurance companies have been able to reduce their underwriting staff by as much as 25% thanks to automation. This is pushing the industry towards a much more tech-focused workforce.
Not only is it faster, but the rapid risk evaluation done by automated systems allows underwriters to adjust policies on the fly based on real-time data. This means the accuracy of pricing models is far better than the static, less dynamic methods used before.
Automation is also improving the accuracy of underwriting, as it can avoid many of the human errors that can occur during data handling. You see, fatigue and information overload can cause human underwriters to miss key indicators, which automation can address.
It's not just about better accuracy, but better fraud detection, too. These systems can analyze massive datasets in real time, identifying suspicious patterns that humans might miss, leading to stronger protection against false claims.
With automation taking care of the routine tasks, underwriters can now concentrate on the more complex cases. This could potentially improve job satisfaction for underwriters, as they'd be able to engage in more strategic decision-making rather than just processing information.
However, there is a risk of creating a gap in the knowledge of traditional underwriting practices, as fewer people will have practical experience with the old methods. This could be a challenge if automated systems ever need to be backed up by human intervention.
Data Analytics and AI Reshaping the Underwriter's Toolbox in 2024 and Beyond - Predictive analytics models analyze high-volume data on risks
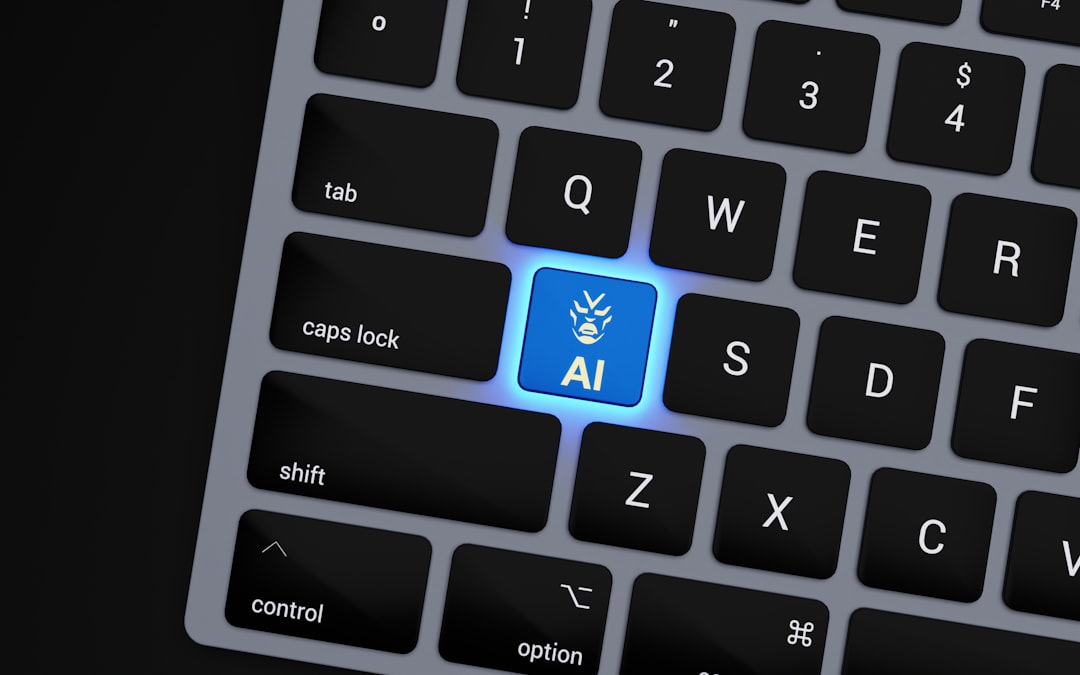
Predictive analytics models are rapidly becoming essential in the insurance industry, especially when it comes to analyzing massive amounts of risk-related data. These models can quickly pinpoint high-risk situations by identifying patterns in historical data. They're essentially helping underwriters get a better grasp on potential exposures. With the advancement of machine learning, these models can now even handle unstructured data, giving underwriters a more complete understanding of risk. This move towards data-driven decision-making raises important concerns though. We need to be sure these models are transparent and their outputs are ethically sound. The insurance industry has a lot to gain from predictive analytics, but making sure it's done responsibly is crucial.
Predictive analytics models are revolutionizing how insurers assess risk, especially in the wake of data-driven approaches becoming increasingly common. It's fascinating to see how these models can analyze massive amounts of data in a fraction of the time traditional methods would take. This speed isn't just about efficiency; it allows insurers to quickly adapt to changing circumstances.
The types of data these models process are also fascinating. We're seeing a move beyond traditional metrics like credit scores to incorporate unconventional sources like satellite imagery or sensor data. This opens up a whole new world of insights into behavior patterns, allowing for more accurate assessments.
Furthermore, these models are becoming increasingly sophisticated, using advanced algorithms to detect anomalies, identify fraudulent claims, and even forecast risk events. This opens the door to truly proactive risk management.
However, as with any powerful technology, ethical considerations come into play. We need to be vigilant about ensuring that these models don't perpetuate biases and that their decisions are fair.
I find it particularly exciting to see how these models are being used not only for pricing but also for regulatory compliance. This could significantly streamline the industry and make it more transparent.
Overall, it's clear that predictive analytics are dramatically altering the insurance landscape, However, as an engineer with a curious mind, I'm eager to see how the industry will grapple with the ethical challenges and ensure that these powerful tools are used responsibly.
Data Analytics and AI Reshaping the Underwriter's Toolbox in 2024 and Beyond - AI reduces non-core task time for underwriters from 40%
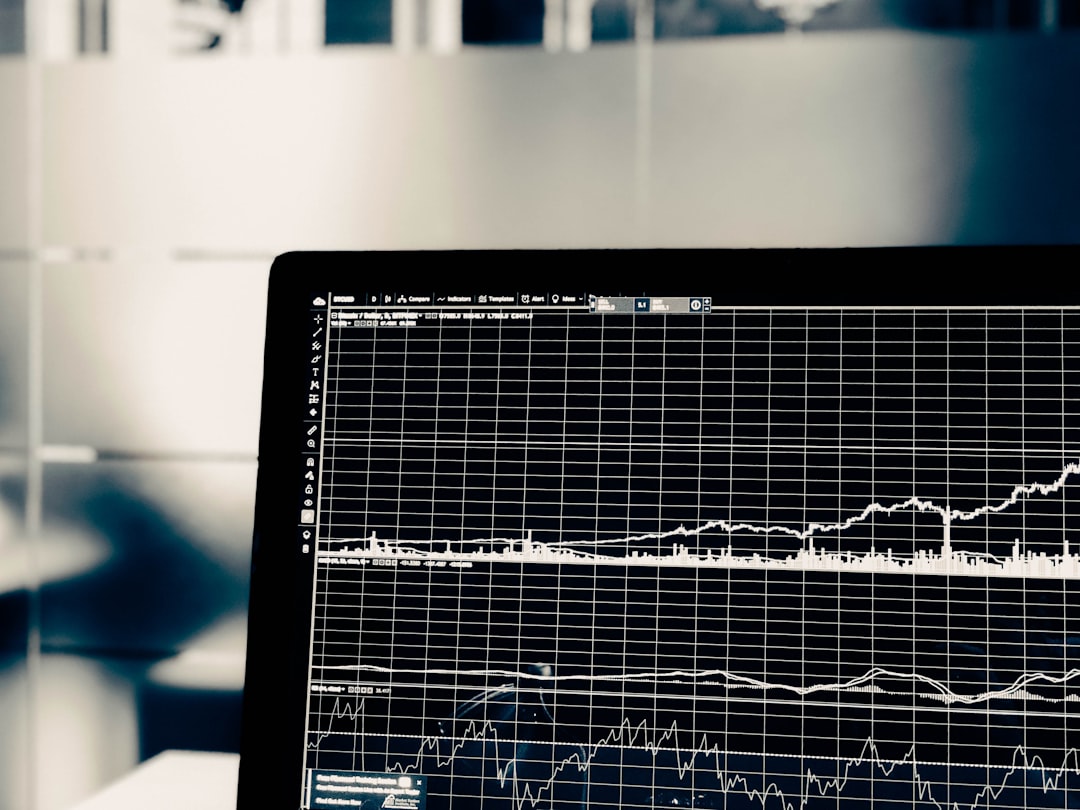
Artificial intelligence (AI) is transforming the way underwriters work by significantly reducing the time they spend on routine, non-core tasks. This can free up as much as 40% of an underwriter's workday, allowing them to focus on more complex, nuanced risk assessments that require human judgment. This shift towards a more data-driven approach, where AI handles repetitive tasks, means underwriters can spend more time on tasks that require their expertise and experience. While the potential for increased efficiency is huge, there are also concerns about the ethical implications of AI-driven decision-making and the potential for underwriters to become less familiar with the intricacies of traditional underwriting methods. The future of the insurance industry will likely involve finding the right balance between human expertise and the power of AI, to ensure a more efficient and ethical approach to risk evaluation.
While I'm impressed by the speed at which AI can analyze data – it's cutting down on the time underwriters spend on tedious tasks by up to 40% – I'm also a bit skeptical. I see a clear benefit to having more time for analysis, but how much of this time will actually be spent on high-level work instead of just "busy work" generated by the AI?
I'm curious about how AI is handling unstructured data. Is it really getting a grasp on the complex details that could be missed by traditional methods, or just spitting out generic reports based on superficial data? We need to be sure the AI isn't creating a false sense of certainty, especially when working with complex, nuanced situations.
I'm excited about the potential for AI to improve predictive analytics and adjust pricing models in real-time. It could be a huge step towards more accurate assessments. However, I'm also worried about the potential for bias. We need to be careful to make sure that these powerful tools are used responsibly and fairly. I hope that, as we continue to see AI integrated into underwriting, we'll also see more robust ethical safeguards put in place.
More Posts from insuranceanalysispro.com: