AI Insurance Policy Analysis and Coverage Checker - Get Instant Insights from Your Policy Documents (Get started for free)
7 Key Technologies Reshaping Car Insurance Telematics in 2024
7 Key Technologies Reshaping Car Insurance Telematics in 2024 - Low Earth Orbit Satellites Enable Rural Coverage For Usage Based Insurance
Low Earth Orbit (LEO) satellites are emerging as a powerful tool to extend usage-based insurance into areas previously underserved by traditional telematics. Their proximity to Earth allows for much faster data transfer and reduced delays compared to traditional satellite systems, which is vital for the real-time data needed by usage-based insurance. A major hurdle for insurers expanding telematics has been the lack of reliable internet access in many regions. LEO satellites potentially address this by offering a relatively straightforward and readily available infrastructure solution, especially in areas where existing infrastructure is limited or non-existent. The fact that these services can be accessed with relatively simple, portable equipment makes them a compelling option for improving communication in such environments. As insurers strive to offer more personalized and nuanced insurance policies based on individual driving habits, the role of LEO satellites in telematics is likely to become increasingly important. Their potential to reshape the landscape of car insurance in these previously difficult-to-reach areas is undeniable.
Satellites in Low Earth Orbit (LEO) are positioned much closer to Earth than the traditional geostationary variety, hovering between 160 and 2,000 kilometers above our planet. This proximity significantly reduces the time it takes for data to travel, achieving latency as low as 20 milliseconds. This speed is essential for usage-based insurance (UBI) because telematics data needs to be transferred in real-time to be effective.
There's been a huge surge in the deployment of LEO satellite constellations lately, with numerous companies launching thousands of smaller satellites to achieve broad coverage. This is especially beneficial for rural areas that are often underserved by conventional cellular networks. Because of their ability to provide connectivity in these remote locations, insurers can now leverage LEO satellites to collect more comprehensive telematics data. This, in turn, allows them to more accurately gauge driver behavior and tailor premiums to reflect individual risk profiles.
Unlike traditional satellites, which can take hours to transmit data between a vehicle and a server, LEO satellites are far more responsive. They can relay data almost instantly, which is crucial for fast reactions in emergency situations, prompt policy adjustments, or simply ensuring timely access to critical information for drivers. Especially in remote locations where the standard infrastructure is minimal, the satellite network can provide emergency response tracking and real-time traffic updates. This ensures safety by delivering timely insights when drivers need them.
LEO satellites hold the potential to elevate predictive capabilities in insurance models. By collecting a richer dataset from even geographically scattered populations, previously elusive risk patterns may become apparent. This enhanced understanding will likely improve how insurers evaluate and price insurance policies. It could also lead to significant operational efficiency gains because the scalability of LEO networks allows for rapid expansion of coverage without the need for extensive ground infrastructure improvements, making the overall insurance operation more cost-effective.
While the improved data quality from LEO satellites offers advantages, there are aspects to consider regarding the accuracy of data collection. Since LEO satellites are relatively close to the surface, they can achieve a higher resolution when collecting data compared to their geostationary counterparts. This will help in improving the accuracy of mileage calculations and other metrics used in UBI. It's worth investigating the impact of this enhanced resolution on the accuracy of telematics data and how it ultimately influences risk assessment and policy adjustment for insurers.
Furthermore, in areas prone to natural disasters, having resilient communication channels like LEO satellites becomes even more valuable. These networks can keep telematics systems operational even when ground-based communications systems are disrupted, allowing insurance assessments to continue during times of crisis. It's important to continue to explore the role of LEO satellite technology in making insurance more adaptable and robust in the face of environmental challenges and unexpected situations.
7 Key Technologies Reshaping Car Insurance Telematics in 2024 - Predictive Analytics Using Mobile Edge Computing And 5G Network Data
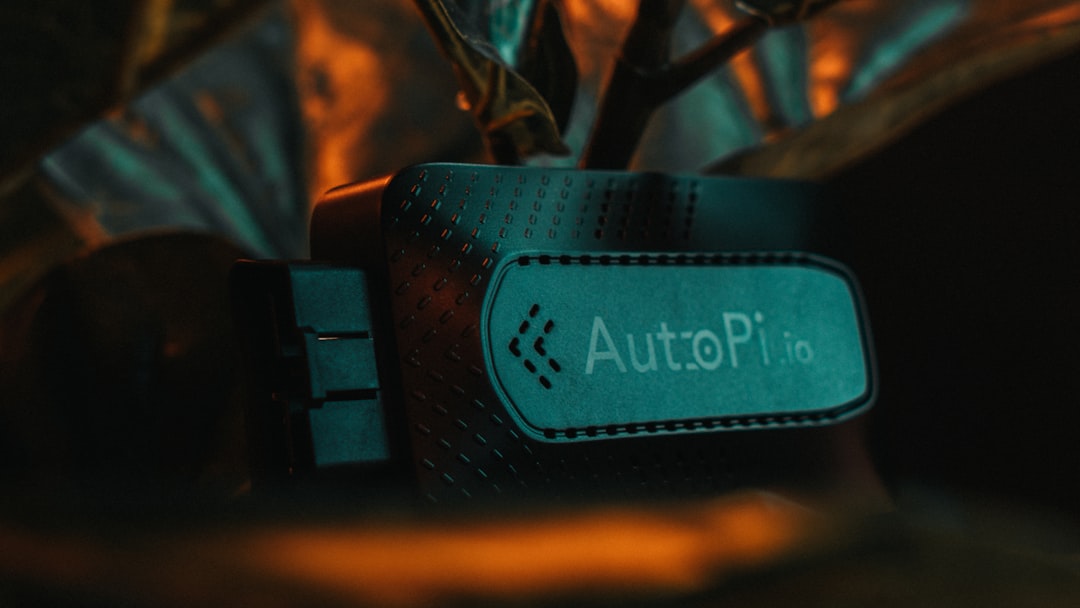
The convergence of predictive analytics, mobile edge computing (MEC), and 5G networks is significantly altering the landscape of car insurance telematics. By enabling data processing at the edge, closer to the vehicle, MEC drastically reduces delays and improves the responsiveness of services. This is particularly crucial for risk assessment and prompt claims handling. Integrating machine learning within this setup empowers insurers to better forecast changes in demand and pinpoint driving behaviors with greater accuracy. The ultra-fast speeds and low latency of 5G networks unlock the potential for advanced applications in telematics. This includes the ability to personalize insurance offerings and effectively manage network resources through strategies like network slicing. The overall effect of this technology combination is a transformation of the car insurance industry, driving more streamlined claims processing and the development of more precise risk profiles for individual drivers. It's a shift toward a more agile, data-driven approach to insurance. While promising, it's important to consider potential challenges related to data security and privacy in this new environment.
The convergence of mobile edge computing (MEC) and 5G network data is fundamentally altering the landscape of predictive analytics in car insurance telematics. By pushing data processing closer to the source, like inside a vehicle, MEC minimizes delays, enabling real-time insights into driver behavior. This is especially important with the emergence of 5G, which provides a quantum leap in data speeds, allowing for the analysis of massive datasets generated by connected vehicles.
Imagine being able to predict when a vehicle might need maintenance before it actually breaks down. This is becoming a reality through the power of MEC-based predictive analytics. By examining data streams from vehicle sensors, it's possible to anticipate maintenance requirements and potentially prevent breakdowns. From an insurer's standpoint, this can contribute to a smoother claims process and fewer claims associated with unexpected breakdowns.
Further, this dynamic duo creates a pathway to more detailed driver profiles. Through continuous monitoring of driving behavior and related data, insurers can craft more personalized risk assessments. This allows for fairer and more nuanced premium adjustments based on individual driving patterns instead of relying solely on broader demographic factors.
However, this ability to glean a deeper understanding of driver habits raises interesting questions about the use and privacy of sensitive information. It's crucial to ensure that data collection and processing protocols are secure and protect drivers from potential privacy breaches.
The advancements in this area also extend beyond individual drivers. Geolocation data, processed with MEC-powered predictive analytics, could potentially be used to suggest alternative routes that avoid accident-prone zones. Such location-based services might help improve driver safety and contribute to a reduction in accidents.
Furthermore, 5G and MEC's lightning-fast data transmission speeds can be a game-changer in emergency situations. Should an accident occur, a rapid notification to emergency services can be facilitated, hopefully improving outcomes for those involved and accelerating the claims process.
Of course, as with any emerging technology, there are hurdles to overcome. One of the major challenges will be scaling up MEC and 5G infrastructure to match the growing number of connected vehicles. If these technologies are not scaled thoughtfully, their potential benefits might not reach their full potential in diverse environments and across a wide range of applications.
Also, machine learning models can be utilized to identify anomalous driving patterns in real-time, allowing for timely interventions by insurers. For example, if erratic driving behavior suggests an elevated risk of accidents, an insurer could provide proactive feedback to the driver. This could lead to improved driver behavior and a more effective approach to managing risk.
Businesses that run vehicle fleets can also reap benefits from these new capabilities. Using MEC and 5G to improve fleet management means optimizing routes, reducing inefficiencies, and improving driver safety, leading to better operational costs and improved safety outcomes.
The future of telematics in car insurance is being driven by the innovations in MEC and 5G. However, we must be mindful of the potential challenges and focus on robust and secure data protocols to ensure that this technological evolution ultimately benefits all parties involved.
7 Key Technologies Reshaping Car Insurance Telematics in 2024 - Vehicle To Everything Communication Standards Track Group Driving Behavior
Vehicle-to-Everything (V2X) communication is a growing technology that's changing how people drive and making roads safer. It essentially allows vehicles to "talk" to each other, infrastructure like traffic lights, pedestrians, and even online services. This interconnectivity can lead to a smoother and safer driving experience. The US government's focus on V2X initiatives, like "Saving Lives with Connectivity", highlights the potential for significantly reducing traffic accidents. The rise of technologies like Cellular Vehicle-to-Everything (CV2X) is improving driver awareness in real-time, providing them with more information to make better decisions and potentially avoid accidents. This interconnected environment is influencing traffic management, and also leading to vehicles that are more autonomous and rely on a network of information. However, with increased reliance on shared data, comes important considerations about security risks and ethical questions around data privacy in this connected landscape.
Vehicle-to-Everything (V2X) communication is an interesting area of research, especially when considering how it can influence driving behavior, not just individually but collectively. It's more than just cars talking to each other. V2X encompasses communication with infrastructure, pedestrians, and cloud services, essentially creating a network of information exchange that can significantly impact road safety and traffic management. The US Department of Transportation's National Deployment Plan for V2X is an example of how seriously this technology is being taken, aiming to reduce roadway accidents.
One intriguing aspect is the potential for analyzing group driving behavior. Imagine a scenario where vehicles can share information about sudden braking or erratic lane changes with others nearby. This could lead to improved safety by providing a heads-up to drivers before a potentially dangerous situation unfolds. It's like having a collective awareness on the road. Initial studies suggest a possible 30% reduction in traffic congestion through this coordinated movement and route optimization based on real-time data. This concept opens up the possibility of not only preventing accidents but also making commutes smoother.
Another interesting development is the integration of V2X into collision avoidance systems. By communicating intentions, such as lane changes or braking, vehicles can create a proactive safety net. This real-time data sharing can enhance the effectiveness of existing safety systems. It's a step beyond just reacting to danger and into actively preventing it.
V2X also enhances a driver's overall perception of their surroundings by offering relevant contextual information. Imagine receiving alerts about pedestrians, cyclists, or road obstacles. In complex environments, this added awareness can be particularly useful for making safer and quicker decisions.
The insurance industry is also taking notice of V2X's capabilities. The idea is to leverage the data to refine existing risk assessment models. If we can observe how groups of drivers behave in certain situations, we can potentially create more accurate insurance premiums. It's a more nuanced approach than relying solely on broad demographic factors.
Furthermore, the technology holds the potential to promote better driving behavior through the analysis of collective actions. If we can observe that certain patterns are common amongst groups of drivers, we could design feedback mechanisms – reminders, incentives or other nudges – to encourage safer driving.
The ability of V2X systems to dynamically react to environmental factors is quite powerful. If weather conditions change – like heavy rain – vehicles could adapt their speed recommendations collectively. This shared understanding of changing conditions can enhance safety in a coordinated fashion.
The advancement of autonomous vehicles is heavily reliant on V2X technology. It provides the vital information that autonomous vehicles need to navigate complex environments and make decisions in real-time, taking into account the actions of nearby human drivers.
One challenge that remains is the need for global standardization. Different regions might adopt separate communication protocols, creating compatibility issues. It'll be interesting to see how regulatory bodies and manufacturers come together to resolve these interoperability issues.
Overall, V2X holds tremendous potential for enhancing safety and traffic management. As this technology matures and is more widely adopted, it's likely to have a considerable impact on our driving experiences and the safety of our transportation systems. It's an area that will be worth watching closely in the coming years.
7 Key Technologies Reshaping Car Insurance Telematics in 2024 - Advanced Driver Assistance Systems Feed Real Time Risk Assessment
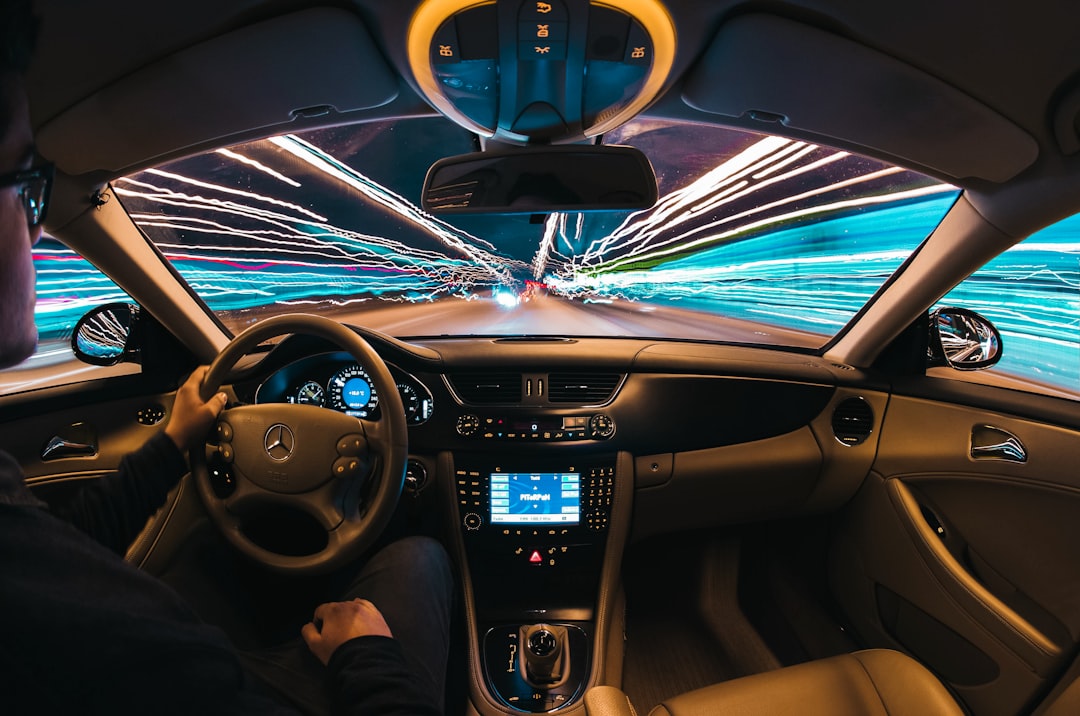
Advanced Driver Assistance Systems (ADAS) are no longer just safety features, but are increasingly becoming integral to real-time risk assessment during driving. Systems like Blind Spot Warning and Automatic Emergency Braking, while improving safety, also generate data valuable for insurance purposes. Algorithms incorporated into ADAS can calculate lane change risks and offer early collision warnings, giving insurers a more granular understanding of driver behavior and associated hazards. The integration of AI and deep learning within ADAS further enhances this capability, allowing for real-time detection of things like driver drowsiness, and providing more refined risk profiles. This enhanced data potentially leads to changes in how insurance policies are designed and priced. The increasing use of ADAS arguably allows both drivers and insurance companies to be more responsive and knowledgeable about risks, but also raises concerns about data privacy and security in this more connected world.
Advanced Driver Assistance Systems (ADAS) are increasingly becoming integrated into vehicles, influencing how we assess risk in real-time. They combine a variety of sensors, such as cameras, radar, and laser-based systems (LiDAR), to build a detailed picture of a vehicle's surroundings. This sensory fusion lets ADAS systems detect potential hazards from various directions and distances, providing a more nuanced understanding of the risks present. It's like giving a car extra eyes and a heightened awareness of its environment.
The beauty of these systems is that they aren't static. Machine learning algorithms are built into many ADAS setups, allowing them to adapt to different driving conditions and scenarios over time. As they gather more data from diverse environments, they become increasingly proficient at predicting risk. Think of it as the system constantly learning and refining its understanding of risk. This continual refinement makes the risk assessments more precise over time.
ADAS systems also often incorporate sophisticated collision avoidance mechanisms that react much faster than a human driver. When sensors detect an impending collision, these systems can instantly initiate braking or steering maneuvers, sometimes in milliseconds. In many situations, ADAS interventions have the potential to significantly minimize the severity or even avoid accidents entirely.
Beyond just the environment, many ADAS features also monitor the driver's actions, such as reaction times and decision-making patterns. This driver behavior data is highly valuable for insurers seeking to create personalized risk profiles for individual drivers. By blending environmental data with driver behavior, insurers gain a holistic view of factors contributing to risk. This could potentially lead to more customized insurance policies based on how a driver typically behaves behind the wheel, in addition to standard criteria.
Furthermore, some ADAS systems are equipped to predict when a vehicle might need maintenance or repairs before a breakdown actually happens. They can detect subtle changes in the vehicle's performance that might otherwise go unnoticed. This can proactively flag the need for repairs, increasing safety and likely reducing claim payouts for insurers.
ADAS features can also incorporate location data through GPS, allowing for the implementation of digital boundaries, or geofencing. This ability to detect when a vehicle enters a high-risk area, like a notoriously accident-prone section of road, can trigger alerts and help insurers assess risk in specific areas.
The data collected by ADAS is fundamentally changing how insurance companies price policies. Rather than relying on traditional methods based on generalized demographics, insurers can potentially shift towards models that dynamically adjust premiums based on real-time driving behavior. This could lead to a fairer system that rewards safer drivers with more affordable rates.
In the event of a mishap, some ADAS systems can even integrate with emergency services, providing the exact location of the accident, along with vehicle information. This can significantly speed up response times and lead to streamlined claims processes.
But with the widespread collection of personal driving data comes a very real concern: data privacy. With ADAS systems continually tracking our behavior, questions arise regarding how this information is stored, used, and protected. Strict measures must be established to ensure that driver information is treated with the utmost respect and confidentiality.
Finally, the use of ADAS in vehicles raises legal and ethical considerations, especially as autonomous systems become more prevalent. In situations where ADAS intervenes to prevent an accident, it's critical to establish a clear understanding of who is accountable: the driver, the vehicle manufacturer, or perhaps the technology itself. Navigating this will likely require new insurance policies and regulations to account for these scenarios.
As ADAS features become commonplace in vehicles, they will profoundly reshape how we perceive and manage risk while driving. While there are still issues to resolve concerning privacy and legal responsibility, it's clear that ADAS technologies are contributing to a more data-driven approach to safety and insurance.
7 Key Technologies Reshaping Car Insurance Telematics in 2024 - Blockchain Based Smart Contracts Automate Claims Processing
Blockchain technology, specifically through the use of smart contracts, is poised to revolutionize how car insurance claims are handled. These self-executing contracts are designed to automate the claims process, potentially speeding up claim settlements and reducing administrative burdens. They achieve this by comparing policy terms with information from trusted external data sources, which enables automatic claim approvals or rejections based on predetermined criteria. This automated approach allows for the exploration of novel insurance concepts, like parametric insurance, where payouts are triggered by external events (like weather or flight delays) without the need for individual claim assessments. The inherent transparency and efficiency of blockchain-based smart contracts can also boost trust and transparency across the insurance landscape, fostering improved relationships between insurers and customers. As blockchain continues to gain traction, the changes it's expected to bring to the way claims are processed and managed within car insurance could significantly alter the industry's operating environment by the end of 2024. While promising, potential obstacles related to the security and privacy of data within this new system still require careful consideration and solutions.
Blockchain technology, with its inherent ability to record and verify transactions immutably, is increasingly being explored by car insurers to streamline the claims process through the use of smart contracts. These contracts are essentially automated agreements written in code, that can execute specific actions when certain conditions are met. This means that claims can be settled automatically, based on pre-defined criteria, and potentially reducing delays significantly. For example, if a car is damaged in an accident, and the agreed-upon conditions are met, a smart contract could initiate the repair process or even release payment directly to a repair shop, all without the need for a human intermediary.
The transparent and immutable nature of blockchain is a key benefit. Every step of the claim process is recorded on a public ledger that's accessible to everyone involved, from the insured to the insurer to the repair shop. This increased transparency fosters trust and reduces the chance of disputes, as all parties can readily verify the validity of the transactions. It's also worth noting that smart contracts can be designed to include specific compliance checks, ensuring that all relevant regulations are followed, thereby reducing the chances of insurers incurring penalties related to compliance failures.
While eliminating human intervention can lead to significant gains in efficiency, it also raises the question of how unforeseen circumstances or subjective aspects of claims would be managed. A focus on standardized templates for the smart contracts is a possible solution for minimizing these kinds of concerns, and we'll likely see an increasing level of standardization in these types of agreements as the technology matures.
Another interesting area is the ability of these smart contracts to incorporate external data. Imagine a scenario where telematics data, connected to a smart contract, automatically triggers a claim if the vehicle's sensors detect certain conditions. This capability allows for a more responsive claims process and can even support new types of insurance products, like parametric insurance where payouts are tied to external events, for example, weather data.
The prospect of integrating real-time data from telematics and other sources into the smart contracts presents an intriguing possibility for dynamic pricing of insurance policies. Instead of premiums being static for long periods, smart contracts can be programmed to adjust premiums based on an individual driver's risk profile, which is continuously assessed by the telematics data. While seemingly beneficial for insurers, the ethical considerations and potential biases built into these algorithms need careful examination.
However, a key aspect to remember is that this technology, while showing promise, is still under development in the insurance world. While it has been successful in other industries, there are a lot of factors in the car insurance domain that need to be accounted for to make widespread adoption a reality. There's also the matter of scalability, as handling a high volume of claims in a completely automated fashion will require robust infrastructure.
The idea of integrating blockchain into the car insurance ecosystem is undoubtedly fascinating. It has the potential to reshape claims processing significantly by automating certain steps and reducing administrative burden, while increasing transparency. But we still have to explore many crucial aspects related to standardization, data privacy, ethical considerations, and operational challenges. It will be fascinating to observe how these areas develop and influence the broader landscape of car insurance over the coming years.
7 Key Technologies Reshaping Car Insurance Telematics in 2024 - Digital Twins Monitor Vehicle Health And Predict Maintenance Needs
Digital twins are virtual representations of physical vehicles, powered by real-time data from sensors and other sources. They're becoming increasingly important in the automotive industry, particularly for monitoring vehicle health and anticipating maintenance needs. Essentially, these virtual twins provide a constant stream of data on a vehicle's condition, allowing for proactive maintenance scheduling and potentially extending the life of vehicles. Companies like General Motors and Tesla are using this technology to predict potential problems and schedule maintenance before issues arise, which can lead to increased operational efficiency and fewer unexpected breakdowns. The ability to monitor vehicle health in real-time also has implications for insurance. By integrating digital twins with the Internet of Things (IoT), insurers can gain a far more detailed and precise picture of a vehicle's health, allowing them to create more accurate and personalized risk profiles for insurance customers. While the potential of this technology is significant, it's important to be mindful of any challenges that might arise with the increased reliance on complex, connected systems. As digital twin technology matures, we'll likely see its impact across various aspects of vehicle life, from design and manufacturing to ongoing maintenance and insurance.
Digital twins are essentially virtual replicas of physical vehicles that leverage real-time data from various onboard sensors to monitor their health. It's like having a constant stream of information about a vehicle's condition, allowing for the immediate identification of irregularities and potential issues. This continuous data flow can potentially prevent breakdowns before they happen, which is a significant improvement compared to relying solely on traditional, periodic maintenance schedules.
These virtual twins aren't just passive observers, they employ advanced predictive analytics to sift through historical and current vehicle data. Based on this analysis, they can forecast when maintenance will likely be necessary with surprising accuracy. Studies suggest that by implementing these predictive technologies, unexpected repair costs can be slashed by up to 20%, making operations smoother and potentially contributing to lower premiums.
However, digital twins don't only look at the car in isolation. They can integrate data on the conditions in which the vehicle operates, how it's driven, and even environmental factors. This comprehensive approach produces a more complete picture of the vehicle's performance and its maintenance needs. It's more than just checking if the oil needs changing. They also provide context that can be helpful in understanding what is causing certain issues with the vehicle.
One of the advantages of incorporating digital twins is the potential for cost savings. Manufacturers and insurers can leverage the data to streamline operations and reduce costs. It's been estimated that fleet operating costs can drop by 10-15% due to less downtime from unplanned repairs. Of course, there are upfront costs in integrating this technology, so a cost-benefit analysis is always critical. It's important to verify the actual cost savings in various situations.
But perhaps the most interesting aspect is the way it provides insights that help refine insurance models and better assess risk. Digital twins can utilize massive amounts of data from telematics and past performance records to build a detailed profile of the vehicle and its use. This data can be combined with other information, such as driver behavior and location data, to refine the insurance underwriting process, making risk assessment more precise.
As this technology improves and data collection becomes more ubiquitous, we will likely see a shift towards usage-based insurance. The idea is to adjust insurance premiums dynamically based on how a vehicle is actually used. Safer drivers, or those that take better care of their vehicles, could end up with lower insurance premiums. Of course, any shift to usage-based insurance requires careful consideration and thorough transparency in how the insurance premiums are calculated.
There are exciting opportunities to combine digital twins with Advanced Driver-Assistance Systems (ADAS). Digital twins can be used to create simulated driving environments based on real-time conditions, enabling the system to anticipate and address potential maintenance needs more effectively based on driving behavior and conditions. It’s like the car is thinking ahead and planning for maintenance needs before they develop into major problems.
Moreover, the technology extends its influence throughout a vehicle's entire lifespan. This means that from the design and manufacturing stages to post-sale maintenance, manufacturers can effectively track and manage a vehicle's lifecycle through its digital twin. This could improve the overall lifespan of vehicles while optimizing repair schedules.
Interestingly, digital twins can be utilized for benchmarking a vehicle's performance against others within the same class or against industry-wide standards. This comparative analysis can furnish valuable information to both manufacturers and insurers, enabling them to identify potential risks early on.
The implications for safety are also quite significant. By constantly monitoring key vehicle indicators, digital twins can assist in triggering proactive safety protocols. If a certain part or system is degrading, or nearing failure, the digital twin can inform decisions and prompt preventative measures like a recall or a focused maintenance inspection. The goal is to identify issues before they lead to critical failures and potentially increase the safety of vehicles overall. Of course, these safety protocols need to be carefully designed to prevent overuse, but it is a promising new area of development.
The use of digital twins in vehicle health management is certainly an area of active development. There are still questions to be resolved in areas like the cost-effectiveness of these models for insurers and manufacturers. However, the potential for improved safety, optimized maintenance, and enhanced insurance offerings is undeniable. We will likely see the use of digital twins continue to expand throughout the coming years, particularly in the automotive industry.
7 Key Technologies Reshaping Car Insurance Telematics in 2024 - Machine Learning Algorithms Detect Insurance Fraud From Telematics Data
The use of machine learning algorithms is transforming how insurance companies detect and prevent fraud within the context of telematics data. By analyzing vast quantities of driving data, these algorithms can spot unusual patterns and driver behaviors that suggest fraudulent claims. The algorithms are designed to learn and adapt, improving their fraud detection abilities over time as they encounter new and evolving fraudulent schemes, based on the historical data they've processed.
There's a growing interest in understanding the relative effectiveness of various machine learning techniques, with methods like Random Forest, Support Vector Machines, and K-Nearest Neighbors being actively explored. The goal is to find the best models for specific insurance fraud challenges.
The insurance industry faces substantial financial losses due to fraudulent claims, making the need for sophisticated fraud detection tools, like machine learning algorithms, increasingly important. While the application of these algorithms holds promise in minimizing these losses and potentially leading to lower premiums for honest policyholders, it's crucial to acknowledge the ongoing research into these models and the need to continually evaluate their performance. Furthermore, there are still challenges to overcome, particularly balancing the need for effective fraud prevention with the importance of safeguarding driver data and privacy.
Machine learning is changing how insurance companies tackle fraud by analyzing massive amounts of data from telematics systems and recognizing suspicious patterns. These algorithms are designed to learn and adapt, improving their ability to detect fraud over time by identifying new tricks used by those trying to cheat the system. There's a lot of discussion about whether supervised or unsupervised learning works best for this, although research on unsupervised methods for this purpose is still relatively limited.
The most advanced fraud detection systems are using the latest AI and machine learning specifically tailored to the problems that the insurance industry faces. Some of the common algorithms used are things like Random Forest, Support Vector Machines, and K-Nearest Neighbors. Insurers are using this technology to train models on telematics data, along with other things like customer and vehicle information, to assess risk and detect fraud. There have been a few initial studies and from what I've seen, different models have varying levels of success in identifying fraudulent claims, which shows us the need for more rigorous testing.
One intriguing finding is the potential to significantly speed up claims processing with AI. For example, Ping An Insurance in China is using AI to process claims 4000 times faster than before. Beyond just speed, machine learning offers a potential path to cutting down on losses from fraudulent claims, potentially leading to lower premiums for honest policyholders. It's becoming increasingly important for insurers to use machine learning to detect fraud, especially considering the escalating costs of this problem to the industry.
It is interesting to note that these machine learning models can be surprisingly nuanced. They're not just looking at simple metrics. These models can factor in thousands of data points from telematics data. Think about things like a driver's braking habits, how fast they accelerate, their route deviations, or even where they tend to drive. These details allow for more complex patterns of potentially fraudulent behavior to be recognized. For example, algorithms are good at identifying anomalies, or things that stand out compared to what's typically seen. They can potentially notice unusual driving patterns that might indicate fraudulent behavior. This technology can analyze telematics in real-time, so if something suspicious happens, the insurance company can potentially react right away, making fraud prevention more effective.
But it's not just about reacting to fraud. These algorithms are designed to adapt and improve over time as they process new data. So as new fraud techniques emerge, the system is capable of evolving and becoming better at recognizing those patterns. Also, these systems can assign risk scores based on driver behavior, potentially helping prioritize cases that require more detailed investigation. Furthermore, machine learning models can use vast amounts of historical telematics data to help identify normal behavior for drivers, making it easier to identify deviations that could be related to fraudulent activities. Some of the most advanced techniques even look at patterns across groups of drivers, identifying trends that can highlight potential fraud. Beyond just the numbers, it's possible to use machine learning to get deeper insights into driver behavior. Using telematics, insurers can gain a more comprehensive understanding of not just how someone drives, but possibly even psychological elements of driving patterns, which could enhance fraud detection strategies. These systems are also able to provide feedback to drivers if they display risky behaviors, which could not only help identify fraud but also potentially prevent future incidents through driver education.
The bottom line is that implementing machine learning for fraud detection can really help cut down on operating costs for insurance companies, because it automates things that were previously done manually. This can lead to faster investigations and a reduction in fraudulent claims payouts. While this is an exciting area of development, we must be mindful of any potential biases that might creep into these algorithms and ensure that any driver data used is treated with the appropriate level of care and protection.
AI Insurance Policy Analysis and Coverage Checker - Get Instant Insights from Your Policy Documents (Get started for free)
More Posts from insuranceanalysispro.com: