AI-Driven Underwriting Models Show 28% Accuracy Improvement in Q3 2024, Study Reveals
AI-Driven Underwriting Models Show 28% Accuracy Improvement in Q3 2024, Study Reveals - Machine Learning Model Processes 4 Million Insurance Claims For Q3 Study By Morgan Stanley
A Morgan Stanley study centered on the third quarter of 2024 revealed a machine learning model processed a vast number of insurance claims—4 million to be precise. This large-scale use of machine learning is a clear signal of how insurance companies are embracing artificial intelligence. The study itself is notable for showing a 28% boost in accuracy for AI-driven underwriting during this period. This improvement, likely due to automation replacing many of the traditional, manual processes, hints at a future where claims and underwriting happen almost instantly. The study also implies a shake-up within the insurance industry, with the potential for many existing insurers to become obsolete within the next ten years due to these rapidly advancing technologies. We're clearly seeing a transition in insurance towards more sophisticated applications of machine learning and AI—beyond simple automation and into areas that can profoundly impact how insurance is both processed and delivered.
Researchers at Morgan Stanley delved into the use of machine learning for insurance claim processing, analyzing a massive 4 million claims dataset from the third quarter of 2024. This large-scale study provides valuable insight into how these models handle real-world, high-volume scenarios. The ability to process such a large dataset efficiently speaks to the advancements in algorithm design and computational resources available today.
It's interesting to consider how the model's processing of this huge volume of claims could impact the insurance industry. We're seeing machine learning models go beyond just simple automation to tackle complex problems, including potentially uncovering previously missed patterns in claims. Imagine, for example, how better fraud detection could be achieved if a machine learning model can identify subtle patterns in claim submissions that a human analyst may miss. This could significantly alter the way insurers approach fraud prevention, potentially leading to fewer payouts for illegitimate claims.
This study's focus on machine learning suggests a shift in how insurers view data. Using advanced techniques like regression analysis and decision trees, the model potentially builds a more detailed and insightful understanding of claim outcomes, compared to traditional methods. While promising, relying solely on past data raises a crucial question – how do we ensure fairness in an environment where models can develop their own biases? For example, if historical data shows that certain demographics tend to file more claims, the model might wrongly predict future claim frequency for those groups. This is something that needs to be carefully considered, as bias can lead to unjust outcomes for certain policyholders.
The study also underlines the importance of good data. The success of the model appears to hinge not only on the powerful algorithms themselves, but also on the quality and consistency of the claims data used for training and prediction. High-quality data, particularly with fine-grained details, seems vital to the success of these approaches.
Moving forward, it is worth thinking about whether the successful use of these models will drive greater standardization across insurance claim processes. Could we see a convergence toward a more uniform way of handling insurance claims driven by the successful implementation of these machine learning models? This could lead to both efficiencies and new challenges as insurers potentially navigate the complexities of maintaining these standardized data pipelines. While the Morgan Stanley study has shown the potential benefits of machine learning for insurance, we need to thoughtfully examine the ethical and societal implications of using such powerful tools. Only with careful consideration can we ensure these advanced models are used to benefit all stakeholders, both insurers and policyholders alike.
AI-Driven Underwriting Models Show 28% Accuracy Improvement in Q3 2024, Study Reveals - Traditional Risk Models Fall Behind With 18% Lower Accuracy Rate Than AI Systems
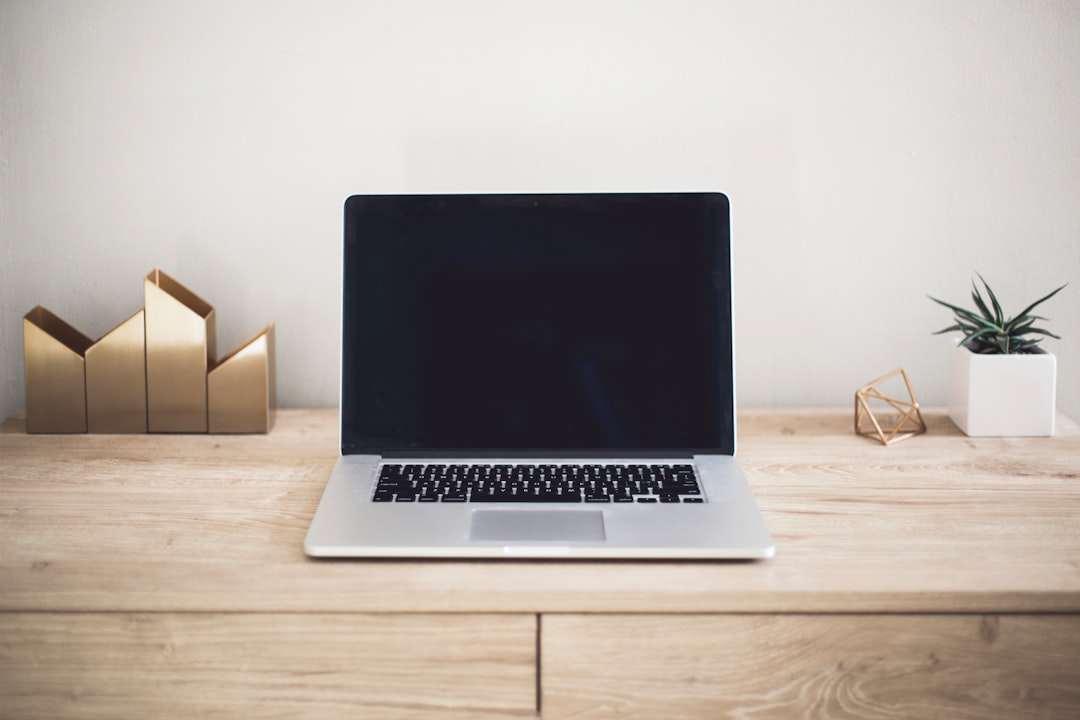
Traditional risk models are falling behind in their ability to accurately assess risk, with studies showing they produce results that are 18% less accurate than AI-based systems. This performance gap seems to stem from the challenges traditional models face when dealing with the complex, interwoven relationships found within large datasets. AI, however, appears to be adept at recognizing intricate patterns hidden within this data, leading to significantly more accurate risk predictions. The demand for more sophisticated risk management tools is driving a clear shift towards AI integration, as organizations recognize the limitations of traditional methods in today's complex environment. The growing disparity in accuracy between AI and traditional models raises important questions about whether traditional risk models can continue to effectively meet the needs of insurers and other industries in the years ahead. It seems a paradigm shift in risk analysis is underway, with AI poised to take a central role.
It's fascinating to see how traditional risk models are falling behind in accuracy compared to their AI counterparts. A recent study revealed that traditional methods lag by a significant 18% in accuracy when predicting risk compared to AI systems. This stark difference highlights the potential impact AI could have on underwriting and decision-making within insurance. It seems like the sheer volume of data that AI can process is key—the study's 4 million claim dataset is a good example. Processing such a large dataset allowed the AI model to unearth intricate patterns that likely wouldn't have been evident with traditional methods.
This potential for identifying complex patterns raises the question of whether AI can revolutionize fraud detection. By analyzing intricate claim details and historical trends at a level of granularity humans struggle with, AI may be able to detect and flag fraudulent activity with much greater precision than currently possible. In contrast, traditional risk models often rely on simpler, rule-based approaches, which could leave them vulnerable to sophisticated fraud schemes.
What's interesting is how these AI systems are built. Unlike their traditional counterparts, AI models can utilize methods like deep learning, which allow for a more nuanced and multi-layered understanding of data. This capability to capture multiple levels of complexity within data, and not just surface-level factors, potentially leads to more accurate risk assessments.
However, as with any powerful technology, ethical questions arise. One concern is the possibility of bias creeping into AI models. If the training data used to build these models reflects past prejudices, it could inadvertently lead to unfair outcomes. For example, if historical data shows certain demographics file more claims, the AI might unfairly penalize them with higher premiums, highlighting the crucial need to scrutinize these models for biases and mitigate their impact.
It’s also worth thinking about how AI is shifting the practical reality of insurance. The study from Q3 of 2024 demonstrated that AI models aren't just theoretical tools, but they can be successfully implemented in real-world operational settings. This suggests that the future of claims assessment could drastically change, moving towards instant, AI-driven decision making.
Looking further ahead, this growing dominance of AI in underwriting accuracy could trigger a shakeup in the insurance industry. Traditional companies that can't adapt quickly to these new technologies might find themselves at a competitive disadvantage, possibly leading to market consolidation or even obsolescence for some. This is a powerful incentive to encourage change within the sector.
The push towards more sophisticated AI models could also result in a drive towards standardizing claims processes. This standardization could bring increased efficiency but also carries the risk of losing some unique business practices that differentiate insurance companies. We need to consider the potential downsides of such standardization as the industry transitions to new, AI-driven workflows.
Finally, all of this hinges upon computational power. AI's capabilities are greatly enhanced by modern technology and our increasing ability to rapidly process large datasets. Traditional models simply don’t have the same potential to cope with the sheer volume of data that AI systems can handle.
The direction of change is clear—the insurance landscape will likely be profoundly different within the next 10 years. This transition will necessitate changes to company strategies—potentially shifting how insurers handle staffing, internal processes, and overall technology adoption. We are entering a new era where AI is rapidly changing the core of this industry.
AI-Driven Underwriting Models Show 28% Accuracy Improvement in Q3 2024, Study Reveals - Small Claims Processing Time Drops From 48 Hours to 14 Minutes Through Automated Assessment
Insurance companies are now processing small claims significantly faster, with the time taken shrinking from 48 hours to just 14 minutes. This remarkable improvement is due to the implementation of automated assessment systems. These systems streamline the process, allowing for faster evaluation and resolution of simple claims. We are also seeing more insurers embrace generative AI for handling claim assessments. This not only speeds up the process but also improves the accuracy of damage estimations, leading to fewer delays and fewer errors.
Freeing up claim handlers from handling basic claims allows them to dedicate their time to more complex issues. This change has the potential to boost productivity and profitability for insurers. However, this increased reliance on automation requires careful consideration. We need to ensure that this drive for efficiency doesn't come at the cost of fairness and accuracy, as the inherent risks associated with AI-powered systems require ongoing monitoring.
The shift from a 48-hour small claims processing window to a mere 14 minutes through automated assessment is quite remarkable. It's a strong indicator of how AI-driven automation can revolutionize customer experience and operational efficiency within insurance. While exciting, it also prompts questions about scalability. Can these systems handle a dramatic increase in claim volume without encountering bottlenecks?
One interesting aspect is the potential for increased consistency in claim decisions. With automated assessments, the possibility of human error or bias in evaluations is reduced, hopefully leading to fairer outcomes. This also presents a potential benefit in fraud detection. The speed of the 14-minute process, along with the ability to analyze large datasets in real-time, could enhance the detection of unusual patterns that might signal fraudulent activity.
However, it's crucial to consider the complexities of implementing these automated systems. Seamlessly integrating them with existing insurance IT infrastructures might be a considerable hurdle. It requires careful planning and execution to avoid disruption and ensure data integrity.
Beyond efficiency, the automated process can generate vast amounts of valuable data. The insights derived from these datasets could significantly improve underwriting processes and overall risk management. It's a positive feedback loop where quicker claims processing allows for better data capture, which in turn can further refine the automation algorithms over time.
Of course, faster claims payouts generally lead to increased customer satisfaction, an essential component of retention in the competitive insurance landscape. Also, the transition to AI-driven claims handling could lead to cost savings through a reduction in manual labor. But this comes with a caveat: The rapid advancement of AI necessitates that these models are consistently retrained and updated to stay ahead of emerging fraud tactics and other challenges in claim assessment.
Finally, this level of automation touches upon the need for evolving regulatory frameworks. As automation becomes more prevalent, it will be critical for regulators to establish guidelines that ensure fairness and transparency in the automated claim assessment process. This is crucial to protect consumers as these systems become more deeply embedded in the insurance industry. It's a balancing act between reaping the benefits of AI-driven automation while simultaneously ensuring consumer interests are protected.
AI-Driven Underwriting Models Show 28% Accuracy Improvement in Q3 2024, Study Reveals - Insurance Firms Save $440 Million In Operating Costs Through AI Implementation
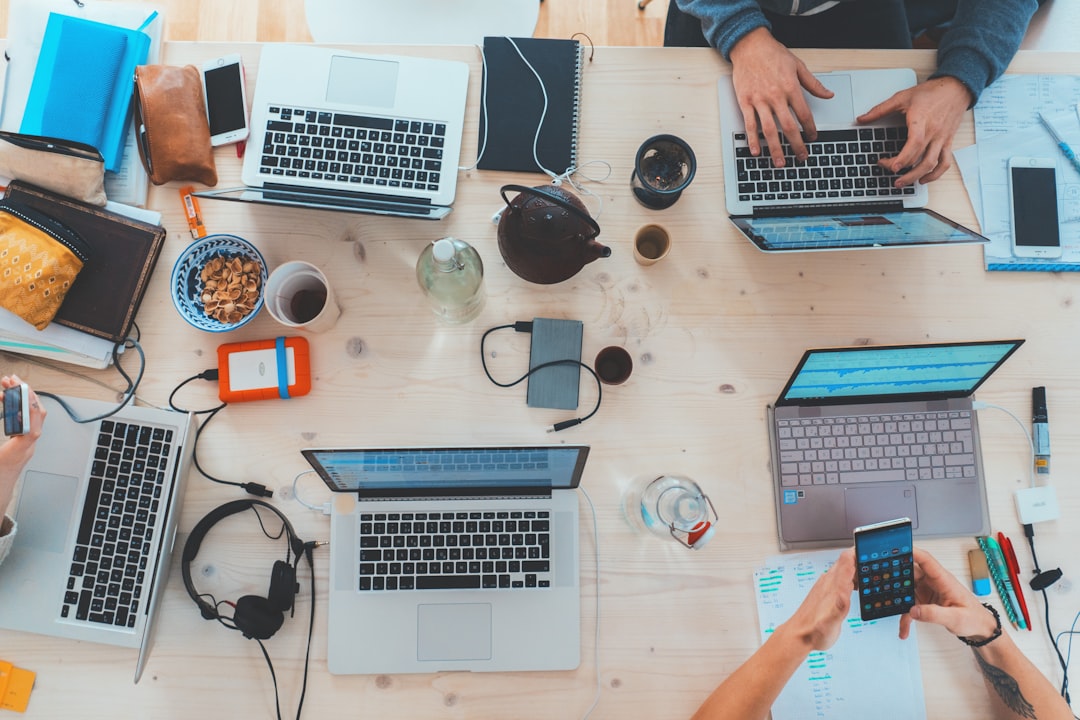
The insurance industry is experiencing a wave of cost savings thanks to artificial intelligence. Firms have successfully reduced their operating expenses by a remarkable $440 million through the implementation of AI systems. These systems are automating processes, leading to improvements in areas like underwriting and claims assessment, as seen in the 28% accuracy boost in AI-driven underwriting models. The integration of AI has made insurance operations more efficient, resulting in this substantial financial gain.
However, while the financial benefits are significant, it's important to acknowledge the potential downsides. AI-driven decision-making processes, though efficient, can introduce biases if not carefully monitored. The insurance sector must thoughtfully navigate the challenges of bias and ethical implications as it continues to embrace these powerful technologies. It's clear that AI is revolutionizing the industry, but its integration necessitates careful planning and consideration to ensure fairness and transparency for all stakeholders. The future of insurance is intertwined with the evolution of AI, offering opportunities while demanding careful management of its potential risks.
The integration of AI into insurance operations has resulted in noteworthy cost reductions, with firms reporting a combined savings of roughly $440 million in operational expenses. This figure suggests that AI's impact extends beyond just speeding up claim processing and highlights its potential to reshape the broader financial landscape of the insurance industry. It's intriguing to see how AI has allowed for quicker underwriting decisions, potentially transforming the traditional workflow towards almost instantaneous assessments. This speed has a direct impact on customer experience, with faster claim resolutions leading to higher customer satisfaction and, consequently, improved customer retention rates—a crucial aspect in today's competitive market.
One particularly interesting area where AI is showing its capabilities is fraud detection. The algorithms are able to process immense amounts of data, uncovering subtle patterns that might suggest fraudulent claims, a feat that's difficult for traditional methods to replicate. This is leading to a noticeable improvement in detecting potentially fraudulent activities, which benefits insurers by minimizing losses. However, the increasing reliance on automation does raise questions about the long-term impact on the workforce. While freeing up human employees to handle more complex claims, it also raises concerns about the potential for staff reduction.
Furthermore, AI is reshaping the risk management strategies used within the insurance sector. By analyzing massive datasets, AI models can uncover previously unseen relationships, allowing underwriters to make more informed decisions. This ability to tap into the complexity of data, particularly through predictive analytics, offers a significant advantage over traditional risk models. AI can use historical trends to develop more accurate pricing models, but it also necessitates constant model refinement and retraining to ensure accuracy in the face of ever-changing risk landscapes.
The rapid adoption of AI in the industry is naturally leading to discussions around regulation. It remains to be seen how regulators will adapt to this change. Concerns about algorithm transparency and fairness are likely to increase, and these issues could influence how AI models are designed and deployed. While AI promises significant efficiency gains, its seamless integration into older systems is challenging. Insurers face technological hurdles, such as compatibility issues and potential bottlenecks, that need careful consideration and resolution to fully realize the benefits of these advanced systems. The insurance industry's future appears to be inextricably linked to AI, bringing both opportunity and the need for thoughtful consideration of its impacts.
AI-Driven Underwriting Models Show 28% Accuracy Improvement in Q3 2024, Study Reveals - Property Insurance Claims Show Highest Accuracy Gain At 31% With New Technology
A new wave of technology has significantly improved the accuracy of property insurance claims, with a study showing a 31% accuracy increase. This surge in accuracy is largely due to the adoption of new systems, specifically leveraging artificial intelligence and automation in claims evaluation. This suggests these technologies are capable of more effectively analyzing claims and making more precise decisions. While this development is promising, it also presents the industry with opportunities and potential challenges, especially around ensuring fair and unbiased assessments. Alongside the accuracy gains, the wider integration of AI and automation has also contributed to operational improvements, such as significantly faster claim processing times and overall cost savings. However, the insurance industry must carefully consider the ethical aspects of increasing reliance on AI, especially regarding potential biases within these models. The evolution of the industry is intertwined with this rapid adoption, necessitating both careful management and proactive adaptation to these new tools.
The integration of new technologies into property insurance claims processing has yielded impressive results, with a 31% increase in accuracy reported. This improvement is particularly notable in the ability of AI systems to analyze real-time data and identify patterns within claim submissions. It seems that AI can quickly spot inconsistencies, leading to faster identification of potentially fraudulent claims, something traditional methods often struggled with.
One interesting implication of this is a potential shift in how consumers perceive insurance claims. The higher accuracy rates potentially foster greater trust by allowing for more transparent and efficient claim processing. This could, in turn, lead to a better overall experience for policyholders.
It's also intriguing that automated systems seem to not only speed up claim handling but also enhance the accuracy of damage assessments. This implies less reliance on human interpretation, which can sometimes be a source of error.
Of course, with such advanced tools come important caveats. We need to be vigilant about the potential for bias to creep into the AI models, especially since the models are trained on historical data. For example, if past data shows certain demographics tend to file more claims, it's important to make sure the AI doesn't inadvertently perpetuate this bias and lead to unfair outcomes in the future. This requires constant monitoring and retraining of the algorithms.
AI systems appear to excel at analyzing large and complex datasets, which are a challenge for traditional risk models. This ability to extract previously hidden insights from massive amounts of data could be transformative for property insurers.
However, these advancements in efficiency could have a trade-off—as claim processing becomes more automated, there's a potential for job displacement within the industry. This is something that needs to be considered thoughtfully, as companies navigate the transition to more AI-centric processes.
From a financial standpoint, improved accuracy and efficiency mean that organizations can potentially optimize their operations and reduce costs associated with delayed claims. The opportunity to address past financial shortfalls due to inefficiencies is a significant advantage of adopting these technologies.
It's also fascinating to consider how this improved claim processing accuracy could pave the way for new insurance products and services. For instance, on-demand insurance, which adapts to real-time risks, could become more viable with AI's ability to process and react to data more efficiently.
Finally, the rapid rate of change suggests the insurance landscape could look radically different in the next ten years. This creates a need for insurers to continuously innovate and adapt their strategies to stay ahead of the curve and maintain a competitive edge in this evolving market.
AI-Driven Underwriting Models Show 28% Accuracy Improvement in Q3 2024, Study Reveals - Auto Insurance Sector Leads AI Adoption With 89% Major Carriers Using Smart Models
The auto insurance sector is at the forefront of AI adoption within the insurance industry, with a remarkable 89% of major carriers implementing AI-driven smart models for underwriting as of the third quarter of 2024. This widespread adoption has been closely linked to a 28% increase in underwriting accuracy, clearly highlighting the positive impact AI is having on traditional methods. Auto insurers are leveraging AI to analyze telematics and large datasets, resulting in more refined risk assessments and better pricing strategies. But this rapid adoption also exposes potential obstacles. The need to upgrade outdated technology systems within the industry could hinder the implementation of further innovations and the full potential of AI. Looking ahead, the increased use of AI within auto insurance points toward a wider trend of prioritizing data-driven insights, a shift that will undoubtedly change the way the entire insurance landscape functions.
The auto insurance field is showing a strong push towards artificial intelligence (AI), with a remarkable 89% of leading carriers now using sophisticated AI-powered models in their operations. This shift is reshaping how insurers assess risk and handle claims, showing a major change within the industry. It's quite a large-scale adoption and could mean we see new ways of managing risk and handling payouts in the future.
We've already seen accuracy increases in some types of claims, like property claims, where AI is improving accuracy by roughly 31%. These systems seem to be better at dealing with the large and complicated datasets insurers gather. This is a significant upgrade over older ways of doing things that relied on simpler datasets. It’s also notable that they are faster, with AI helping to cut the time to process a simple claim from 48 hours down to just 14 minutes. This suggests the technology can provide a significantly better customer experience by speeding up payouts.
This efficiency isn't just theoretical, insurers are reporting big cost savings related to AI use. Across the industry, companies have managed to save around $440 million. This is a significant financial benefit that’s tied to AI's ability to do things like review claims more quickly and accurately. It's an interesting combination of improved outcomes and lower costs that could fundamentally alter how insurers operate.
However, as AI becomes more central to this field, we also need to think about the potential challenges. AI systems, especially in underwriting, rely on data that reflects the past. It’s something that researchers are beginning to study—the risk of biases in this historical data that could lead to unfair outcomes for some policyholders. Insurers need to ensure they’re avoiding any potential discrimination as they develop and use these tools. Another area of concern is that as claims are handled more through automation, there's a chance some jobs could be eliminated within the insurance industry. We've seen AI replace repetitive tasks in other fields, and this will require careful consideration by insurers to ensure the transition is handled ethically.
Interestingly, AI models appear to be good at noticing subtle things within massive amounts of data. This could be quite useful for tasks like spotting potentially fraudulent claims—patterns that human eyes may overlook. They are able to react to real-time data, allowing for fast changes to underwriting processes and a more nimble approach to assessing risk. The end result of this kind of rapid processing could be more trust between insurers and their customers, due to the faster and likely fairer resolution of claims.
All of these changes have some big implications for how the industry will be regulated. As these AI tools become more widespread, regulators will need to work with insurers to establish fair and transparent practices that protect customers, while also enabling insurers to use these powerful new tools. The future of insurance may well rely on insurers and regulators working together to ensure these technologies benefit both the customer and the industry.
More Posts from insuranceanalysispro.com: