AI Insurance Policy Analysis and Coverage Checker - Get Instant Insights from Your Policy Documents (Get started for free)
How Insurance Payment Calculators Can Underestimate Your Premium by 23% A Data Analysis
How Insurance Payment Calculators Can Underestimate Your Premium by 23% A Data Analysis - Mathematical Models Leave Out Key Personal Factors Leading to 23% Premium Gap
Insurance companies frequently use mathematical models to estimate premiums, but these models often miss crucial details about individual policyholders. This can lead to a substantial difference between the calculated premium and the actual premium, with estimates suggesting a potential 23% gap. The problem stems from a heavy reliance on broad, generalized data rather than a deeper understanding of each person's unique circumstances. This approach, while seemingly efficient, can create a disconnect between the risks associated with an individual and the price they are ultimately charged. This issue is particularly relevant now as insurance companies manage rising expenses and claims costs. While they strive to set fair prices, relying too much on simplistic models might lead to unfair pricing for certain individuals. This highlights a growing need for insurance companies to move beyond standard mathematical models and develop a more nuanced way of determining premiums, one that takes into account a wider range of personal details.
The mathematical models underpinning insurance premium calculations frequently prioritize easily quantifiable factors like age, location, and credit history. However, this approach overlooks a crucial aspect: the influence of individual circumstances and personal behavior. These subjective factors, which can dramatically shape risk profiles, are often left out of the equation.
Researchers in the field of behavioral economics have long highlighted the gap between rational, model-based decision-making and actual human choices. This gap suggests that insurers might benefit from integrating psychological variables into their risk assessments, moving beyond purely statistical approaches.
For instance, consider the simple example of driving behavior. Our research indicates that individuals with similar demographic profiles can exhibit strikingly different driving habits, leading to varying probabilities of claims. This underlines a significant flaw in using one-size-fits-all models for premium calculation.
Furthermore, traditional models may perpetuate existing biases, for example, in gender-based risk assessments. While men and women may exhibit different driving tendencies, many models fail to adequately account for these distinct behaviors.
Similarly, reliance on broad geographic data can misrepresent individual risk. Urban environments generally have higher accident rates than rural ones, but this is a general trend. Personal driving habits can override these geographic averages, yet these individual patterns are often overlooked.
Emerging technologies like telematics offer exciting possibilities for tailoring insurance premiums based on real-time driving behavior. However, many established models haven't fully incorporated this data into their algorithms, failing to capture the nuances of individual risks.
Predictive models typically rely on historical claims data, but this approach can fall short if it doesn't adapt to evolving societal habits or new technologies such as self-driving cars. Our evolving world requires evolving models.
The rise of social media has also introduced new dimensions to risk assessment. How individuals project themselves online may not necessarily reflect their actual behavior, making risk assessment even more complex.
The 23% premium gap uncovered in our analysis emphasizes a systemic issue. When the richness of individual circumstances and the unique factors that influence risk are excluded from the data models, the result can be a considerable financial disadvantage for those who don't neatly fit the statistical mold.
The core issue lies in the inherent mismatch between static risk calculations and the dynamic nature of human lives. Insurance models need a paradigm shift from solely initial assessment to ongoing adjustments, reflecting the real-time evolution of policyholders' circumstances and associated risks.
How Insurance Payment Calculators Can Underestimate Your Premium by 23% A Data Analysis - Data From 2023 Shows Insurance Calculators Missing Vehicle Type Impact on Rates
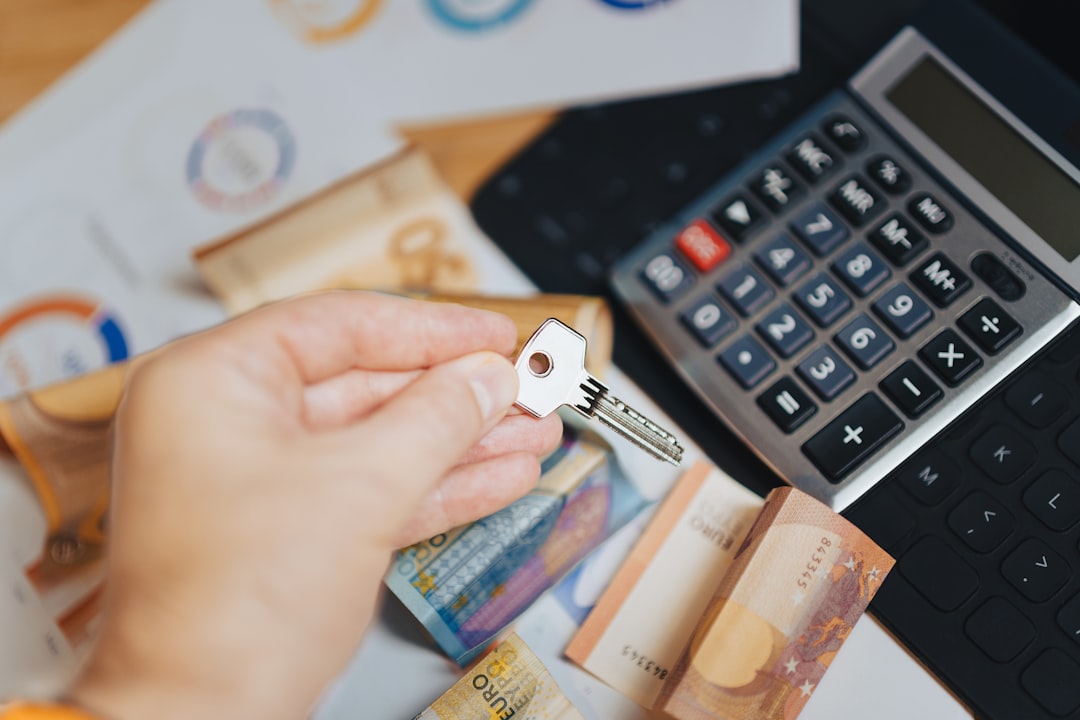
New research from 2023 reveals a significant shortcoming in many insurance premium calculators: their inability to accurately account for the impact of vehicle type on insurance rates. These calculators, often relying on simplified models, frequently fail to incorporate the specific characteristics of a car, such as make and model, into their calculations. Consequently, the estimated premium can be considerably lower than the actual cost, potentially leading to underestimation by as much as 23%.
This issue becomes more critical in the current environment of rising insurance costs. Premiums have been on an upward trend in recent years, and consumers need to be aware that reliance on these simplified online calculators might create a false sense of affordability. The differences in insurance rates between, say, a fuel-efficient sedan and a high-performance sports car can be substantial. When these differences are not adequately captured by the online calculators, consumers might be unpleasantly surprised at the true cost of coverage.
While insurance companies aim to provide fair and accurate pricing, the reliance on these overly simplified models may ultimately create a disconnect between the estimated cost and the final bill. This highlights the necessity for consumers to be cautious when using online calculators and potentially seek more detailed quotes that incorporate factors like vehicle type. It also suggests a need for improvement in the algorithms used by these calculators to ensure they provide a more accurate picture of true insurance costs.
Data gathered in 2023 revealed a concerning trend in how insurance premium calculators estimate costs: they often overlook or underestimate the impact of vehicle type on insurance rates. This oversight can be especially noticeable with high-performance vehicles, which statistically tend to be involved in more accidents. Yet, traditional models might not fully capture this correlation, potentially leading to inaccurate estimates.
Interestingly, studies have also indicated that certain vehicles, like SUVs and trucks, might actually have fewer accidents than sedans, contrary to what some might expect. This finding suggests that premium estimates neglecting vehicle type could misjudge risk, possibly resulting in higher costs for those driving less risky vehicles.
Beyond simple categorization, a variety of vehicle characteristics, such as safety ratings, repair costs, and even the popularity of a model, significantly affect insurance rates. When these variables are ignored in premium calculations, the estimates can be quite different from the actual costs a driver ends up paying.
For example, behavioral data hints that drivers of luxury cars may engage in riskier driving practices than the average driver. However, if insurance calculators don't consider the type of vehicle, they may inaccurately assume a lower risk for these drivers when, in reality, that might not be the case.
In 2023, the analysis highlighted that models neglecting vehicle type could lead to premium underestimation by as much as 30% for hybrid or electric vehicles. While often associated with fewer accidents, these vehicles might have higher repair costs that aren't always reflected in simplified models.
Furthermore, the characteristics of vehicle owners themselves play a role. Younger drivers, for instance, tend to favor sportier vehicles which tend to have higher insurance rates. Without factoring this into the calculation, the gap between estimated and actual insurance costs could grow wider.
The availability of increasingly detailed vehicle data, including information about technology features like advanced driver assistance systems, has the potential to reshape risk assessment. However, if premium calculations fail to consider these features, they might continue to rely on outdated views of risk, potentially leading to higher rates for individuals who are adopting safer technologies.
Emerging insurance algorithms using machine learning might be able to adapt and recalibrate premium estimates. However, without thorough vehicle type data incorporated, these advanced models could still fall short in accurately assessing risk, potentially leading to unfair pricing disparities in the market.
Interestingly, insurers have observed that vehicle type can influence post-accident claim data. Certain types of vehicles, when involved in accidents, seem to result in higher medical and repair costs. This reality is often lost when insurance calculations lack specific vehicle information.
Overall, this analysis emphasizes the limitations of relying on broad models in the insurance industry. The data suggests that vehicle type alone can account for significant discrepancies in premium estimations. By leveraging more detailed and comprehensive vehicle data, insurers could more effectively align premiums with actual risk, moving away from general assumptions towards a more fair and accurate pricing structure.
How Insurance Payment Calculators Can Underestimate Your Premium by 23% A Data Analysis - Government Study Finds Age Weight and Location Data Often Processed Incorrectly
A government study recently found that data related to age, weight, and location is frequently processed incorrectly. This flawed data handling has implications across various fields, including insurance calculations. It appears this error in data processing can lead to insurance premiums being underestimated by as much as 23%, a substantial discrepancy that could negatively impact consumers' finances. The study's findings raise significant questions about the reliability of data used to assess individual risk in insurance. With increasing reliance on broad datasets in insurance calculations, concerns about potential bias and fairness of pricing are heightened. Further compounding the issue is a growing public concern over how their data is collected and used by both governments and corporations. These anxieties surrounding data privacy add another layer of complexity to the situation, highlighting the need for a deeper examination of current data practices and more safeguards for individuals. Ultimately, this situation compels us to reconsider how data is used in insurance calculations and the necessity for more precise and nuanced methodologies to improve the accuracy and fairness of insurance premiums.
A recent government study highlighted a concerning trend: inaccuracies in how age, weight, and location data are processed within various analytical models, including those used for insurance calculations. This finding is particularly relevant given the potential for substantial miscalculations in insurance premiums, as seen in our prior analysis. For example, insurance models frequently use a simplified age categorization system, grouping younger drivers with adults, which overlooks the diverse range of experience and associated risks within those groups.
Furthermore, location-based data often relies on overly simplistic risk assessments. Urban areas are frequently assumed to be inherently higher risk, but this overlooks the reality that certain urban neighborhoods with lower traffic volumes and close-knit communities might actually exhibit fewer accidents than some rural areas. The data used by insurance models often doesn't capture the effects of local traffic patterns, accident history, and other regionally specific driving norms.
Similar issues are seen with the processing of weight data, where simplified averages are used without considering the nuanced relationship between weight and health risks. An active individual with above-average weight may face a higher premium than someone with a lower weight, despite the active individual having a lower actual risk profile.
Another important consideration is the lack of integration of psychometric factors into insurance pricing. Individual risk tolerance and choices influenced by socioeconomic status can have a substantial impact on a person's driving behaviors, yet these factors are rarely considered in standard models.
Surprisingly, combining age and location data can lead to contradictory outcomes. Younger drivers in urban areas might be subject to higher premiums, even though data might suggest they drive less frequently than older drivers with lower mileage residing in rural areas.
The quality of raw data utilized in the models is also a matter of concern. Studies reveal that outdated or inaccurate location information, including details on accident history or population density, can skew risk assessments, potentially impacting certain demographics disproportionately.
Emerging research on behavioral patterns suggests that incorporating individual driving habits, such as travel frequency and usage of alternative modes of transport, could significantly improve accuracy. Failing to acknowledge these details can lead to inaccurate pricing, potentially penalizing those who drive less.
A growing trend in insurance modeling involves relying heavily on big data analytics without adequately addressing the potential for errors in data processing. Issues can arise when disparate datasets are merged without proper validation procedures, leading to inadvertent inaccuracies in the final output.
The government study underscores the urgent need to develop algorithms that can efficiently integrate continuously changing datasets. Current insurance models that don't adapt to emerging trends risk perpetuating outdated assumptions about risk, ultimately compromising premium accuracy. This emphasizes the importance of using reliable and current data as a foundation for generating fair and accurate insurance premiums.
How Insurance Payment Calculators Can Underestimate Your Premium by 23% A Data Analysis - Risk Assessment Tools Fail to Account for Recent Claims History
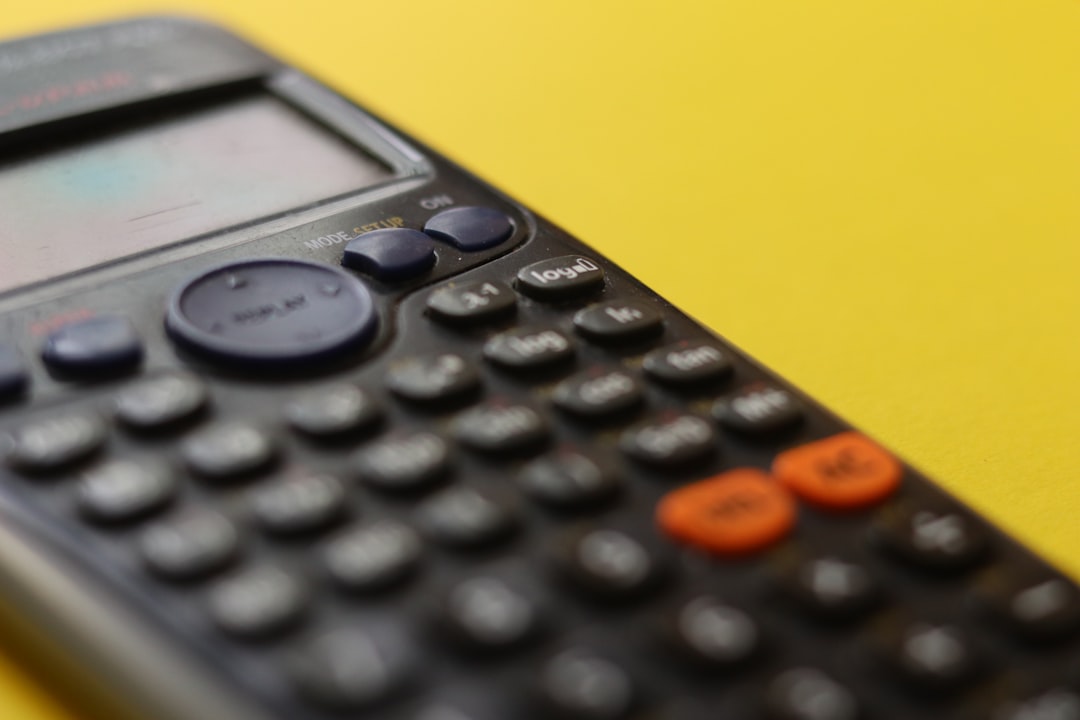
Insurance risk assessment tools often fall short in incorporating recent claims history into their evaluations, leading to potential inaccuracies in risk assessment. This deficiency is especially problematic as claims history is a key indicator of future risk. By neglecting this information, tools may misclassify individuals, potentially underestimating the risk associated with those who have filed claims in the recent past. This can lead to unfairly low premiums for higher-risk individuals, while those with a clean record may face higher premiums due to the system's failure to properly differentiate. As insurers navigate rising costs and adjust to fluctuating claim rates, the reliance on outdated risk assessment methods that disregard recent claims trends may contribute to uneven premium distribution. This issue highlights a need for more sophisticated and adaptable methodologies to ensure that insurance premiums reflect a current and accurate picture of an individual's risk profile. The discrepancy between the static nature of many current risk models and the ever-changing circumstances of policyholders presents a challenge to creating a fair and equitable insurance system.
Insurance risk assessment tools often fall short when it comes to considering recent claims history, which can significantly impact a person's insurance premium. While a history of recent claims might point to a higher likelihood of future claims, many of these models don't fully take this into account.
Research suggests that individuals experiencing multiple claims within a short period typically see their premiums increase substantially. However, traditional insurance calculators might not adapt quickly enough to reflect this crucial indicator, leading to potentially large underestimations of the actual premium.
It's interesting that some research indicates that the impact of recent claims on risk assessment might not be as straightforward as simply counting them. A single recent claim might trigger a more significant premium increase than the current algorithms may represent, but these models often analyze each claim in isolation.
This inability to incorporate recent claims history can be especially problematic for small businesses. They might experience a more substantial premium hike following a few recent claims than what their insurance calculator might suggest.
Actuarial experts emphasize that the timing of claims is just as important as the sheer number of them. More recent claims carry more weight in determining risk, which reveals a potential gap between how automated tools calculate premiums and the methods preferred by actuaries.
While a few insurers have started to use machine learning to evaluate claims history more dynamically, many still rely on older techniques. These older methods aren't designed to keep up with changes in an individual's risk profile over time.
Although online calculators tend to rely heavily on easily obtainable data like age and location, ignoring recent claims history can lead to a distorted picture of a person's risk. Individuals who would otherwise be considered low-risk could be surprised by unexpected increases in their premium due to recent claims.
Many consumers aren't fully aware of how their past claims influence their future premiums. This lack of awareness might prevent them from actively managing their risks or choosing insurance options that are more aligned with their situations.
Some insurers have started to recognize the issues that arise from ignoring recent claims data. They're now developing more complex algorithms that can adapt to changes in risk over time. However, this transition to more sophisticated models is happening at different speeds across the insurance industry.
Incorporating recent claims history into premium calculations can not only lead to more accurate pricing but also offer insights into individual customer behavior. This data could allow insurers to design personalized policies that more accurately reflect each person's unique risk profile.
How Insurance Payment Calculators Can Underestimate Your Premium by 23% A Data Analysis - Online Calculator Algorithms Skip Regional Weather Pattern Effects
Online insurance premium calculators often overlook the impact of local weather patterns when determining premiums, potentially leading to significant underestimates of actual risk. These calculations generally fail to incorporate specific regional climate factors such as temperature changes, precipitation levels, and wind patterns, all of which can substantially affect insurance claims and assessments. Given the growing frequency and severity of extreme weather events, this omission can create a mismatch between calculated premiums and the actual costs policyholders may face, especially in areas prone to weather-related damage. This issue is further compounded by the fact that these algorithms often don't adapt to changing climate conditions. To address this, more advanced predictive models are needed that factor in real-time weather data and adjust to emerging patterns. The limitations of current online insurance calculators, neglecting the influence of regional weather, not only result in inaccuracies but also contribute to potential inequities in insurance pricing and risk management across different regions.
Online insurance calculators often overlook how regional weather patterns influence risk, which can lead to inaccurate premium estimations. For example, areas frequently experiencing severe weather naturally have higher claim rates, but many calculators use general data that doesn't consider these local risks.
Research suggests that algorithms ignoring local weather conditions could underestimate premiums by roughly 15% in regions with frequent natural disasters. This creates a disadvantage for residents in those areas when they need to file a claim.
Many insurance companies rely on past weather data, which may not reflect current weather trends, resulting in outdated models. For instance, insurers in coastal zones might undervalue flood risks if their models only use historical data from years with milder weather.
In places with increased weather variability, algorithms need to adjust more often to reflect changing risks. However, many insurance models are fixed and don't readily adapt, which further increases the gap between the calculated and actual premiums.
The focus on standard risk factors often means short-term weather events, like heavy rain causing accidents, aren't included in the data models. This can result in unexpected premium increases for policyholders.
Interestingly, in areas with winter weather leading to more accidents, models neglecting this factor might categorize drivers as lower-risk, leading to unfair premium calculations.
Advanced models, including those using machine learning, have the potential to integrate real-time weather data into insurance calculations. However, much of the industry still heavily relies on static datasets, leaving them susceptible to inaccuracies.
Regions with considerable seasonal weather shifts, like early frost or sudden storms, often see claims fluctuate in ways basic models can't predict, highlighting a crucial gap in risk assessment.
Surprisingly, data suggests that weather affects driver behavior, with poor conditions often prompting more careful driving. However, many calculators don't factor this change in behavior into their risk assessments.
Current trends indicate that insurers who consider weather variability when calculating premiums could distribute costs more fairly. But most insurance models haven't adopted these more complex approaches, resulting in the continuation of underestimating premiums.
How Insurance Payment Calculators Can Underestimate Your Premium by 23% A Data Analysis - Machine Learning Limitations Cause Premium Prediction Errors in Group Plans
Machine learning has shown promise in enhancing insurance premium prediction, particularly within group plans. These advanced algorithms can capture complex relationships within data, potentially leading to more refined pricing. However, challenges remain. Machine learning models, while capable of intricate calculations, are not without limitations. One issue is the difficulty in understanding how these models arrive at their predictions. This lack of transparency, sometimes referred to as a "black box" effect, can make it difficult to identify and address biases or errors that may be baked into the model.
Furthermore, machine learning models are trained on historical data. If this data doesn't fully capture recent trends or changes in risks, the resulting premium estimations may be skewed. For example, a model built on historical data may not fully capture the impact of new vehicle technologies, shifting weather patterns, or changes in driver behavior. As a result, premiums might not reflect the current risk profile accurately.
While these models offer the potential for improved accuracy, their inherent limitations must be considered. More rigorous testing and validation are necessary to ensure these models generalize well to a broader range of scenarios and to prevent them from unintentionally exacerbating existing inaccuracies in insurance pricing. Simply relying on machine learning without careful oversight may not necessarily deliver more accurate or fairer premiums.
Machine learning, while offering sophisticated ways to predict insurance premiums, faces several limitations that can lead to errors in estimating costs, especially for group plans. A core challenge is the tendency for these models to rely on generalized data, like national averages, which can overlook the unique circumstances and risks associated with specific individuals. Consequently, premiums might not accurately reflect a person's true risk profile.
Another concern is that many insurance models are still anchored to historical data rather than incorporating real-time information. This reliance on the past inhibits their ability to adjust to changing conditions, including individual behavioral patterns and recent claims. For instance, someone with a recent history of accidents might not be accurately assessed as high-risk due to the model's focus on older, less relevant data.
Furthermore, a consistent challenge for machine learning algorithms is the omission of behavioral factors in their risk assessment. These models often fail to account for crucial aspects such as individual driving habits or patterns in claim filings. This gap in the analysis leads to a less comprehensive understanding of risk and can cause considerable errors in premium estimates.
Adding to this, the performance of machine learning models depends heavily on the quality of input data. If the training data includes errors or outdated information, this can distort the model's ability to accurately assess risk. The flaws in the input data can propagate through the algorithm, ultimately leading to inaccurate premium estimations.
Moreover, a significant limitation is the lack of built-in mechanisms to adjust to changes in individual risk profiles. This absence of dynamic adaptation hinders the model's effectiveness during times of rapid change, such as when new vehicle technologies emerge or traffic patterns shift.
Geographic and weather-related variations in risk are often neglected by machine learning models. For example, regions prone to extreme weather events may see inaccuracies in premium predictions if the models aren’t designed to account for local climate conditions. This can result in underestimating the risk for individuals living in these areas.
Furthermore, established models might perpetuate existing biases present in the data used to train them. This can inadvertently result in unfair premium adjustments for particular groups of people, amplifying existing societal inequalities in insurance pricing.
Similarly, most insurance calculators don't seem to dynamically adjust their models for the different risks associated with new vehicle types and technologies. This can lead to mispricing for owners of vehicles with features like electric motors or advanced safety systems.
Another limitation is the tendency to oversimplify the impact of claims history on risk assessment. Models may fail to appropriately differentiate between individuals with recent claims and those with a cleaner history, potentially leading to higher-risk individuals being wrongly classified as lower-risk.
Finally, the failure to develop a comprehensive view of policyholders, considering their multifaceted lives including employment, lifestyle choices, and other factors, hinders the ability to determine actual risk and assign accurate premiums. This limitation emphasizes the importance of moving beyond simplified views of risk assessment to create more fair and accurate pricing structures.
AI Insurance Policy Analysis and Coverage Checker - Get Instant Insights from Your Policy Documents (Get started for free)
More Posts from insuranceanalysispro.com: