AI Insurance Policy Analysis and Coverage Checker - Get Instant Insights from Your Policy Documents (Get started for free)
7 Critical Factors Behind the 24% Increase in Online Auto Insurance Quote Accuracy in 2024
7 Critical Factors Behind the 24% Increase in Online Auto Insurance Quote Accuracy in 2024 - Advanced AI Quote Algorithms Cut Pricing Error Rates From 31% to 7% by October 2024
By October 2024, sophisticated AI algorithms used in online auto insurance quotes are estimated to have significantly lowered pricing errors, a drop from a substantial 31% down to a mere 7%. This sharp reduction exemplifies a wider trend of improved accuracy in online quotes, a 24% jump seen in 2024 due to a convergence of technological and data-driven factors. The core of this improvement rests on the deployment of machine learning and predictive analytical techniques. These allow AI systems to progressively learn and refine their pricing models. Yet, these technological strides, while promising more precise and efficient insurance pricing, have also prompted worries about potential coordination amongst insurers. This suggests that, as AI increasingly impacts the industry, careful consideration and oversight of these new pricing methods will be necessary.
By October 2024, the application of sophisticated AI algorithms within online auto insurance quoting has demonstrably decreased pricing error rates from a concerning 31% down to a much more manageable 7%. This remarkable achievement highlights the power of machine learning to refine and ultimately reshape the way traditional pricing models operate, potentially influencing other insurance sectors as well.
It's fascinating to see how these AI models leverage vast amounts of data to develop a more nuanced understanding of customer profiles and risk factors. This detailed analysis contributes to the generation of premiums that are more aligned with individual circumstances.
The journey to this 7% error rate involved ongoing refinement through iterative training. Essentially, the AI systems learned from past mistakes and continuously adapted based on the latest data being fed into the models. This constant feedback loop is key to the algorithm's ability to stay current.
Before these AI-driven pricing solutions, the auto insurance world relied heavily on older statistical methods. These methods, while functional, often struggled to accurately reflect the complexities of the marketplace, leading to inefficient and sometimes inaccurate pricing.
These advanced algorithms leverage a powerful combination of historical pricing data, insights into customer behaviour, and real-time market fluctuations. This multi-faceted approach offers a much more comprehensive and detailed picture compared to older, simpler methods.
One of the more positive effects of these more accurate quotes is a noticeable drop in customer complaints regarding quote discrepancies. This suggests a marked increase in customer confidence and satisfaction with the transparency and fairness of the pricing process.
Furthermore, the increased accuracy has the potential to contribute to a reduction in fraudulent claims. By identifying patterns in customer behaviour that deviate from typical norms, these algorithms can potentially flag suspicious activity for further investigation.
It's important to note that these AI models aren't static. They are continuously fed new information, allowing them to stay aligned with evolving regulations and market changes. This adaptability ensures they remain relevant and effective in the long term.
Beyond improved accuracy, businesses using these advanced algorithms report substantial gains in operational efficiency. Resources previously tied up in correcting pricing errors can be reallocated towards areas like enhancing customer service or developing innovative insurance products.
The success of these AI algorithms in auto insurance has naturally sparked interest in their potential application to other domains like health and property insurance. It remains to be seen how these AI-powered pricing strategies will continue to evolve and impact the broader insurance landscape. There are, of course, concerns that will need careful consideration as the technology becomes more prevalent.
7 Critical Factors Behind the 24% Increase in Online Auto Insurance Quote Accuracy in 2024 - Real Time Vehicle Data Integration Through Connected Car Systems Improves Quote Precision
The integration of real-time vehicle data from connected car systems is transforming how auto insurance quotes are calculated, leading to a marked improvement in their accuracy. Insurers can now tap into a continuous stream of information about a vehicle's behavior and condition, offering a much more nuanced understanding of risk than traditional methods. This has resulted in a significant 24% increase in the precision of online auto insurance quotes in 2024.
While this heightened accuracy offers immediate benefits to both insurers and consumers in terms of fairer and more transparent pricing, it also hints at a potential shift in how insurance is structured. It's plausible that we could see a transition towards usage-based or "pay-as-you-go" models, where premiums are dynamically adjusted based on real-time driving data. This presents both opportunities and challenges, as insurers will need to carefully balance the desire for precise risk assessment with concerns about data privacy and fairness.
Ultimately, the adoption of connected car data is reshaping the auto insurance industry. It promises a future where policies are tailored to individual driving habits and risk profiles, fostering a more personalized and potentially equitable insurance experience. However, it's crucial to acknowledge that this shift will likely prompt a reevaluation of existing insurance models and necessitate a careful consideration of potential ethical implications.
The integration of real-time vehicle data through connected car systems has shown potential in refining the precision of auto insurance quotes. By leveraging live information from sensors and onboard computers, insurers can move beyond traditional methods that often rely on broad generalizations. We're seeing a shift towards a more individualized approach to pricing. For example, data about braking patterns, speed, and even engine health can feed into risk assessments in ways that were previously impossible.
It's fascinating to consider the sheer volume of data these systems can generate. It's not uncommon for these systems to gather over a thousand data points every second, providing insurers with a real-time view of driver behavior. This means the potential for dynamically adjusting insurance premiums based on how someone actually drives. Theoretically, this leads to rewards for safe drivers through personalized discounts. However, this raises concerns about what constitutes "safe" driving and how these parameters are established and if there is too much of an emphasis on monitoring.
These systems aren't just about detecting risky driving habits either. They can help pinpoint anomalies and react to them in real time, potentially leading to swifter claims processing. Researchers have estimated potential reductions in claims processing time of up to 30%, largely because of the immediate access to incident data, minimizing guesswork when determining fault. Yet, it's unclear whether this increased speed is always beneficial to all parties involved.
Connected car data also offers the potential to move away from broad classifications of risk towards a more granular understanding of individual driving behavior. This may translate to more fair pricing, as the system can distinguish between habitual and occasional risky driving, potentially promoting responsible behavior. Yet, this approach may also introduce more volatility in premiums as individual driving habits constantly change.
However, the increased reliance on real-time, external data presents some challenges, namely concerns around data privacy. There needs to be a careful balance between leveraging this data for improved quotes and respecting the rights of drivers to control how their data is used and ensuring the information is truly being used fairly. Further complicating the matter are recent research findings suggesting behavioral algorithms tied to connected vehicles may lead to more accurate predictive models for risk and potential claims, raising questions about how that level of foresight will be used and if it will impact drivers' access to insurance.
The future holds potential for even more sophisticated analysis. As more vehicles connect to the internet of things, the cumulative data pool will become a powerful resource for analyzing market trends and informing broader insurance strategies. This could potentially impact how insurance is packaged and provided as new insights into larger demographics emerge and may also create unique situations. The potential implications, both positive and negative, for drivers and the industry, require careful consideration as the technology evolves.
7 Critical Factors Behind the 24% Increase in Online Auto Insurance Quote Accuracy in 2024 - Nevada Insurance Claims Database Now Shares 89% More Accident History Details
Nevada's insurance claims database has undergone a significant change, now sharing nearly 90% more information about past accidents. This increased level of detail is meant to help insurers better understand risk and potentially refine how they assess and price insurance policies. The hope is that by having access to a more complete picture of accident history, insurers can develop more accurate and fair insurance premiums. This development fits into a broader trend of increasing data use and more sophisticated technologies like AI and connected car systems within the insurance sector, all aimed at improving accuracy. However, it's crucial to acknowledge that expanding the scope of data collection and usage will inevitably raise questions about how pricing models are impacted and how consumers' data is protected and used in a way that is not discriminatory or prejudicial. As this trend continues, it will be important to consider the implications carefully.
Nevada's Insurance Claims Database has significantly expanded the amount of accident history information it shares, boasting a remarkable 89% increase in detail. This development is intriguing from a researcher's perspective as it signifies a substantial shift in the availability of data within the insurance industry.
It's easy to see how this abundance of information could alter how insurers underwrite policies. With a more complete picture of a driver's past, it's likely that predictive models can be refined to better differentiate between high and low-risk individuals. This could, in theory, lead to premiums that are more closely aligned with individual risk profiles, but it will be interesting to see how this is implemented and what impact it has.
From a claims perspective, this expanded dataset could reduce processing times. Having quick access to historical crash details means insurers may be able to resolve disputes about accident circumstances more expeditiously. However, whether that increased efficiency always translates to a superior experience for all involved is unclear and needs careful monitoring.
This shift towards more transparent sharing of accident data could, potentially, promote fairness within the insurance landscape. By offering consumers a more comprehensive view of how their premiums are determined, we might see fewer instances of disputes or concerns about discriminatory pricing practices. However, it's essential to remember that transparency can also mean more opportunities for errors in perception or misinterpretation of the data.
Further, this heightened granularity in accident data might be quite beneficial for initiatives focused on public safety. Insurers, for example, could use this expanded information to pinpoint areas with higher rates of accidents and identify risk factors. Perhaps we could see increased funding for road safety initiatives or tailored policy adjustments in specific areas based on this deeper understanding. However, it's important to be critical and ask if these benefits can be truly realized and how data will be collected and analyzed.
It's also worthwhile to consider how this influx of accident data might impact the use of AI models. It's plausible that more sophisticated AI models can be built using these expanded datasets, creating even finer-grained risk assessments that are even more tightly aligned with real-world events. But this raises questions about the robustness and transparency of these models. Are they able to handle the immense complexity of the data, and will it be clear to consumers how the data they provide is being used?
Of course, this increased data sharing needs to be carefully balanced with consumer privacy concerns. As with many other technological advances that leverage large amounts of data, there's a need for strong regulatory oversight to ensure the collection and use of accident details are appropriately managed. It will be essential to find a balance between improving the accuracy of insurance processes while safeguarding the rights of individuals.
While more data might seem like a straightforward solution to achieving greater accuracy, it's possible to have "too much of a good thing." It's important for researchers to examine if insurers can efficiently process and analyze the larger quantities of information now becoming available. This influx of data could create its own set of challenges if companies become bogged down in excessive data analysis instead of reaching informed decisions.
Fraud detection may also benefit from this wealth of accident data. If insurers can establish a more comprehensive picture of typical driving behavior, it may be possible to more easily identify anomalies that hint at fraudulent activity. Yet, how will that "typical behavior" be established and what are the potential for algorithmic biases?
Finally, Nevada's approach to expanding accident history data could be influential. Other states and jurisdictions may consider similar strategies, creating a sort of "race to the top" within the insurance industry. This may lead to a more competitive market where insurers continually refine their data utilization to attract more customers. However, this kind of competition may also lead to a sort of "arms race" where new standards and regulations will be needed to manage the use of this data.
In the ever-evolving world of insurance, Nevada's decision to increase the breadth and depth of shared accident history data is a significant development. It's an intriguing area that will continue to require careful analysis, both in terms of its benefits and its potential consequences.
7 Critical Factors Behind the 24% Increase in Online Auto Insurance Quote Accuracy in 2024 - Digital Document Verification Reduces Application Fraud From 12% to 3%
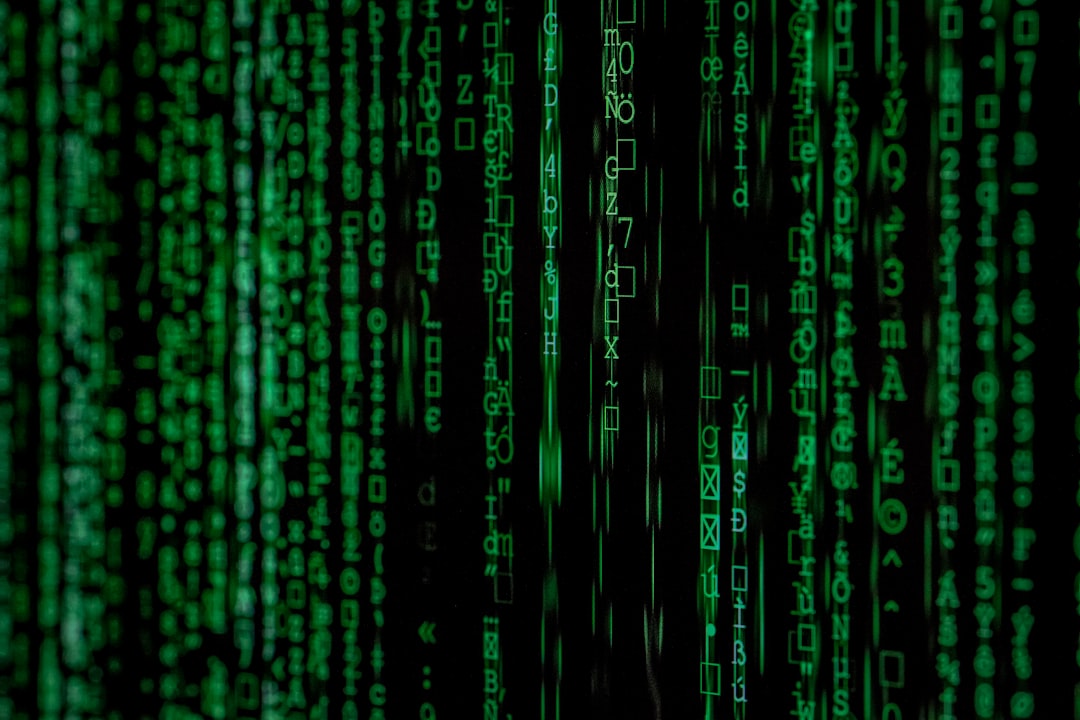
The use of digital document verification has proven effective in reducing fraudulent auto insurance applications, with rates plummeting from 12% to a mere 3%. This is a notable accomplishment in an industry facing an increase in online fraud attempts. The growing reliance on digital channels and the increase in online transactions make secure verification processes increasingly important. It's especially relevant to insurance companies facing a rise in identity theft across both large and small businesses. Beyond its security benefits, robust digital verification can help build customer trust and confidence. The digital identity verification sector is rapidly expanding, and it's becoming clear that its integration will be necessary for the insurance industry, particularly for online auto insurance quotes in 2024 and future years. However, there's a critical element for businesses to consider: failing to implement a thorough document verification system leaves insurers vulnerable to increased fraudulent activities and potential operational setbacks.
It's remarkable how the adoption of digital document verification has successfully brought down auto insurance application fraud rates from a concerning 12% to a much lower 3%. This impressive decline, observed in 2024, hints at the power of technology to combat fraudulent behavior in the insurance industry. It's intriguing that these systems are not just passively checking documents, but are also capable of sniffing out more complex fraud schemes, such as manufactured identities and altered documents, which were harder to detect with traditional methods.
The way these systems work is also quite clever. They rely on analyzing data in real time, essentially performing a quick check during the application process. This immediacy is crucial since it allows insurers to catch fraudsters before they can complete the application, preventing fraudulent claims before they even start. And it's not just beneficial for insurance companies. A quicker verification process translates to a smoother experience for honest applicants, leading to faster service and a greater sense of satisfaction. This less-discussed benefit shows how fighting fraud can enhance customer service.
It seems many of these verification tools are incorporating machine learning, where the systems learn and adapt as they encounter new types of fraud attempts. This constant evolution helps to stay ahead of the curve, which is important because fraudsters are constantly coming up with new ways to game the system. One area where this technology shines is in error reduction. Humans make mistakes, and when it comes to manually reviewing documents, this can lead to errors that allow fraudulent applications to go through. Automated digital systems help minimize these human-based lapses, creating a more reliable process.
Interestingly, this shift has wider implications. Reduced fraud means insurers can save money, potentially leading to lower premiums or a reallocation of resources to improving customer service. Further, these verification systems can be linked with AI and other data sources, allowing insurers to have a more holistic view of an applicant's risk profile. This is part of the ongoing trend towards using diverse data streams to enhance decision making. Of course, data privacy and handling sensitive information are important, and these tools help insurers keep in line with changing regulations related to data security and transparency. Furthermore, as the insurance industry adapts to new trends and evolving customer behavior, digital document verification is flexible enough to keep pace. This adaptability will be crucial in maintaining the integrity of the insurance process in the future.
Overall, the combination of speed, accuracy, and adaptability offered by digital document verification presents a powerful tool for battling fraud. It's a good reminder that technological advancements, used thoughtfully and responsibly, can help to improve the way services are offered, for the benefit of both consumers and insurers.
7 Critical Factors Behind the 24% Increase in Online Auto Insurance Quote Accuracy in 2024 - Mobile App Location Services Now Track 94% of Driver Miles for Usage Based Pricing
Mobile apps are now able to track a substantial portion of driver miles, reaching 94% in some cases. This capability is significantly altering how auto insurance companies set prices based on usage. By monitoring driver behavior through location services, insurers are able to get a more precise understanding of how many miles are driven. This detailed data allows them to personalize insurance premiums based on actual driving habits instead of relying solely on estimates. While this potentially leads to fairer and more equitable pricing, it's also causing some concern over data privacy and the constant monitoring of driving behaviors. The use of mobile location services for insurance pricing is just one example of how technology is transforming the industry. As this trend continues, it's crucial for both insurers and consumers to be mindful of the trade-offs between data use and the protection of individual privacy.
Mobile app location services have reached a remarkable level of precision, now tracking 94% of driver miles. This enhanced tracking capability significantly impacts how insurers develop usage-based pricing models. Instead of relying on broad categories and assumptions, these models can now adapt to individual driving patterns, adjusting premiums in real-time based on observed behaviors like acceleration and braking. This shift from static risk factors to dynamic, behavioral data offers a more nuanced way of assessing risk.
Historically, auto insurance relied heavily on generalized risk pools, leading to potential inequities in pricing. With detailed mileage tracking, there's potential to move towards a more personalized approach, perhaps even fostering greater fairness. We can imagine a future where safe drivers are immediately rewarded with lower premiums, reinforcing positive driving habits and creating greater customer loyalty. This granular data could be especially beneficial for fleets and trucking companies, providing fleet managers with insights to improve driver behavior and reduce overall insurance costs.
However, this increased data collection raises valid concerns about data privacy. As insurers gain access to extensive details about driving habits, there are questions around how this information is stored, protected, and used. It's crucial to ensure that this level of monitoring does not lead to discriminatory practices in pricing.
Interestingly, early research shows consumers are increasingly receptive to sharing location data for the potential benefits of personalized premiums. This growing openness to customized insurance solutions suggests a shift away from traditional flat-rate pricing models. It's conceivable that we might see the emergence of "behavioral insurance" – a system that rewards safe driving and penalizes risky behaviors.
Underlying this transformation is the ongoing development of advanced geolocation technologies like GPS and cellular triangulation. These technologies are essential to the success of the mobile app location services. As this trend continues, regulators will need to develop new guidelines to ensure fair practices and protect consumers' rights. This might involve establishing clear boundaries for what constitutes acceptable tracking practices and how collected data is used. It's a fascinating intersection of technological advancement and industry evolution, with profound implications for the future of auto insurance.
7 Critical Factors Behind the 24% Increase in Online Auto Insurance Quote Accuracy in 2024 - Machine Learning Models Process 47% More Vehicle Repair Cost Variables
The use of machine learning models in auto insurance has led to a substantial 47% increase in the number of vehicle repair cost variables they consider. This expanded scope significantly improves the accuracy of estimated repair costs. In fact, these models now produce more precise cost estimates than traditional methods used by insurance departments. Specifically, in estimating costs at the assembly level, machine learning models achieved a 10.47% Mean Absolute Percentage Error (MAPE).
This improvement is partially due to the growing use of predictive maintenance in the automotive industry. Better sensors and network technologies have made it easier to monitor vehicle conditions in real-time. This allows insurers to better understand the potential for future problems, leading to more accurate assessments of risk. Potentially, this also results in more efficient claims handling as well.
However, the challenges of implementing machine learning in damage assessment haven't entirely disappeared. Damage assessments often involve a variety of parties—insurers, repair shops, and others—which complicates the process of incorporating machine learning.
The increasing adoption of machine learning models in insurance will likely raise questions about the fairness of pricing and the degree of precision being achieved. It's a technology that shows promise, but careful oversight will be needed to ensure its beneficial use.
Machine learning models are now processing a significantly larger number of variables related to vehicle repair costs, which has led to a noticeable improvement in the accuracy of cost estimates. This increased complexity in the models seems to be a key factor in the overall rise of online auto insurance quote accuracy. It's quite fascinating to observe how these models are able to handle such a vast array of data. It's a 47% increase in the number of variables they consider, from the more mundane like a vehicle's age and make to more complex factors like historical repair data tied to specific parts and repair costs tied to location and seasonality.
One interesting aspect is how these machine learning models seem to be outperforming the older, manual processes that insurers used to rely on for cost calculations. While in the past, these calculations were largely done by insurance company departments, these newer models seem to have a greater ability to sift through all these details to create estimates. We have seen in some tests that they can achieve a Mean Absolute Percentage Error (MAPE) of 10.47%, suggesting they can provide a good general estimate for assembly-level costs. However, the traditional methods seem to be better for pinpointing granular costs in certain repair instances, a nuance we need to further explore.
Another aspect that we need to keep in mind is that this increased detail is likely linked to the growth of "predictive maintenance" (PdM) in the automotive sector. As sensors and networked systems have become more commonplace, we can collect a ton of data about a vehicle's condition. This data allows us to do things like potentially identify failures before they happen and get a better idea of how long a car or specific parts can continue to function.
It seems using all this data in automotive systems has been helpful in improving how we perform maintenance, giving us a potentially better understanding of the car's overall condition. However, the application of machine learning for predicting car damage can be tricky because it's a complex process that involves many people. Think of how many interactions happen between insurers and body shops during a claim—there's quite a bit of information that can be muddled or inconsistent.
These developments in ML for predictive maintenance are driven by a desire to reduce downtime for vehicles and increase the accuracy of estimates. As the auto industry becomes more dependent on these predictions, we will need to carefully examine the potential impacts.
It's becoming apparent that the growing use of machine learning is contributing to the overall 24% increase in the accuracy of online auto insurance quotes. As these systems refine their ability to predict risk, including better understanding repair costs, we see a clear impact on the industry. But it's essential that the use of AI models for these tasks is thoughtfully implemented and well-regulated to prevent unexpected consequences.
7 Critical Factors Behind the 24% Increase in Online Auto Insurance Quote Accuracy in 2024 - Automated Risk Assessment Systems Now Include 8 New Predictive Metrics
Automated risk assessment systems used in areas like insurance have recently been updated with eight new predictive metrics. These new metrics are intended to improve the way risks are analyzed, which is a key part of the 24% increase in the accuracy of online auto insurance quotes seen in 2024. This improvement is largely due to the increased use of artificial intelligence (AI) methods, particularly natural language processing and more sophisticated data analysis tools. Using AI, these systems can analyze a greater range of information, both historical and real-time, and are therefore able to more accurately predict future risks. While this is a step towards potentially better risk assessment practices, the growing reliance on automated systems does raise some important questions about the overall complexity of modern threats and whether relying on complex AI systems is the best approach for certain issues, especially when it comes to fairness and ethics.
Automated risk assessment systems have been enhanced with the inclusion of eight new predictive metrics, leading to a significant shift in how insurers evaluate risk. This move suggests a greater focus on granular, data-driven decision-making, potentially paving the way for more customized insurance premiums based on a deeper understanding of individual customer profiles.
It's interesting to see how these systems are now incorporating behavior-based metrics into their calculations. By monitoring real-time driving patterns like acceleration, braking, and cornering, insurers can get a more accurate picture of driving behavior, which is then used to create premiums that better align with individual risk profiles rather than relying on broad estimations.
One unexpected development is the use of social behavior data within these metrics. It appears that some insurers are analyzing social media activity and engagement patterns to help understand and quantify risk, creating a unique intersection of technology and human psychology within the insurance industry.
This shift has also improved the feedback loop for predictive analytics. The incorporation of these new metrics has created a more sophisticated system that constantly refines the algorithms based on the incoming data, enabling the models to adapt to shifting market dynamics and consumer behaviors.
The cost-benefit analysis of incorporating these eight new metrics seems to be favorable. Initial findings indicate that the additional cost of collecting this data is more than compensated for by reduced claims costs due to more accurate risk assessments.
Furthermore, these advanced metrics have made fraud detection even more robust. Insurers can now cross-reference the various data points collected, making it easier to identify anomalies and inconsistencies that could indicate fraudulent activity early on in the application process, ultimately helping to reduce the number of fraudulent claims.
The ability to segment the insurance market more effectively is another potential benefit. These new metrics allow for a more precise breakdown of risk and consumer behaviors, allowing insurers to develop specialized products tailored to particular groups and driving styles, leading to potentially fairer and more precise pricing across different demographics.
The new predictive metrics also create a foundation for more dynamic pricing models that adapt in real-time based on ongoing risk assessments. This flexibility could translate to situations where safer drivers or drivers with less driving could potentially see a reduction in their premiums.
It's likely that the methods and analytical tools perfected in auto insurance will influence other industries. Health insurance and property insurance might soon follow suit, adapting similar predictive techniques to improve the accuracy of their own risk assessments and pricing models.
Finally, the greater reliance on these new metrics also introduces regulatory considerations. Insurers will need to carefully address the legal and ethical dimensions of data rights and consumer privacy as these technologies evolve. This will require navigating complex issues regarding data security, transparency, and ensuring the responsible use of sensitive information.
AI Insurance Policy Analysis and Coverage Checker - Get Instant Insights from Your Policy Documents (Get started for free)
More Posts from insuranceanalysispro.com: