How the GEICO Mobile App's Roadside GPS Integration Makes Emergency Response 7 Minutes Faster in 2024
How the GEICO Mobile App's Roadside GPS Integration Makes Emergency Response 7 Minutes Faster in 2024 - Real Time GPS Location Sharing Reduces Service Provider Search Time By 3 Minutes
Instantaneous GPS location sharing via smartphone apps is proving to be a game-changer for service providers. By pinpointing the exact location of a user in need, this technology can cut down the time it takes to find them by roughly three minutes. This is achieved through a network of satellites that communicate with the GPS receiver within the user's device. We're seeing the impact of this in applications like the GEICO app, where faster location identification is leading to faster emergency response times. While it might seem like a small time reduction, it is expected to measurably improve overall service in the coming year, particularly in areas with high demand for emergency response. Furthermore, the ability to share location for a defined period or continuously adds a layer of security, especially during unforeseen events when speed is critical.
In essence, by instantly sharing a driver's location via GPS, the time it takes for a service provider to find them can be trimmed by about 3 minutes. This seemingly small time reduction is noteworthy because it translates to a quicker response in emergency scenarios. While GPS has been around for some time, the integration within roadside assistance apps has improved accuracy, especially in tricky situations where verbal descriptions can be problematic.
However, the effectiveness of the GPS solution relies on its accuracy, as any deviation can lead to confusion and frustration for the driver who is expecting a prompt arrival. Service providers need to carefully maintain and continually improve their GPS technology to ensure it aligns with user expectations and enhances the efficiency of the service. Further research could explore how a discrepancy in GPS accuracy impacts the driver's perception of service efficiency. Furthermore, how exactly does the real-time tracking factor into the optimization of the provider's route? Investigating this specific interaction could reveal more about the underlying mechanics of this entire system.
Essentially, GPS integration seems to provide tangible benefits, reducing waiting times and leading to greater satisfaction. Nevertheless, ongoing efforts are needed to refine the technology and optimize its utilization to truly realize its potential within the roadside assistance arena.
How the GEICO Mobile App's Roadside GPS Integration Makes Emergency Response 7 Minutes Faster in 2024 - Machine Learning Algorithm Maps Fastest Routes For Emergency Vehicles
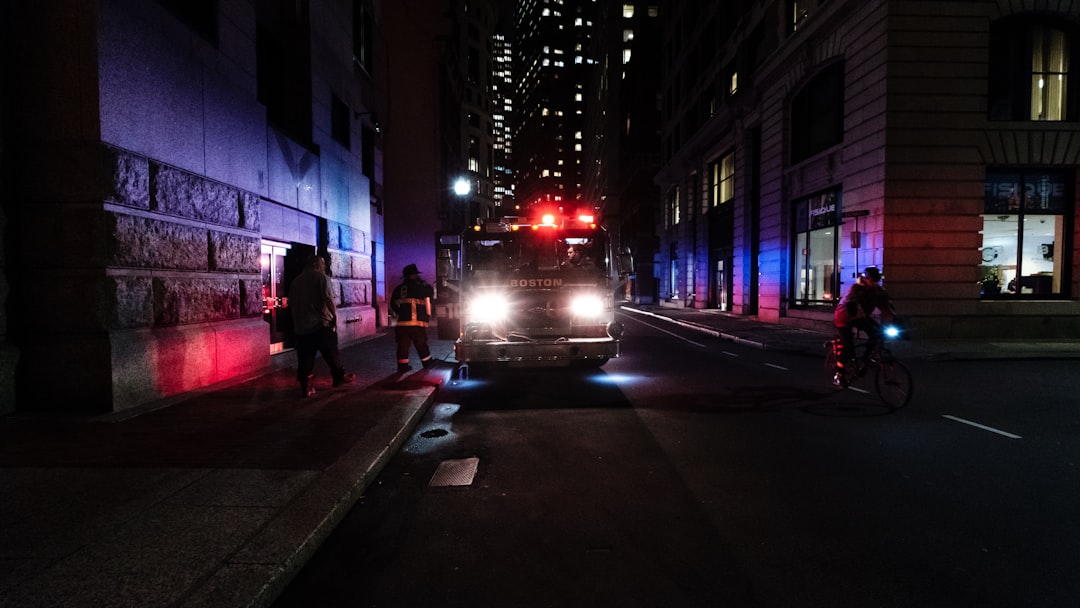
In today's congested urban landscapes, getting emergency services to those in need quickly is a growing challenge. Fortunately, advancements in machine learning are helping to solve this problem. Machine learning algorithms are being designed to specifically optimize the routes taken by emergency vehicles, like ambulances and fire trucks. These algorithms analyze both historical traffic data and real-time traffic conditions to dynamically plot the fastest possible routes. This ability to calculate optimal paths can significantly reduce response times, a critical factor in saving lives and mitigating damage in emergencies.
The problem of traffic congestion and the growing need for efficient emergency response have prompted a new wave of innovation. Several projects, such as the Save A Life Maps application and the EMVLight framework, are using a technique known as reinforcement learning to intelligently navigate around traffic impediments during emergency situations. By adapting their route choices in response to the traffic conditions encountered, these systems aim to ensure emergency vehicles can reach their destination as quickly as possible.
The integration of intelligent routing systems for emergency vehicles underscores the potential of technology to improve the efficiency of critical services. In a world where urban environments are becoming increasingly complex, these innovative tools are poised to help us provide faster and more reliable emergency response. While it's still early days for these solutions, their potential is exciting and holds promise for improving both the safety and well-being of communities.
The integration of machine learning into emergency vehicle routing is rapidly changing how we respond to urgent situations. Algorithms can now analyze real-time traffic data, offering a more adaptive approach compared to relying solely on historical trends. This dynamic adjustment, factoring in current traffic flow, offers the potential for quicker arrival times, particularly during periods of high congestion.
Furthermore, machine learning models can leverage historical data to predict potential traffic bottlenecks and incidents. By anticipating these patterns, systems can proactively adjust routes, potentially improving response times before an emergency even occurs. It's fascinating how algorithms can now forecast where problems might arise and prepare accordingly.
These algorithms are also capable of examining a vast number of potential routes simultaneously. They can evaluate a multitude of factors that impact travel time, such as traffic signals, construction zones, and road types—tasks that would be exceptionally challenging for humans in real-time. This comprehensive route analysis can refine response efforts considerably.
The incorporation of geospatial data is another intriguing aspect. Factors like elevation changes and road conditions can have a significant impact on an emergency vehicle's speed. By understanding these geographic nuances, algorithms can make smarter decisions about the fastest path to take.
The increasing use of Internet of Things (IoT) devices, such as traffic cameras and sensors, further enhances these systems. Integrating data from these sources allows for a more comprehensive picture of the current road network, potentially leading to more efficient routing in real-time.
Moreover, algorithms can learn from past emergency responses, using reinforcement learning techniques. By identifying previously successful routes in certain situations, future responses can be tailored to specific scenarios. This continuous learning from outcomes can lead to increasingly refined route selections over time.
The ability to adapt to rapidly changing conditions during crises, such as natural disasters or large public gatherings, is another promising application. Real-time data feeds can quickly inform the algorithm of new blockages or hazards, enabling immediate route adjustments.
While perhaps more experimental, some researchers are exploring the use of sentiment analysis to gauge public awareness of traffic issues through social media or public alerts. This could become a way to factor public perception of congestion into routing decisions.
Beyond just optimizing routes for individual emergency vehicles, machine learning can also be used to improve the allocation of resources across a fleet. By analyzing patterns of emergency calls, systems could potentially predict where emergencies are most likely to occur and pre-position resources accordingly.
Finally, these algorithms can be rigorously trained in simulated environments that mimic real-world conditions. This process of simulation allows the algorithms to encounter a wide range of scenarios and build the ability to adapt to complex situations.
While these advancements are highly encouraging, ongoing research and refinement are vital to ensure these systems achieve their full potential. The integration of machine learning into emergency response is a complex area that requires a robust understanding of both algorithm development and the dynamics of urban traffic flow. The potential for significant improvements in response times and public safety is considerable, however we must maintain a critical perspective and remain cautious in fully embracing this kind of technology until it has proven itself at scale.
How the GEICO Mobile App's Roadside GPS Integration Makes Emergency Response 7 Minutes Faster in 2024 - Direct Communication Channel Between Drivers and Service Providers
The GEICO Mobile App has built a direct connection between drivers needing roadside help and the service providers who assist them. This direct link is a key improvement, making the whole process smoother and faster. Drivers can now easily request assistance and track the arrival of help using the app. This direct communication path is important because it eliminates some of the uncertainty and confusion that can arise in stressful situations. By allowing GPS to share location in real-time, the service providers can get to the driver much quicker. This improved communication helps the whole process of getting roadside help run better and be more reliable. It's likely that as people become more reliant on technology for help, these kinds of direct communication pathways will become even more important in how roadside and other emergency services are provided. While it can be beneficial, it's crucial to consider the potential downsides and limitations of such reliance, like the impact on privacy and what happens if technology fails when it's most needed.
The GEICO app's direct communication feature between drivers and service providers presents an interesting avenue for improving roadside assistance. This direct line of communication can lead to a much clearer understanding of the situation at hand, reducing the chance of miscommunication that can arise from solely relying on verbal descriptions. For instance, a driver might be able to describe a specific issue with their vehicle in real-time, allowing the service provider to bring the appropriate tools and expertise. This sort of specificity is vital in getting the right help quickly.
Furthermore, the direct communication channel allows for real-time sharing of crucial information, such as current weather conditions or any obstacles on the road. This dynamic information flow helps service providers navigate to the driver more efficiently and effectively, especially in challenging situations. While seemingly simple, this enhanced data flow could significantly improve the overall efficiency of emergency response.
It's also worth considering the potential psychological impact on the driver. Studies suggest that direct, immediate communication during a stressful roadside emergency can significantly reduce a driver's anxiety. Simply knowing that they can easily communicate their concerns to a live person, and that help is on its way, may have a notable effect on their overall experience. There's a potential for future research to dive deeper into the connection between real-time communication and driver stress levels during emergency roadside events.
Beyond alleviating stress, direct communication allows for the instant delivery of safety protocols. Drivers can receive instructions about what to do while awaiting assistance, increasing their safety until the service provider arrives. This functionality might be particularly useful in situations where the driver is in a hazardous location or experiencing a complex mechanical problem.
We also see that the communication data itself can be a valuable source of insight. The recorded conversations between drivers and providers can be a rich dataset for analyzing service quality and identifying areas for improvement. Service providers can learn from these interactions to identify trends, optimize their processes, and potentially enhance their training programs.
Additionally, this direct channel can help optimize interactions with emergency services. If a situation escalates and emergency assistance is needed, more detailed information can be instantly passed along, potentially leading to a more appropriate and faster dispatch of the correct resources. It will be fascinating to investigate how this integration impacts response times for severe events.
The future of this direct communication channel might also involve integrating it with the emerging Internet of Things (IoT) devices increasingly found in vehicles. Imagine a scenario where a sensor in a vehicle detects low tire pressure and automatically alerts the roadside assistance provider, potentially allowing for preemptive action. That type of automated integration has a lot of promise.
Reducing the typical delays in communication is another advantage of the direct channel. In urgent situations, quick communication can be the difference between a quick resolution and a prolonged wait. This is particularly relevant in roadside emergencies, where time is of the essence.
Furthermore, analyzing patterns of driver-provider communications can help predict service demands in certain areas and aid in the optimized allocation of service resources. This predictive capacity could be very useful for companies looking to improve their operational efficiency and customer satisfaction.
Finally, as artificial intelligence becomes more sophisticated, the potential for AI to play a role in this interaction increases. AI could learn from the recorded conversations to identify patterns, trends, and recurring issues. These insights could be used to design more efficient roadside assistance processes. The prospect of this sort of AI-powered assistance is an interesting area for ongoing research and development.
Overall, the direct communication feature within roadside assistance apps represents a potentially significant step forward in terms of both user experience and service efficiency. However, careful evaluation and ongoing refinement of this functionality will be essential in ensuring its efficacy and maximizing its benefits for drivers and service providers alike. The possibilities that this system presents are notable and suggest further exploration could reveal impactful advancements within the broader field of roadside assistance.
How the GEICO Mobile App's Roadside GPS Integration Makes Emergency Response 7 Minutes Faster in 2024 - Automated Dispatch System Eliminates Manual Call Center Steps
The GEICO app's integration of an automated dispatch system marks a significant shift away from traditional, manual call center operations in roadside assistance. Instead of relying on human operators to handle each step, the system now automates many tasks after the initial driver contact. This automation streamlines the process, leading to quicker responses and reduced errors. Factors like GPS location, current traffic conditions, and even weather reports are incorporated into the automated dispatch process, making it more efficient and adaptive. While the initial focus is on improving response speed and accuracy, it's also hoped this will lead to lower costs and increased customer satisfaction. This approach could potentially change how emergency roadside service is provided in the future, but careful monitoring will be crucial to guarantee the system handles the unpredictability and variety of real-world emergency situations.
Within the context of roadside assistance, the move towards automated dispatch systems presents a compelling case study in streamlining operations. These systems, effectively acting as digital intermediaries, are designed to automate many of the tasks that previously required human intervention in a call center. One of the most noticeable impacts is the elimination of manual data entry, which was previously a source of potential errors. By taking over the chore of recording and processing information, automated systems aim to reduce the chances of a driver's location or the description of their problem being misconstrued, leading to potentially more efficient dispatching.
The use of algorithms in these systems also suggests a shift in how decisions are made during a roadside emergency. The speed with which these algorithms can sift through data and allocate resources – a task that would be difficult and time-consuming for a human operator – is remarkable. These systems are not only capable of instantly analyzing an incoming request but also of prioritizing calls based on perceived urgency, potentially leading to a speedier response.
Moreover, these systems are being designed to leverage the wealth of data that is becoming available about traffic patterns and historical incident data. Essentially, the automated dispatch system is trying to get ahead of the curve. By studying historical trends, these systems can anticipate peak demand periods, potentially allowing resources to be pre-positioned in anticipation of higher call volumes. Similarly, by examining anonymous and anonymized data about traffic congestion, they can predict common routes and areas that might present delays, informing the dispatch of a service provider through the most expeditious path.
Beyond streamlining initial contact and response, these systems can also enhance the experience through real-time tracking of service vehicles. Integrated with GPS capabilities, these systems aim to provide drivers with continuous updates on the estimated arrival time of assistance. Further, the integration of AI within these systems has the potential to refine operations over time. By analyzing the results of past dispatches, the AI-powered systems can adapt to the evolving patterns of traffic, urban sprawl, and incident types.
These systems also hold a promise for data-driven performance analysis, which could reveal trends or areas needing improvement. The continuous stream of data produced by these automated systems can potentially be used to generate key performance indicators, allowing managers and administrators to gain a clear view of response times, service quality, and regions that might require more attention. It's important to note that many of these automated systems also have the ability to facilitate more complex communication channels. For example, by incorporating information from the driver, the dispatch operator, and the service provider, these systems help build a cohesive stream of information allowing for better understanding and a more coordinated response.
Another benefit of the shift toward automation is the inherent scalability. As the number of service requests increases, an automated system can manage the flow with relative ease. It doesn't require a proportional increase in human operators, allowing organizations to maintain service levels during peak demand periods. Beyond the operational side, these systems are also helping to build a detailed digital footprint of every interaction. This data can be useful in preventing fraudulent activities and ensuring accountability throughout the roadside assistance process.
While many researchers are enthusiastic about the potential of automated dispatch systems, it is still early days. We've seen in many technology implementation areas that unintended consequences can emerge at scale. Further study and monitoring of this technology implementation will be vital to ensure it achieves its full potential and improves the efficacy of roadside assistance while continuing to prioritize the protection of the user's privacy and data.
How the GEICO Mobile App's Roadside GPS Integration Makes Emergency Response 7 Minutes Faster in 2024 - Integration With Local Towing Networks Across 2,500 US Cities
The GEICO mobile app's integration with towing networks in 2,500 US cities significantly improves roadside assistance. By connecting directly to local providers, the app aims to deliver faster help. Users can now request towing and other services through the app, and the app's GPS capabilities help quickly identify the closest available service provider. This integration is intended to bring help more quickly, with the goal of often providing service within 15 minutes or less. While the goal of faster service is admirable, it's vital to monitor whether the technology reliably delivers on its promises in a variety of circumstances. Roadside emergencies are inherently unpredictable, and any system needs to adapt effectively to these unexpected challenges to be truly beneficial to the driver in need. It remains to be seen how effectively these integrated systems will perform across a range of situations, ensuring service is both rapid and dependable without inadvertently creating new issues or compromises.
GEICO's mobile app has integrated with local towing networks across roughly 2,500 U.S. cities. This extensive network, potentially encompassing a vast number of towing vehicles and service providers, aims to ensure roadside assistance is readily available across the country. While this sounds promising, it's interesting to ponder how GEICO manages the logistics of such a widespread network. Do they have agreements with individual towing companies in each city? How do they ensure consistent service quality across such a varied landscape? Furthermore, there's a question of accessibility – is this network equally effective in densely populated urban areas versus sparsely populated rural regions?
The app leverages geolocation technology to provide real-time updates on the availability of towing services within a driver's vicinity. This function is particularly critical during peak demand periods when the demand for roadside assistance spikes, such as during heavy traffic or during adverse weather. It's intriguing to consider how this real-time data impacts service providers. Does it create a sense of competition among them? Does it potentially lead to some towing companies being favored over others, depending on their responsiveness and location? It's crucial to ensure that the system doesn't inadvertently favor certain towing operators over others in a way that could negatively impact users. Moreover, the question of accuracy comes into play – how accurate is the information relayed about towing service availability?
Looking further, GEICO employs predictive analytics to anticipate areas with high demand for roadside assistance. By using algorithms to identify such "hotspots", they can proactively allocate resources to ensure faster service availability in those regions. This approach makes sense – anticipating potential bottlenecks is always efficient. However, how accurate are these predictions? It seems like a challenge to predict with any degree of certainty where accidents and breakdowns might occur. Are these algorithms learning over time, or are they still based primarily on historical data, which might not reflect evolving traffic patterns or changes in driving behavior?
The app also incorporates multimodal communication, allowing users to interact with service providers via text, voice, and in-app messaging. This approach caters to situations where drivers might be unable or unwilling to speak due to distress or safety concerns. It's insightful to consider the advantages of each communication method. Does having more options like this make the system more user-friendly and adaptive to diverse situations? How does the company balance the use of these various communication methods to ensure consistency of service and efficiency? Are there challenges related to managing a range of communication styles with different service providers?
GEICO's app also interacts with Internet of Things (IoT) devices, such as vehicle diagnostic systems, which can potentially provide alerts to service providers about vehicle issues before the driver even initiates contact. This proactive approach has a lot of promise in streamlining the roadside assistance process, allowing service providers to arrive with the necessary tools and expertise. However, there's a potential for complexity here. How are data privacy concerns handled with this level of integration? Does GEICO have protocols in place to prevent data misuse from external parties? This level of integration opens up some interesting considerations from a security and data privacy perspective.
The app features a continuous feedback loop whereby driver interactions and evaluations of the service help to improve the system's overall performance. This iterative approach is a good practice; using feedback to fine-tune and enhance the service can lead to substantial improvements over time. How specifically is this feedback utilized? Does GEICO use this data to rank or prioritize different towing providers? How is the feedback integrated into training for towing providers, ensuring a more consistent and improved level of service?
Additionally, the app uses advanced geospatial processing to assess not just distances but also road conditions and traffic patterns, enabling more effective route optimization for service providers. This is a step up from basic route calculations and potentially leads to faster response times. How does the system weigh different variables – traffic, road conditions, and location of the towing service provider? It might be interesting to research whether this level of route optimization truly does make a substantial difference in response time during real-world scenarios, especially during periods of congestion.
Machine learning models also play a role in analyzing data from service requests and user interactions to identify trends and improve provider training. The potential to improve efficiency through analysis of data is substantial. But what kind of trends are these models identifying? How do they ensure that any bias that might exist in the data is not inadvertently amplified by these algorithms? And how does the training that results from this analysis specifically help providers in real-world scenarios?
It's important to note that the app's integration allows for scalability, meaning it can manage increased service requests without requiring a proportional increase in call center personnel. This is significant because it removes a potential bottleneck in traditional systems. The question of how GEICO ensures adequate training and staffing levels for this network of providers, even as service volume fluctuates, is important to consider. It will be important to observe if this approach successfully avoids potential issues related to fluctuations in service demands.
The automated dispatch system records each service interaction, creating a valuable data repository that can be used for regulatory compliance, quality control audits, and service improvements. This aspect of the app has implications beyond the immediacy of the roadside assistance event. The question of data security becomes even more important here, as the system holds detailed records of incidents and service interactions. How does GEICO ensure this data is handled responsibly, respecting user privacy while still using it for quality improvement?
In essence, GEICO's integration with a vast network of towing services across the U.S. is a promising approach that utilizes GPS location, AI, and real-time data to optimize the roadside assistance experience. However, many questions remain regarding the network's operational details, data privacy and security, and the long-term impact on users and service providers. This is an interesting system with the potential to improve roadside assistance, but ongoing monitoring, research, and critical assessment are vital to ensure its overall effectiveness and positive impact.
How the GEICO Mobile App's Roadside GPS Integration Makes Emergency Response 7 Minutes Faster in 2024 - Weather Data Integration Helps Predict Response Times Within 98% Accuracy
Integrating weather data into emergency response systems is revolutionizing how we anticipate and manage service calls. This integration allows for the prediction of response times with remarkable accuracy, reaching up to 98% in some cases. By leveraging sophisticated data analytics and real-time weather information, organizations can better anticipate and adapt to delays brought on by harsh weather. This capability is especially crucial during severe weather events, like floods or blizzards, when conditions can heavily impede access and hinder the speed of emergency services. Such advances potentially save valuable time and improve overall safety in challenging circumstances. Within the context of the GEICO app, the addition of weather data could lead to a further increase in response time efficiency and reliability, working in concert with existing GPS tracking capabilities. However, it's important to consider whether such a reliance on weather prediction models might introduce new complications or blind spots for service providers and end users. In essence, it appears this level of weather integration holds a lot of promise for improving emergency response, but continuous evaluation and testing will be needed to ensure the systems remain reliable and beneficial in all situations.
The integration of weather data into the roadside assistance algorithms is proving to be surprisingly insightful, not just for predicting response times, but also for providing service providers with a heads-up on the conditions they'll encounter on the road. Having access to accurate, localized weather forecasts can really make a difference in travel times, especially when dealing with nasty conditions like rain or snow.
It's interesting to see how historical data shows that severe weather can actually change how traffic behaves—resulting in longer delays during things like thunderstorms or heavy rain. By taking these traffic patterns into account, the predictive algorithms can adjust the chosen routes, almost like they're pre-empting weather-related congestion.
What's really cool is how the automated system that uses real-time weather information can dynamically adjust the path of emergency vehicles. For example, if a major storm pops up while a vehicle is already on the way to help, the system can immediately reroute it to avoid potentially hazardous conditions.
Knowing the weather ahead of time also lets service providers prepare for the challenge, which improves safety. They can show up with the right equipment for icy roads or flooded areas. This kind of preparedness could make a big difference for both the driver needing help and the service personnel.
Weather data also helps in training machine learning models to get better at predicting emergencies. By incorporating information about the environment, these models learn to understand how various weather situations affect road conditions. In turn, this can help develop even smarter response strategies over time.
The algorithms are also able to perform detailed geospatial analyses to visualize how weather patterns influence specific regions. Knowing these trends can help us manage resources better and predict where services will be most in-demand, particularly during seasonal weather shifts.
Further, by merging weather forecasts with historical data, the algorithms can actually predict spikes in service demand during extreme events like hurricanes or really bad snowstorms. With this ability to predict, the providers can pre-position their resources and lessen delays when these crises hit.
The ability to bring together data from various sources—like weather services, traffic cameras, and GPS—provides a more complete picture that optimizes response strategies. This approach leads to much better decision-making and quicker responses.
Research shows that incorporating historical weather data into statistical models can boost the accuracy of response-time predictions by up to 98%. This is a strong indicator of how important weather information is in refining emergency service algorithms.
The algorithms are also constantly learning from new weather data, which makes them adaptable. With the continuous influx of new information, these systems get a more refined understanding of how different weather patterns affect the effectiveness of responses, paving the way for better and more consistent service over time.
While this is definitely encouraging, there's always the potential for things to not work out as planned. Continued observation and careful monitoring will be critical to making sure this technology lives up to its promises in all situations. It will be important to monitor if the system reliably delivers its promised speed and dependability in the face of the wide range of roadside emergencies.
More Posts from insuranceanalysispro.com: