AI-Driven Underwriting How Machine Learning is Revolutionizing Online Car Insurance Pricing in 2024
AI-Driven Underwriting How Machine Learning is Revolutionizing Online Car Insurance Pricing in 2024 - AI Streamlines Data-Intensive Underwriting Workflows
AI is reshaping how insurers handle the complex, data-heavy tasks of underwriting. Machine learning and predictive models are being used to refine risk assessment methods, aiming for greater accuracy and efficiency in the overall process. While a majority of insurance leaders acknowledge the potential advantages of using AI, practical implementation remains limited. We're seeing examples like Cytora using generative AI to evaluate risks, showcasing a move towards more automated and data-centric underwriting. This trend suggests the future of insurance underwriting will increasingly rely on advanced analytics, pushing insurers to adopt these tools if they want to keep pace with the evolving industry. The need to modernize underwriting practices to stay competitive will likely become more urgent as the technology matures.
The application of AI, particularly machine learning, is transforming how insurance companies handle the data-heavy process of underwriting, especially in the realm of auto insurance. We're seeing a significant reduction in underwriting times, potentially up to 70%, by leveraging sophisticated machine learning models, accelerating the entire operational flow. These models are capable of processing hundreds of data points concurrently, allowing for a much more thorough and precise risk assessment compared to traditional methods that are limited in the variables they consider.
This shift towards AI has led to the development of systems that can provide instant feedback to applicants, offering quick approvals or rejections. Previously, this process could take days, creating a bottleneck. Interestingly, these AI systems can unearth patterns in claims data that may elude human underwriters, potentially enhancing fraud detection. Moreover, the ability of algorithms to learn continuously from new data ensures that the underwriting models can adapt dynamically to evolving risk profiles without the need for comprehensive human intervention.
There's a growing interest in using AI to simulate accident scenarios based on driving patterns, leading to a more granular understanding of potential losses and allowing insurers to implement more individualized pricing structures. This year, we've also seen the incorporation of natural language processing into the underwriting process, enabling systems to analyze unstructured data such as online reviews and social media posts. This is a powerful development, offering a broader perspective in risk evaluation.
Despite the clear benefits, it's surprising how many insurers are still clinging to decades-old risk assessment strategies, ignoring the documented effectiveness of modern machine learning techniques. This suggests a potential disconnect between current research and the adoption of these technologies in the industry. However, the growing pressure to improve efficiency and reduce costs will likely drive faster adoption. One major advantage of AI in this domain is its ability to enhance regulatory compliance. AI-driven underwriting can systematically track and scrutinize decision-making processes, simplifying the audit trail, a crucial requirement in the insurance sector.
Early adopters of AI-powered underwriting have experienced a considerable decrease in operating costs, with estimates pointing towards a 40% or more reduction in the resources needed for manual review procedures. This suggests a compelling economic case for broader adoption across the industry. It will be fascinating to see how these developments unfold and influence the future of the car insurance market.
AI-Driven Underwriting How Machine Learning is Revolutionizing Online Car Insurance Pricing in 2024 - Machine Learning Enhances Risk Assessment Accuracy
Machine learning is fundamentally altering how insurers assess risk, especially within the context of online car insurance pricing. By analyzing extensive datasets and employing sophisticated algorithms, insurers can generate more accurate risk profiles for individual policyholders. This increased precision enables them to develop insurance policies that are more finely tuned to specific risk factors, potentially leading to more equitable pricing. Furthermore, machine learning expedites the underwriting process, a significant advantage in today's fast-paced environment.
Beyond speed, machine learning enhances underwriting decisions by detecting patterns and anomalies that might be missed by human underwriters relying on traditional methods. This improved accuracy can lead to better fraud detection and a deeper understanding of risk factors. Despite the clear advantages, many insurers have been slow to adopt these advanced technologies, perhaps due to reluctance to depart from established practices. However, the competitive pressure to streamline operations and reduce costs will likely accelerate the adoption of machine learning in the coming years. Insurers that embrace these tools and leverage the power of data-driven decision-making are poised to adapt to the evolving insurance landscape and remain competitive in a rapidly changing marketplace.
Machine learning algorithms are adept at sifting through massive datasets, encompassing historical claims data and demographic information, to uncover risk factors that might elude human underwriters. This ability to detect intricate, non-linear relationships between variables provides a much more nuanced understanding of risk.
One intriguing aspect of machine learning in this context is its capacity to continuously improve its predictive accuracy. These models aren't static; they evolve and refine their predictions based on new data, leading to quicker responses to shifts in risk environments. This constant learning capability makes them more adaptable.
Research suggests that employing machine learning can significantly reduce underwriting errors, potentially by as much as 30%. This is crucial because underwriting blunders can result in substantial financial setbacks for insurance providers.
Integrating real-time data, like telematics or data from IoT devices, into machine learning models empowers insurers to tailor risk assessments to actual driving behavior and environmental factors. This move away from relying solely on historical data creates a more dynamic and responsive approach to risk evaluation.
Interestingly, some machine learning methods utilize ensemble techniques, which involve combining multiple models to generate a single, more robust prediction. This approach often results in risk assessments that are more resilient and accurate than those produced by any individual model.
Despite the obvious benefits, about a quarter of insurance companies remain cautious about adopting machine learning for risk assessment. Many are concerned about the "black box" nature of some models and find it challenging to interpret how complex algorithms arrive at their conclusions. Understanding and interpreting the 'why' behind a decision is still a challenge for many complex AI systems.
The potential of machine learning to promote fairness in risk assessment is noteworthy. By using diverse and inclusive datasets, these models can help mitigate biases that may be present in conventional underwriting methods, fostering a more equitable pricing structure across various demographics. This potential for equitable decision-making is interesting to explore further.
An unexpected advantage of machine learning in underwriting is its ability to swiftly identify emerging trends and previously unforeseen risks more quickly than traditional systems. This allows insurers to take preemptive actions to potentially prevent losses from escalating.
Studies have demonstrated that machine learning algorithms can handle and interpret unstructured data sources, like customer feedback and online reviews. This inclusion of qualitative data adds a new dimension of contextual understanding that traditional numerical data alone cannot provide. This move towards incorporating human-generated data provides a deeper view.
While machine learning models generally offer superior accuracy compared to traditional approaches, their inherent complexity can present challenges with regulatory compliance. Insurers using these models must ensure that their decision-making processes are transparent and easily justifiable to all stakeholders. The interpretability of models is a growing area of research as the usage of complex AI systems increases.
AI-Driven Underwriting How Machine Learning is Revolutionizing Online Car Insurance Pricing in 2024 - Real-Time Data Integration for Dynamic Pricing Models
In today's dynamic markets, real-time data integration has become crucial for the success of dynamic pricing models. Businesses need to adapt quickly to shifting market conditions, competitive pressures, and customer behavior. By continuously incorporating new information, companies can fine-tune their pricing strategies in real-time, reacting to current demands and interactions. This agility not only helps optimize revenue but also enhances the accuracy of pricing, especially in fields like insurance where risk is constantly changing. Traditional pricing methods often struggle to keep up with the vast and ever-changing data landscape. The ability to integrate and use real-time data provides a significant edge in today's competitive environment, pushing companies towards more complex, AI-driven pricing approaches. While these new methods bring great opportunities, they also present new challenges in data quality, integration, and overall infrastructure management. It's clear that companies need to build robust systems to effectively harness the power of real-time data for optimized pricing strategies.
Real-time data integration for dynamic pricing models can incorporate a vast array of information, potentially up to 50 different data points each second. This constant stream of data allows insurers to adjust prices in response to rapidly changing risk factors, such as weather conditions or traffic patterns. It's fascinating how responsive these systems can be.
One notable feature of real-time data integration is the ability to adjust pricing multiple times throughout a single day. This capacity to react adversarially to market fluctuations represents a stark contrast to traditional insurance models, which rely on static, pre-determined pricing. The agility offered by these dynamic models is quite striking.
The advanced machine learning models that power dynamic pricing can detect subtle trends within real-time data, trends that may be masked by analysis of historical data alone. For example, a slight increase in a particular type of accident correlated with a local event could be identified and factored into risk assessment, making the underwriting process far more contextually relevant.
By integrating real-time data from telematics devices, insurers can refine their algorithms to offer personalized insurance premiums based on individual driving behavior. This personalized approach contrasts with traditional methods that rely on broader demographic groups, potentially leading to a fairer distribution of premiums.
Research has shown that insurance providers using real-time data integration have experienced a substantial reduction in underwriting losses, in some cases up to 25%. This finding suggests that the ability to react to live risk signals can have a significant positive impact on the financial stability of an insurer. This data suggests a compelling argument for adopting real-time data approaches.
Interestingly, real-time data integration allows insurers to conduct predictive analysis not only at the initial point of underwriting but also continuously throughout the life of an insurance policy. This approach means premiums can be adjusted as the insured's risk profile changes, ensuring a more accurate reflection of the risk being assumed.
Despite this evidence, a significant portion of insurers – roughly 65% – believe adopting real-time data integration would provide a major competitive edge. However, many remain hesitant due to the perceived complexities of implementing such systems. It's surprising there's this level of hesitancy given the potential benefits.
Real-time pricing models can be further enhanced by incorporating social media data. This allows insurers to analyze public sentiment and assess its potential impact on insurance claims, providing a unique perspective on community-level risks. This approach introduces a completely new dimension to risk evaluation.
It's quite surprising that despite the clear benefits, fewer than 30% of insurance companies are currently using real-time data analysis for adjusting their pricing. This suggests a notable gap between the availability of technological advancements and their adoption within the insurance industry. It is perplexing to see this degree of reluctance to embrace technological advancements.
While dynamic pricing offers many benefits, it also raises certain ethical concerns. For example, individuals who drive less could potentially receive significantly lower premiums compared to those with higher mileage, creating a potential challenge to the traditional insurance principle of risk pooling. These sorts of ethical considerations will need to be explored further as real-time data and dynamic pricing gain traction.
AI-Driven Underwriting How Machine Learning is Revolutionizing Online Car Insurance Pricing in 2024 - Fraud Detection Improvements Lower Insurance Costs
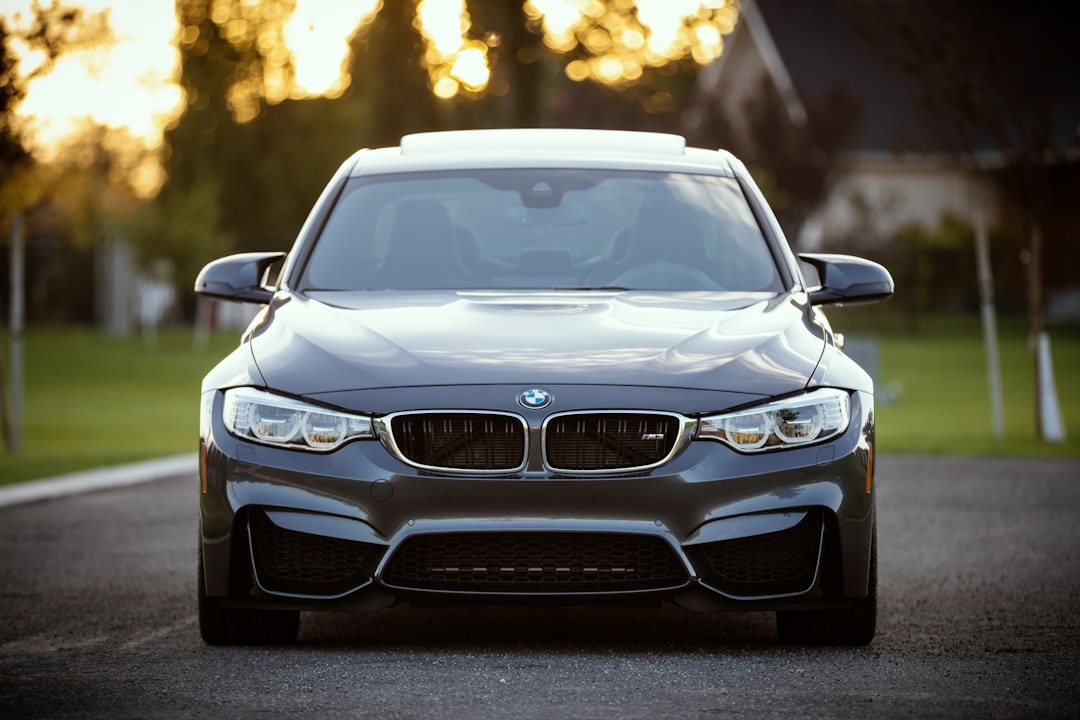
Improvements in fraud detection, fueled by artificial intelligence, are significantly impacting the insurance landscape, particularly in reducing operational costs. Insurance companies face substantial losses due to fraudulent claims, with estimates suggesting that nearly a fifth of auto insurance payouts in the US are linked to fraudulent activity. AI-powered systems are now able to analyze large volumes of data and identify patterns indicative of fraudulent behavior, allowing insurers to more swiftly pinpoint suspicious claims. This enhanced detection capability not only reduces fraud but also simplifies underwriting processes, ultimately leading to lower insurance premiums for consumers. The increasing adoption of these technologies is poised to revolutionize how online car insurance is priced, promoting both efficiency and fairness within the insurance market. While these improvements are promising, the industry will need to grapple with ensuring the responsible and ethical application of these new technologies.
The realm of insurance fraud detection is experiencing a significant shift thanks to machine learning. These models can drastically speed up the process of identifying fraudulent claims, potentially by as much as 60%. This efficiency not only streamlines operations for insurers but also creates the possibility of passing those savings along to policyholders in the form of lower premiums.
AI-powered algorithms are capable of processing claims data in real-time, constantly searching for irregularities and patterns that might signal fraud. This automated approach is a considerable improvement over traditional methods, which usually depend heavily on manual review and take much longer to reach a conclusion. It's remarkable how much more quickly these AI models can spot discrepancies.
It's quite surprising to learn that a substantial portion—almost 80%—of insurance fraud cases go undetected when using conventional fraud detection methods. This staggering figure highlights a crucial need for improved technology. Machine learning systems seem to be emerging as a powerful solution to this issue. The effectiveness of machine learning is quite compelling when one considers this gap in the current state of insurance fraud detection.
Integrating telematics data into machine learning models offers another exciting avenue for improving fraud detection. Not only can telematics enhance risk assessments based on driving behavior, but it can also uncover inconsistencies in reported claims, further improving the detection accuracy of fraudulent activities. It seems like integrating different data sources offers powerful and complementary methods for detecting fraud.
Machine learning is poised to have a considerable financial impact on the insurance industry in 2024. It's estimated that these models could reduce underwriting costs related to fraud detection by up to 35%. This significant reduction is likely to translate to more affordable premiums for those who buy insurance. There is a powerful incentive for insurers to explore this further.
A surprising outcome of implementing better fraud detection is the potential increase in customer trust. About half of all insurers anticipate that improved fraud detection will lead to not only lower costs but also a greater sense of confidence from their clients. The transparency and reliability that comes with an effective system should enhance the relationship between an insurer and those they serve.
Research strongly suggests that predictive models can actually pinpoint fraudulent claims before they're even processed, leading to substantial financial savings for the industry. This remarkable ability potentially represents billions of dollars in savings each year. These types of projections provide a clear financial motivation for the wider adoption of these technologies.
Machine learning offers a tremendous leap forward in detection accuracy. Insurers that use AI for fraud detection can achieve accuracy rates exceeding 90%, a dramatic increase compared to the traditional methods that struggle to reach even 50% accuracy in similar scenarios. The discrepancy between the two approaches is impressive and strongly supports the use of AI in this domain.
One of the remarkable attributes of machine learning models is their ability to learn continuously. They are not static, and they can adapt to new fraud tactics as they emerge. This constant learning and refinement ensure that fraud detection capabilities won't become outdated as criminals develop new methods. It’s a critical feature for ensuring the long-term effectiveness of these systems.
While these improvements in fraud detection technology are very promising, there's still a significant level of skepticism within the insurance industry. About 40% of insurers remain unsure about the reliability of machine learning in fraud detection. This hesitation likely stems from a lack of trust in new and complex technologies. This highlights a challenging dynamic where the gap between the potential of the technology and widespread acceptance of it remains a significant hurdle. It's fascinating to see this interplay between the advancements in technology and market receptiveness to that advancement.
AI-Driven Underwriting How Machine Learning is Revolutionizing Online Car Insurance Pricing in 2024 - Personalized Policies Through AI-Driven Customer Profiling
In 2024, AI is increasingly influencing the creation of personalized insurance policies by analyzing individual customer profiles. Through techniques like predictive analytics, insurers can delve into a wide range of data, from driving behavior to personal lifestyle choices, to develop policies specifically tailored to each customer's risk. This personalized approach can improve customer satisfaction and potentially boost retention rates significantly, with estimates suggesting a possible increase of up to 28%. However, the insurance industry's embrace of AI-powered customer profiling has been somewhat uneven. There's a clear disconnect between the potential benefits and the actual adoption of these methods by many insurers, who might be hesitant to move away from established procedures. As customer demands evolve and competition intensifies, the pressure to integrate advanced AI-driven techniques into policy creation may become a crucial factor for insurers to stay competitive and relevant in the evolving insurance landscape.
The use of AI to create detailed customer profiles is dramatically changing how insurance policies are designed. By analyzing a wide array of data, including driving patterns, location-based risks, and even social media activity, insurers can now generate more accurate representations of individual risk profiles. This level of granularity allows them to develop insurance products that are much more finely tuned to specific circumstances, potentially leading to a more fair pricing structure. It's fascinating to see how AI can simulate numerous accident scenarios based on a person's unique driving history, allowing insurers to more accurately predict potential losses and, in turn, offer more individualized premiums.
Interestingly, research shows that AI-generated profiles can reduce discrepancies in insurance pricing by up to 25%. This is largely due to the reliance on real-time data and the ability to understand nuanced details, which often get lost when relying solely on broad demographic categories used in traditional methods. This precision also has implications for customer acquisition costs. AI-driven targeting allows insurers to focus their efforts on specific segments that match their offerings, leading to potential reductions of up to 30% in these expenses.
One of the most intriguing potential benefits of using AI for customer profiling is the possibility of achieving a more equitable distribution of insurance costs. AI allows for more dynamic pricing, potentially leading to lower premiums for safe drivers while ensuring higher-risk drivers pay a more accurate rate based on their specific behavior, not just broad categorizations. However, this level of personalization also brings up ethical concerns. The use of AI relies on significant amounts of personal data, raising important questions about privacy and data security. It's crucial that insurers build robust systems to protect this sensitive information.
The potential impact of AI-driven personalization extends to customer retention. By offering truly customized insurance solutions, insurers can strengthen the relationship with their policyholders. Some studies have shown that using AI to create personalized policies can lead to an increase in retention rates by as much as 40%. Customers appear to value this individualized approach and feel more appreciated as a result. There's also a potential link between personalized insurance and a reduction in fraudulent claims. It appears AI can identify suspicious behavior patterns in a way that traditional methods often miss, leading to a decrease in fraudulent claims by up to 20%.
Despite the many advantages, the adoption of AI for customer profiling is not without its challenges. There's a lingering concern about potential biases in the algorithms themselves. Roughly half of the insurance industry is hesitant because of this. It's important that these concerns are addressed with ongoing monitoring and adjustments to the models to ensure fairness across different demographics. However, the overall shift towards personalized policies through AI seems poised to fundamentally reshape the insurance industry. This trend is likely to improve customer experience by making the entire process more transparent and establishing a stronger sense of trust between the customer and the insurer. It will be interesting to see how this evolution continues to unfold.
AI-Driven Underwriting How Machine Learning is Revolutionizing Online Car Insurance Pricing in 2024 - Near-Instant Policy Pricing and Binding Capabilities
The demand for faster and more streamlined insurance processes has led to the growing adoption of near-instant policy pricing and binding capabilities. AI-powered systems are making it possible for insurers to price, purchase, and finalize policies in real time. This ability to quickly respond to changing risk factors and market conditions offers significant improvements in efficiency. However, the transition to this new approach is not without challenges. Many insurers still rely on traditional methods, and adopting these new, real-time systems presents complexities. While the potential benefits include greater customer satisfaction and faster service, it's vital that insurers prioritize transparency and ensure their pricing models are fair and equitable. The success of these near-instant capabilities will depend on addressing concerns about the shift away from traditional practices and the careful implementation of AI-driven underwriting.
The insurance industry is experiencing a surge in the adoption of AI-powered tools, especially in areas like pricing and policy issuance. We're seeing a trend towards what could be called "near-instant" policy pricing and binding. Essentially, advanced machine learning models can now price and issue policies in mere seconds, a stark contrast to the traditional process which could take days. These systems can process a vast amount of data—up to 50 different data points every second—and use this to dynamically adjust pricing based on real-time changes in risk. For example, if the weather changes or traffic patterns shift, the policy cost can be adjusted in real-time. This creates a far more responsive system for dealing with the unpredictable nature of risk in the world.
This speed in decision-making isn't just about pricing; it applies to the entire policy process. A policyholder could get near-instant approval, drastically improving the customer experience compared to older systems where approvals could be delayed for days. It's interesting how this speed also enhances the possibility for better risk assessment. These AI models can incorporate data from telematics and other devices to build highly personalized risk profiles, moving away from relying on broad demographic assumptions to those based on actual driving behavior and individual patterns. This shift leads to a much more granular risk assessment, potentially even simulating a large number of accident scenarios tailored to an individual's driving habits.
Furthermore, machine learning systems are proving adept at reducing common mistakes in the underwriting process, potentially lowering errors by up to 30%. This enhanced accuracy benefits both insurers and consumers. Insurers experience lower costs from fewer misjudgments, and customers might see that translate into lower premiums. Interestingly, insurers can also maintain accurate pricing throughout the life of a policy by dynamically adjusting premiums based on the changes in a driver's behavior and risk profile. This proactive approach to pricing ensures a continuous alignment between the risk and the price, which has never been possible before using traditional models.
There's also a potential for AI systems to improve the fairness of insurance by detecting and mitigating possible biases in traditional methods. While the promise is there, it's important to keep in mind the ongoing challenge of ensuring fairness across all demographics. It's fascinating how AI could bring a higher degree of equity to a domain historically prone to unconscious biases in risk assessment. Early adopters are already seeing impressive returns on these technologies, with reports suggesting reductions in operational costs of over 40%. This increase in efficiency might be a strong driver of the adoption of these systems, potentially leading to lower insurance costs overall. The speed and transparency of the entire process also seem to enhance customer trust, as policyholders experience a faster, more direct interaction with the insurer. It's likely that this, in turn, can help foster greater customer loyalty.
However, it remains to be seen how fast the broader industry will adopt these tools, and it's crucial to keep an eye on the ongoing development and evaluation of AI-powered insurance. The rapid development of these new technologies brings both significant advantages and complex ethical considerations that need constant scrutiny.
More Posts from insuranceanalysispro.com: