AI Insurance Policy Analysis and Coverage Checker - Get Instant Insights from Your Policy Documents (Get started for free)
Fleet Insurance Trends How AI is Reshaping Risk Assessment in 2024
Fleet Insurance Trends How AI is Reshaping Risk Assessment in 2024 - AI-Powered Real-Time Data Analysis Reshapes Underwriting
The way fleet insurance underwriters assess risk is undergoing a major change due to AI. Gone are the days of solely relying on manual data checks and human intuition. AI is now able to quickly analyze a vast amount of information from different sources, allowing for a much more nuanced and comprehensive view of risk. This means that underwriting decisions can be made faster and with a higher degree of accuracy than before.
The use of AI also opens the door to more flexible pricing models. Instead of fixed premiums, insurers can adjust prices in real-time based on the constantly changing risk factors that AI identifies. This could benefit both the insurers and those purchasing insurance. The future of underwriting in this sector is likely to involve an even greater reliance on real-time data, especially as AI integrates with newer technologies like blockchain and the Internet of Things. This will pave the way for a new generation of insurance products, customized and priced to meet the specific needs of fleet operators in a more refined way than ever before.
Artificial intelligence is fundamentally altering how insurance underwriters evaluate risk, particularly in the dynamic realm of fleet insurance. AI's ability to sift through massive datasets in real-time is enabling more precise and adaptable risk assessments. While traditional methods relied heavily on past data, AI can incorporate a wide array of dynamic factors, including real-time traffic conditions or weather forecasts, that can swiftly influence a fleet's risk profile.
Furthermore, AI extends beyond structured data. Natural language processing allows algorithms to extract valuable insights from unstructured sources like social media or customer reviews, providing a window into fleet performance and driver conduct that might be missed by traditional methods. These insights add another dimension to risk analysis.
Machine learning is proving especially potent. AI models can continuously refine their understanding of risk by learning from new data, recognizing trends in accidents and severity that might not be apparent in historical averages. This adaptability is crucial in a landscape where risk profiles are constantly evolving.
Beyond better insights, AI helps improve fraud detection in real-time, analyzing claims data for unusual patterns. This fosters a more honest operating environment for fleet operators. The ability to integrate telematics data, offering a granular view of individual driving behaviors, allows for more personalized underwriting, potentially rewarding safer drivers with lower premiums.
Looking ahead, AI-powered tools can even anticipate potential mechanical issues based on maintenance data, moving fleet risk management from a reactive to a proactive stance. This capability, coupled with the ability to model different risk scenarios, allows insurers to better understand and prepare for changes in regulatory environments or shifts in driver demographics. By analyzing driver behavior, AI can even pinpoint specific actions linked to accident likelihood, providing a more nuanced understanding of individual and fleet risk.
The overall impact of AI is not only enhanced risk assessment, but also streamlined operational efficiency. AI-powered automation can expedite processes, from initial assessments to claims handling, leading to faster turnaround times and improved customer experience. In essence, AI is redefining the underwriting landscape, transitioning from reliance on historical data to a dynamic, forward-looking approach to risk management, benefiting both insurers and those they insure.
Fleet Insurance Trends How AI is Reshaping Risk Assessment in 2024 - Machine Learning Algorithms Enhance Predictive Analytics for Risk
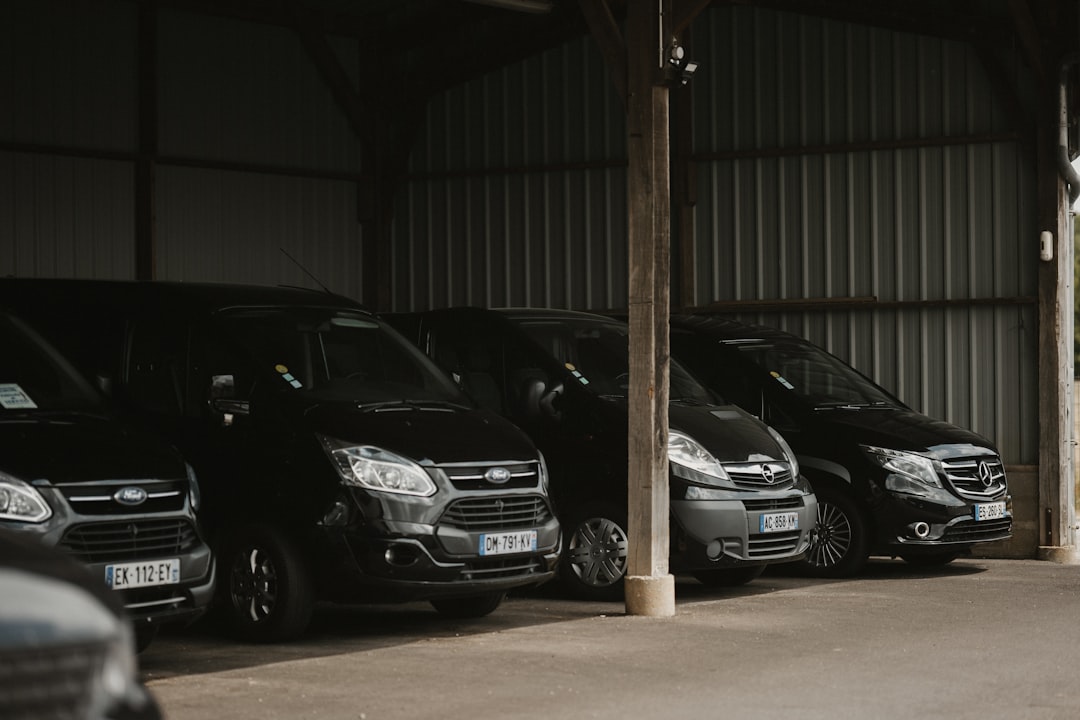
Machine learning algorithms are transforming how fleet insurance assesses risk, moving beyond traditional methods that relied on historical data. These algorithms can now analyze massive datasets in real-time, considering a much wider range of factors. This includes things like driver behavior, current traffic conditions, and even potential mechanical issues. This results in a more nuanced understanding of risk, leading to more accurate underwriting decisions.
Furthermore, machine learning enhances fraud detection by recognizing unusual patterns in claims data, potentially helping to create a fairer playing field. The ability to process and analyze information more quickly with machine learning also brings benefits in operational efficiency. Insurers are seeing faster turnaround times, from initial assessment to claims handling. This ultimately improves the overall customer experience.
Looking ahead to 2024, the continued growth of AI and its applications in fleet insurance suggests an even more profound impact on risk assessment and operational strategies. The industry is still navigating how these new capabilities will reshape the risk landscape, but early signs indicate a shift towards more predictive, adaptable, and precise risk management.
Machine learning algorithms are proving remarkably adept at evaluating risk in fleet insurance by considering numerous factors simultaneously. They can analyze over a hundred variables at once, uncovering complex relationships that traditional methods often miss. This intricate analysis extends beyond the usual historical data, especially with the rise of connected vehicles. A large chunk of predictive analysis now hinges on real-time data, resulting in significantly more accurate risk assessments compared to solely relying on static historical information.
The impact of these advancements extends to operational efficiency, with research indicating machine learning can significantly cut claim processing times, perhaps by as much as 70%. These algorithms automatically flag anomalies and prioritize cases needing prompt attention, streamlining workflows. Furthermore, algorithms are increasingly able to anticipate maintenance issues by recognizing patterns within telematics data. This potentially minimizes unexpected vehicle breakdowns and their associated risks, transforming fleet risk management from reactive to proactive.
Machine learning's continuous learning ability further enhances its capabilities. The predictive models are continuously refined as new data is collected and analyzed, leading to increasingly precise risk assessments over time. This continuous improvement has been shown to be particularly effective in fraud detection, with some algorithms boasting impressive 90% detection rates through intricate pattern recognition. This surpasses traditional methods by a huge margin.
The realm of unstructured data is no longer out of reach for insurers. The use of natural language processing allows algorithms to extract valuable insights from customer feedback and social media posts, offering a window into driver behavior that was previously inaccessible. These insights are vital for a complete understanding of fleet risk.
These technological advancements aren't just about improving risk assessment. The ability to accurately assess individual fleet risk rather than applying a standard premium structure can lead to premium reductions of 10-20% for fleet operators using machine learning predictive analytics. The benefits extend beyond the insured, as insurers utilizing these technologies see about a 15% increase in customer acquisition compared to their competitors who haven't embraced predictive analytics.
The flexibility of these machine learning algorithms allows insurers to engage in a forward-thinking approach to underwriting. Algorithms can create various "what-if" scenarios, simulating the influence of changes in driving patterns, weather, or traffic on risk. This proactive approach gives insurers a distinct advantage in a constantly changing environment. Insurers are able to prepare and adapt to dynamic situations, highlighting the powerful potential of these tools. Overall, these advancements in machine learning are poised to transform the landscape of fleet insurance in significant and lasting ways.
Fleet Insurance Trends How AI is Reshaping Risk Assessment in 2024 - Telematics and IoT Integration Revolutionize Fleet Monitoring
The convergence of telematics and the Internet of Things (IoT) is revolutionizing how fleets are monitored. By harnessing the power of connected devices, we now have access to a wealth of near real-time data about vehicle operations. This data can be used to improve various aspects of fleet management, from scheduling preventative maintenance to optimizing fuel consumption and driver behavior.
The integration of these technologies is particularly beneficial for enhancing safety. Telematics data can identify potential hazards and even predict accident likelihood, giving fleet operators the opportunity to proactively mitigate risks. The ability to track and analyze driver performance in detail can also promote safer driving habits and potentially reduce accidents.
Looking ahead, the incorporation of artificial intelligence (AI) within these systems is poised to further transform fleet management. AI's capacity to analyze vast amounts of data can lead to more efficient operations and more sophisticated risk assessment. This may eventually lead to a future where fleet insurance is more personalized and responsive to individual risk profiles, rather than relying on broad classifications.
However, it's important to consider the potential drawbacks of this technological shift. As we increasingly rely on data collected by connected devices, questions regarding data privacy and security become crucial. Furthermore, the accuracy and reliability of telematics data in informing critical decisions like insurance premiums warrants ongoing scrutiny. As the industry navigates this transition, striking a balance between innovation and responsible data management will be essential.
The convergence of telematics and the Internet of Things (IoT) is dramatically reshaping how we monitor and manage fleets. Connected vehicles are generating a constant stream of data, giving fleet managers a level of insight previously unimaginable. Think of a single commercial vehicle potentially generating over 25 gigabytes of data each day, detailing everything from GPS location to engine diagnostics and driver behavior. This deluge of information paints a far more comprehensive picture of both vehicle and driver performance compared to the old, more limited methods.
One of the most impactful areas is predictive maintenance. By using AI and machine learning to analyze the data patterns from telematics, fleets can potentially reduce vehicle downtime by up to 30%. Identifying potential mechanical problems before they manifest into costly breakdowns is becoming a more realistic goal. This approach to maintenance is proving quite helpful, as is the ability to potentially see insurance premium reductions of 10-15%. By basing premiums on actual driving behaviors rather than just historical trends, insurers can get a more precise picture of risk.
Improved safety is another key area. Fleets using real-time monitoring have seen accident rates decrease by as much as 40%. It seems that immediate feedback on risky driving behavior can promote safer driving practices. Also, the integration of telematics with AI allows insurers to create more sophisticated risk models that include factors such as environmental conditions, traffic, and historical driver behavior to potentially forecast accidents.
Beyond vehicle location tracking, telematics data allows for more advanced behavioral analytics. Algorithms are being developed that can infer driver emotions and behaviors from data, which could potentially shed more light on how things like stress, fatigue, or distractions influence performance behind the wheel. This is an area of active research that might lead to a better understanding of how humans interact with complex machinery.
The benefits of telematics extend to things like cost reduction. Improved route planning and reduced idle times have resulted in fuel cost savings of up to 15% in some fleets. The ability to scale telematics solutions across different size fleets, from small businesses to large corporations, is also important. It's interesting to note that these methods aren't limited to just transportation. Industries like construction and agriculture are starting to adopt similar approaches to enhance safety and productivity.
The integration of telematics and the IoT is still relatively new, and there's a lot we still don't know about its long-term impact. It's exciting to consider the possibilities as these technologies continue to develop and become more integrated into our daily lives. This is an area where researchers and engineers can make a significant contribution, developing innovative ways to leverage the incredible data these systems are generating. The potential for improvements in safety, efficiency, and cost reduction is very promising, and it's only the beginning of this trend.
Fleet Insurance Trends How AI is Reshaping Risk Assessment in 2024 - Automated Claims Processing Accelerates Settlements
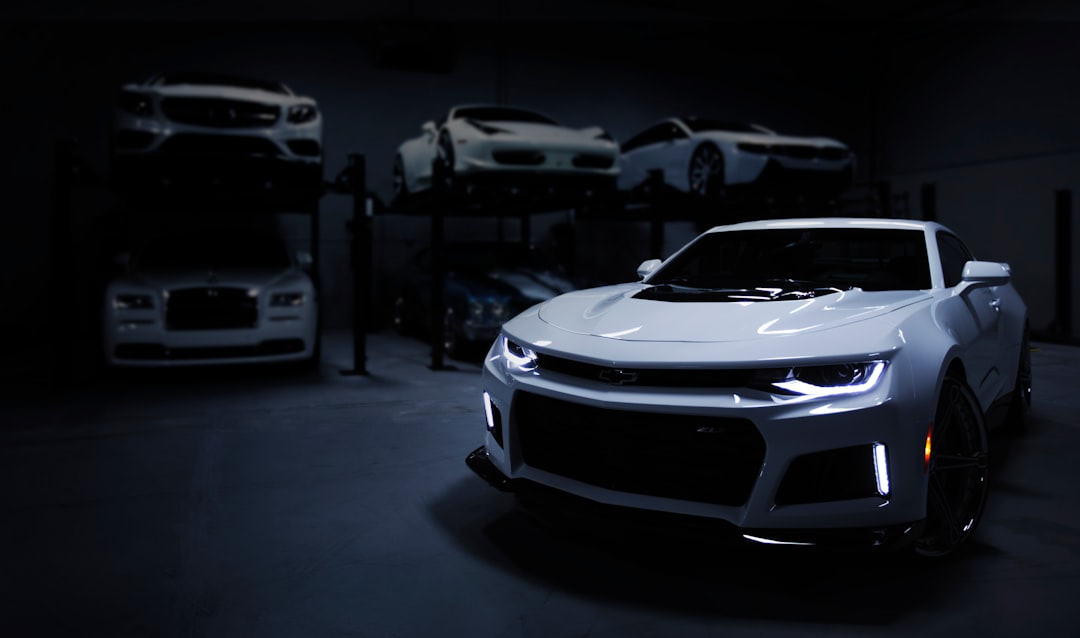
The increasing adoption of AI within fleet insurance is transforming claims processing, making it a faster and more efficient part of the process. Insurers are implementing automated systems that leverage machine learning to analyze large volumes of data, leading to a significant decrease in the time it takes to handle claims, potentially up to a 30% reduction. This speedier processing benefits policyholders with faster payouts and reduces the administrative workload for insurance companies. Beyond speed, these automated systems are enhancing accuracy and helping to minimize fraudulent claims by quickly identifying unusual patterns in the data. This ability to rapidly process and analyze vast amounts of information empowers insurers to make more informed decisions. The trend towards automated claim processing is creating a new paradigm where claims are settled quicker, more accurately, and with greater alignment to individual fleet risk profiles, improving the overall customer experience.
The insurance landscape, particularly in fleet insurance, is seeing a significant shift towards automation in claims processing, driven by the increasing adoption of AI and machine learning. This trend is fueled by a desire to accelerate claim settlements, leading to a more efficient and customer-centric experience. AI-powered systems can process massive volumes of claims data remarkably fast, allowing for much quicker assessments and resolutions compared to the traditional, multi-step, manual process.
While some might be skeptical, the results are compelling. It's estimated that automation can cut claim processing times by up to 30%, translating to faster payouts for policyholders and easing the burden on insurance staff. This reduction in processing time is primarily achieved by algorithms that can rapidly sift through vast amounts of data and identify key information.
Furthermore, AI's ability to analyze real-time data from telematics and IoT devices is a game changer. It's not just about speeding up the process, but also making it more informed. Insurers can now base decisions on actual driver behavior and vehicle conditions, moving away from relying solely on historical averages or estimations.
Of course, the use of AI also presents potential issues. One concern is the potential for algorithmic bias, which needs to be carefully addressed. However, many see its implementation as a significant improvement in fraud detection, with some estimates suggesting that these systems can achieve fraud detection rates as high as 85%. This ability to identify potential fraudulent claims early in the process benefits both insurers and honest policyholders.
Beyond the obvious speed and efficiency gains, the shift towards AI-powered claim processing systems appears to be positively impacting customer satisfaction. Policyholders, rightfully so, are usually not thrilled about filing claims. Automated systems, with their capacity to provide quicker and clearer communication, are contributing to a more streamlined and less stressful experience for policyholders.
It's still early days in the widespread use of these systems, but preliminary results are encouraging. AI's influence on claims is broadening the scope of what's possible within insurance. This includes the integration of predictive analytics, which can help anticipate future claim trends and facilitate better risk management practices. However, there are aspects worth continued investigation. For example, it will be important to examine how data privacy and security are addressed as reliance on these systems grows.
The insurance sector is starting to leverage automated claims processing capabilities in a variety of ways. They can handle larger volumes of claims without increased staff, streamlining operations and improving response times during peak periods. The systems also come equipped with robust reporting capabilities that help insurance companies track and evaluate their risk management strategies, allowing them to make data-driven adjustments to their practices. It is interesting to see how this trend impacts not just claims management, but the very nature of underwriting strategies in the future. As the industry continues to integrate AI into its processes, the focus needs to be on responsible innovation, particularly regarding data security and transparency, ensuring benefits for both insurers and policyholders.
Fleet Insurance Trends How AI is Reshaping Risk Assessment in 2024 - AI-Driven Fraud Detection Systems Improve Accuracy
AI is increasingly crucial for improving the accuracy of fraud detection within fleet insurance. These systems continuously analyze data, identifying new fraud patterns and allowing insurers to spot potentially fraudulent claims in real-time. This prompt identification accelerates investigations and streamlines the claims process. AI's flexibility surpasses traditional, rule-based methods, allowing for swifter responses to evolving fraud techniques. Proven results show AI can detect fraudulent claims with accuracy rates as high as 90%, significantly decreasing fraud and fostering a more reliable insurance environment. The expanding use of AI within fleet insurance has a major impact on risk assessment. This, in turn, leads to underwriting practices that are more informed and responsive to the unique driving behaviors and conditions of individual fleets. While some see potential biases, the benefits of faster processing and accurate fraud detection are becoming increasingly valuable.
AI is significantly enhancing the accuracy of fraud detection within the insurance sector, particularly in fleet insurance where the potential for fraudulent claims can be substantial. AI systems, unlike older rule-based systems, can scrutinize claims data incredibly fast, often within seconds, identifying irregularities that might go unnoticed by humans. This real-time analysis allows insurers to flag suspicious claims promptly, preventing them from growing into larger issues.
One of the remarkable achievements of AI in this area is its ability to detect fraud with a precision exceeding 90%. These systems achieve this level of accuracy by recognizing intricate patterns hidden within massive datasets, something that's simply beyond the capabilities of human adjusters. It's intriguing to see how these algorithms can spot complex connections between data points that humans often miss.
The incorporation of telematics data further refines fraud detection capabilities. Instead of relying solely on historical trends and driver profiles, AI can now assess risk based on a vehicle's real-time usage. This gives insurers a much more detailed and nuanced picture of driver behavior, helping to identify inconsistencies and signs of potential fraud.
The financial implications of fraud can be substantial for insurance providers. Estimates suggest that fraudulent claims can account for up to 10% of overall claim costs. Therefore, AI's role in mitigating these losses is crucial. By streamlining the fraud detection process and automating investigation, insurers can significantly reduce their exposure to fraudulent claims.
AI's ability to analyze unstructured data is also noteworthy. Natural Language Processing (NLP) allows systems to sift through customer interactions, social media posts, and other informal sources of information, uncovering hints or patterns that may indicate fraudulent activities. This ability to understand human language in context provides a broader lens through which to assess a claim's authenticity.
This heightened focus on fraud detection doesn't only accelerate claims processing but also improves the overall accuracy of assessments. Research suggests that insurance providers can potentially realize operational cost reductions of up to 20% by leveraging AI for fraud detection. It's fascinating to observe how technology is impacting the efficiency and cost structure of the insurance industry.
One of the interesting outcomes of AI-driven fraud detection is a potential deterrent effect. When claimants are aware that their claims are being analyzed in real-time, they are often less inclined to make fraudulent claims. This encourages a more trustworthy and transparent environment within the insurance system.
Beyond financial savings, the speed at which AI identifies fraud allows insurers to deploy their resources more efficiently. Rather than getting bogged down in investigating false claims, they can concentrate their efforts on addressing legitimate claims, improving the overall experience for honest policyholders.
Interestingly, the very nature of AI-driven fraud detection allows these systems to continuously learn and adapt. The algorithms utilize a feedback loop, refining their ability to identify fraud as they process more data and learn from past decisions. This continuous learning leads to improved accuracy over time, making these systems increasingly effective.
As the landscape of fleet insurance continues to evolve, the implementation of AI-driven fraud detection is becoming increasingly vital. Insurers who don't embrace these technologies risk falling behind in a rapidly changing industry. It's clear that AI fraud detection will play an increasingly crucial role in maintaining competitiveness, fostering trust among stakeholders, and ensuring the financial stability of the insurance industry as a whole.
Fleet Insurance Trends How AI is Reshaping Risk Assessment in 2024 - Personalized Premium Pricing Based on AI-Generated Risk Profiles
The adoption of personalized premium pricing based on AI-generated risk profiles represents a notable change in the way fleet insurance is handled. Insurers can now develop more precise pricing models by using AI to analyze a wide array of data points, resulting in premiums that are tailored to the unique risks of individual fleets. This offers a more accurate reflection of risk compared to traditional, broader classifications. By incentivizing safer driving through lower premiums for fleets with reduced risk profiles, insurers can also potentially encourage better driving behavior.
Despite the clear benefits of dynamic risk assessment via AI, the implications of this new approach deserve thoughtful consideration. Issues regarding the privacy and security of data used to generate these profiles need to be carefully addressed. Furthermore, the potential for algorithmic bias within the AI models raises concerns regarding fairness and equity in pricing. As this personalized approach to pricing continues to develop, striking a balance between innovation and responsible data handling will be crucial for insurers to build and maintain a sustainable, trustworthy system. This approach must strive to provide benefits to all parties involved in the insurance process.
AI is enabling a new era of personalized pricing in fleet insurance by leveraging the detailed risk profiles it generates. This shift away from traditional, static premium structures means insurers can now tailor premiums based on real-time risk factors. For instance, by integrating telematics data, insurers can dissect driver behavior with a previously impossible level of detail. This allows them to identify specific driving actions linked to accidents, which can then inform personalized premium adjustments.
The impact of this real-time monitoring is profound. AI can assess risk and adjust premiums within moments of an incident, reacting to current conditions instead of relying on historical data alone. This dynamic approach to pricing can lead to significant cost reductions for fleet operators who prioritize safe driving practices. AI algorithms can streamline the entire process, potentially reducing underwriting and claims management costs by as much as 20%, which can translate to lower premiums that are far more aligned with the individual fleet's unique risk profile.
This shift also impacts the proactive management of fleets. AI-driven models can analyze maintenance data and predict potential mechanical failures, enabling fleets to address issues before they cause breakdowns. This shift towards proactive risk management can minimize unexpected problems and their associated costs, potentially leading to further insurance premium reductions.
Beyond cost savings and operational efficiencies, AI helps achieve remarkably high levels of fraud detection, often exceeding 90% accuracy. By identifying fraudulent claims promptly, insurers can not only reduce financial losses but also encourage a more equitable pricing structure, weeding out those with consistently high-risk behavior. Furthermore, tools like natural language processing allow insurers to tap into previously inaccessible data sources, like online reviews or social media. This can reveal insights into driver behavior and overall fleet reputation, informing a more comprehensive understanding of risk.
However, this new level of customization presents certain challenges. Insurers are now in a position to influence individual driver behavior through differentiated pricing – incentivizing safer driving practices while discouraging risky maneuvers. It's a fascinating area from a behavioral economics perspective. At the same time, using sophisticated risk profiles to determine premium costs raises important ethical questions. There are concerns around data privacy and the potential for algorithmic bias to lead to unfair pricing outcomes. It's crucial to consider these legal and ethical implications while evaluating the effectiveness of AI-driven pricing models in fleet insurance. AI models continually adapt and refine their understanding of risk based on newly acquired data. This ensures that pricing strategies remain fair and relevant as fleet profiles evolve over time, keeping pace with the dynamism of this sector.
AI Insurance Policy Analysis and Coverage Checker - Get Instant Insights from Your Policy Documents (Get started for free)
More Posts from insuranceanalysispro.com: